CLHHN: Category-aware Lossless Heterogeneous Hypergraph Neural Network for Session-based Recommendation
ACM TRANSACTIONS ON THE WEB(2024)
摘要
In recent years, session-based recommendation (SBR), which seeks to predict the target user's next click based on anonymous interaction sequences, has drawn increasing interest for its practicality. The key to completing the SBR task is modeling user intent accurately. Due to the popularity of graph neural networks (GNNs), most state-of-the-art (SOTA) SBR approaches attempt to model user intent from the transitions among items in a session with GNNs. Despite their accomplishments, there are still two limitations. First, most existing SBR approaches utilize limited information from short user-item interaction sequences and suffer from the data sparsity problem of session data. Second, most GNN-based SBR approaches describe pairwise relations between items while neglecting complex and high-order data relations. Although some recent studies based on hypergraph neural networks have been proposed to model complex and high-order relations, they usually output unsatisfactory results due to insufficient relation modeling and information loss. To this end, we propose a category-aware lossless heterogeneous hypergraph neural network (CLHHN) in this article to recommend possible items to the target users by leveraging the category of items. More specifically, we convert each category-aware session sequence with repeated user clicks into a lossless heterogeneous hypergraph consisting of item and category nodes as well as three types of hyperedges, each of which can capture specific relations to reflect various user intents. Then, we design an attention-based lossless hypergraph convolutional network to generate sessionwise and multi-granularity intent-aware item representations. Experiments on three real-world datasets indicate that CLHHN can outperform the SOTA models in making a better tradeoff between prediction performance and training efficiency. An ablation study also demonstrates the necessity of CLHHN's key components.
更多查看译文
AI 理解论文
溯源树
样例
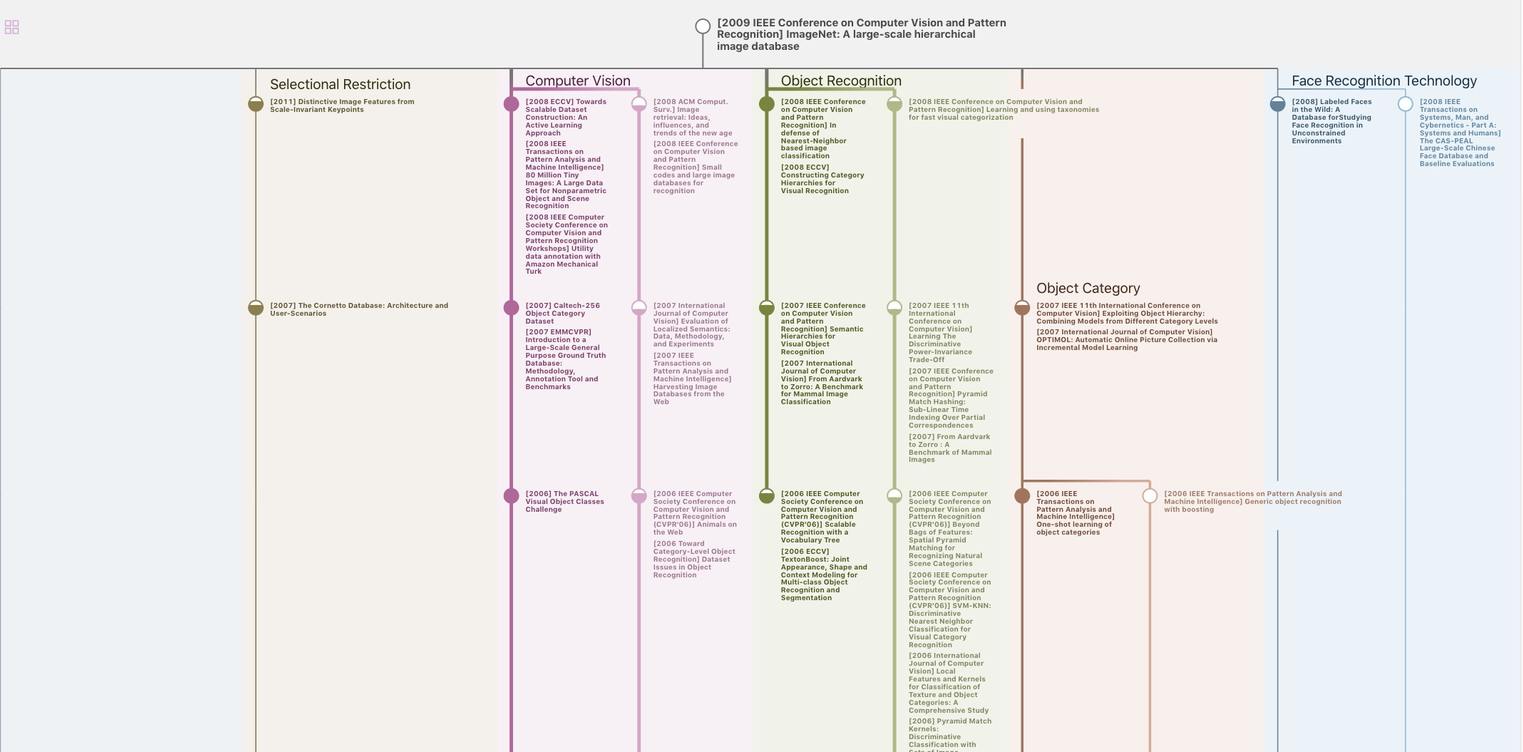
生成溯源树,研究论文发展脉络
Chat Paper
正在生成论文摘要