A Machine Learning-driven EDAC Method for Space-Application Memory
2023 IEEE International Symposium on Defect and Fault Tolerance in VLSI and Nanotechnology Systems (DFT)(2023)
摘要
Space-based applications are susceptible to the effects of energetic particles, resulting in single-event effects (SEEs). This vulnerability becomes critical during a solar particle event (SPE), when space radiation intensity can drastically surge, altering fault probabilities in space applications by several orders of magnitude within hours or days. Traditional fault mitigation methods like error detection and correction (EDAC) may become overwhelmed during SPEs, resulting in an excessive fault rate. Addressing this issue, we propose a dynamic parity per byte and duplication (PBD) method, augmented with machine learning, for effective EDAC based on fault prediction. This approach leverages a pre-trained machine learning model and real-time fault statistics from the PBD method to accurately predict future memory faults. Guided by the prediction, an adaptive strategy adjusts the wash frequency of the PBD method during periods of heightened fault probability, thereby mitigating fault accumulation. The proposed methodology has undergone mathematical verification and empirical validation using actual space environment data from Solar Cycle 24. The results demonstrate the effectiveness of our approach in mitigating fault accumulation during SPEs and enhancing the robustness of the EDAC algorithm under extreme radiation conditions.
更多查看译文
关键词
Error Detection and Correction,Memory Reliability,Machine Learning,Space Environment
AI 理解论文
溯源树
样例
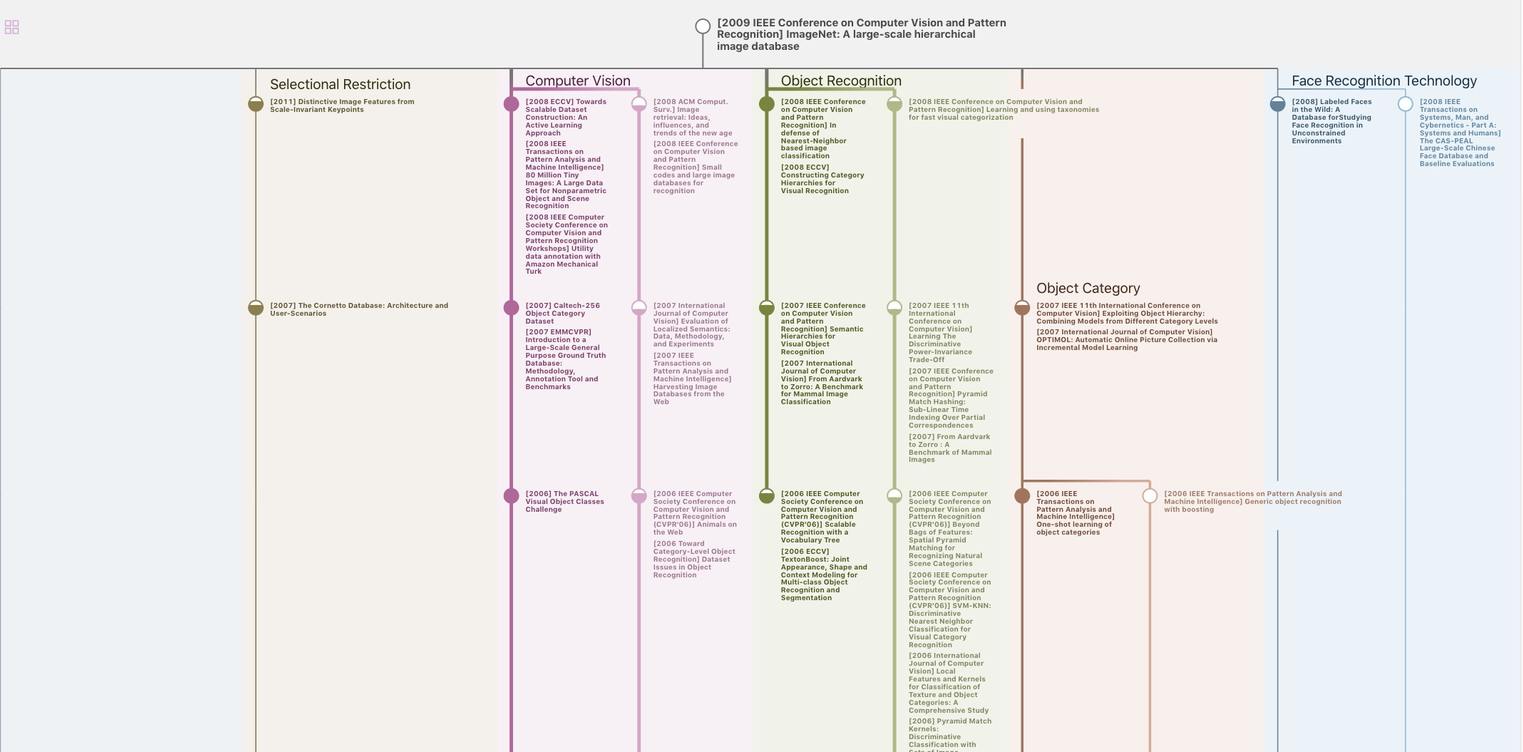
生成溯源树,研究论文发展脉络
Chat Paper
正在生成论文摘要