An Estimation Method of Defect Types Using Artificial Neural Networks and Fault Detection Information
2023 IEEE International Symposium on Defect and Fault Tolerance in VLSI and Nanotechnology Systems (DFT)(2023)
摘要
Fault diagnosis methods for specific fault models might cause misprediction or sometimes cannot provide any solution. Therefore, a fault diagnosis method based on a single universal logical fault model has been proposed, which utilizes multi-cycle capture test sets for scan-designed circuits. Although it has been reported that this method can accurately estimate locations of faults, this method still has problems on the number of suspected faulty lines as well as incapability of specifying defect types. In this paper, targeting fault locations diagnosed under the single universal logical fault model, we propose an estimation method of defect types using artificial neural networks. In the proposed method, the features corresponding to the major logical fault models (i.e., stuck-at fault, dominant bridging fault, open fault, and gate-exhaustive fault) are calculated from the fault diagnosis results for a single universal logical fault model and the logic simulation results. Defect types are estimated from the calculated features by using an artificial neural network. Experimental results for benchmark circuits showed that the proposed method achieved the accuracy more than 95%.
更多查看译文
关键词
fault diagnosis,universal logical fault model,multi-cycle capture testing,artificial neural networks,estimation of defect types
AI 理解论文
溯源树
样例
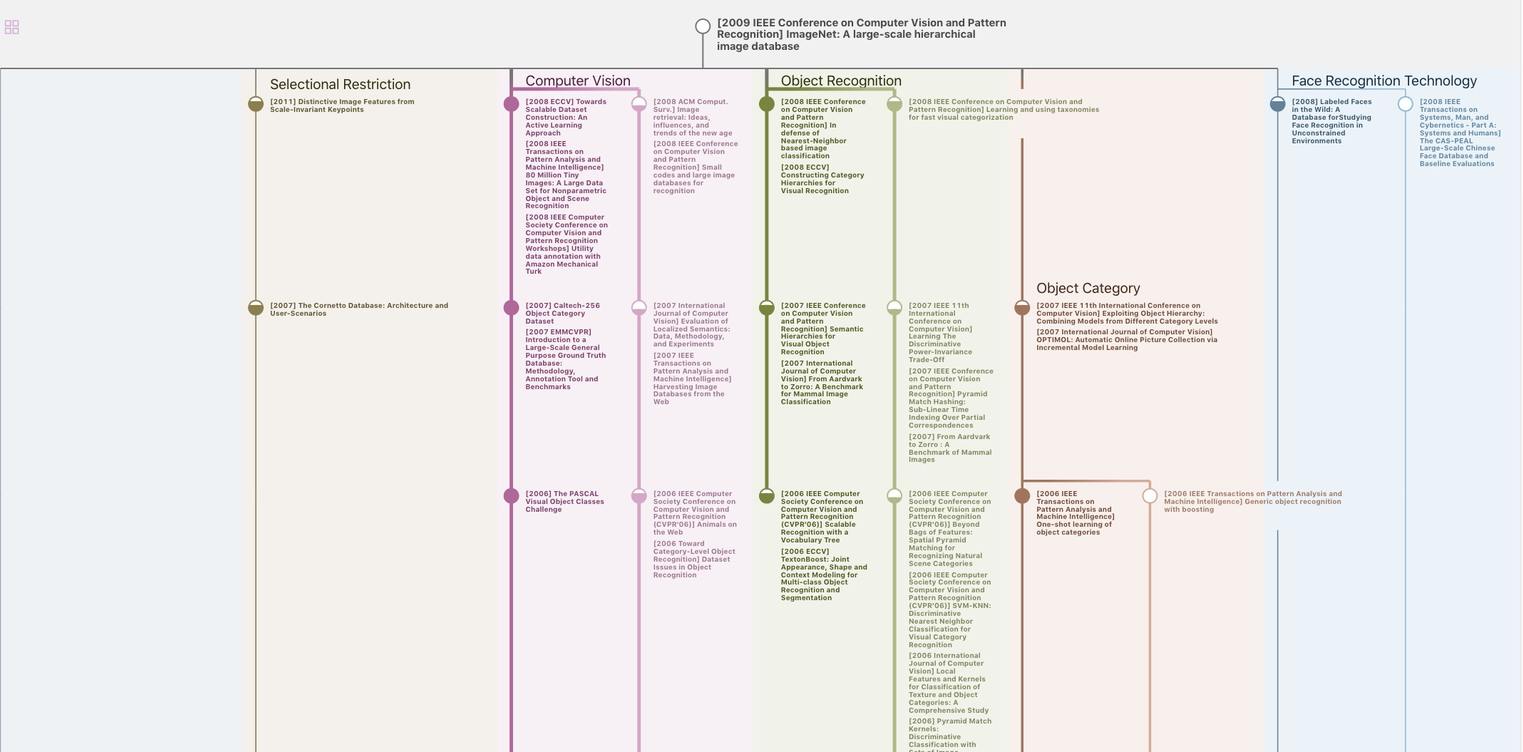
生成溯源树,研究论文发展脉络
Chat Paper
正在生成论文摘要