Discriminative Stacked Autoencoder: Feature-Integration Boosting for Bearing Fault Diagnosis
IEEE SENSORS JOURNAL(2023)
摘要
Traditional deep learning-based fault diagnosis scenarios always rely on a certain type of deep neural networks (DNNs) to extract its inherent features, which can overcome the drawbacks of shallow model methods. However, the invariant features extracted have some inevitable limitations, which lead to the performance degeneration and lower generalization ability of these scenarios. To address this problem, we propose a feature-integration-boosting-based discriminative stacked autoencoder (D-SAE) network for bearing fault diagnosis. Specifically, multiple D-SAEs are used as weak classifiers to extract features from the raw signal. To relax the inevitable limitations of a single DNN, D-SAE networks are employed to build the features by integrating boosting into the feature level adaptively, which can combine the weighted features extracted by the D-SAEs according to the classification accuracy. In addition, the classification information is stored in the hidden layer of the networks, preventing any potential loss of classification information that may occur as the D-SAE becomes more complex. This ensures that the performance of the classifiers does not deteriorate. With extensive experiments on the Case Western Reserve University (CWRU) dataset and a real petrochemical industrial unit dataset, the results indicate that significant improvements over several existing other previous methods were achieved.
更多查看译文
关键词
Adaptive boosting (AdaBoost),discriminative stacked autoencoder (D-SAE),fault diagnosis,rotating machinery
AI 理解论文
溯源树
样例
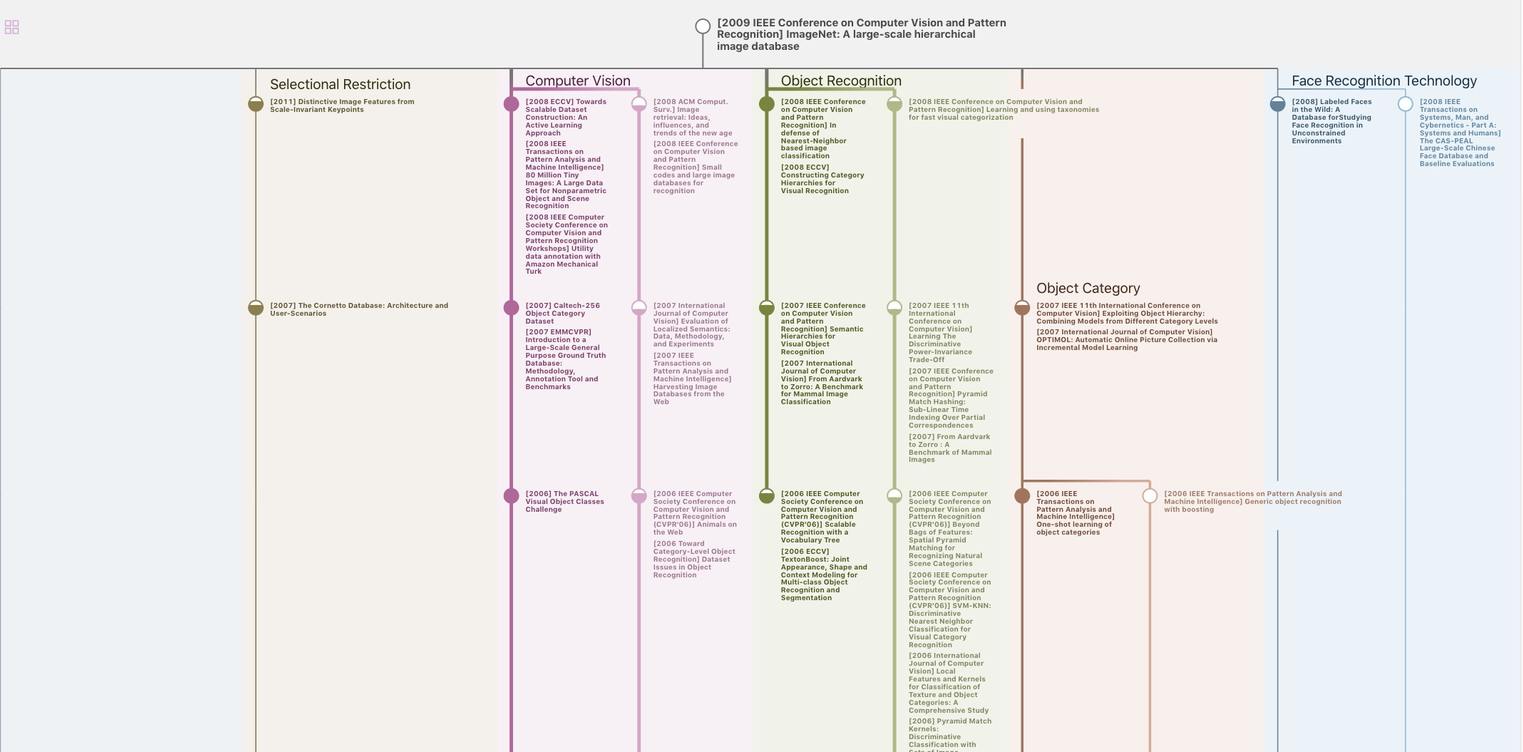
生成溯源树,研究论文发展脉络
Chat Paper
正在生成论文摘要