Deep neural network with self-attention based automated determination system for treatment zone and peripheral steepened zone in Orthokeratology for adolescent myopia
CONTACT LENS & ANTERIOR EYE(2024)
摘要
Purpose: The aim of this study is to develop an automatic model based on deep learning techniques for determining the Treatment Zone (TZ) and Peripheral Steepened Zone (PSZ) following Orthokeratology (OK) treatment. Methods: A total of 1346 corneal topography maps were included in the study. A deep neural network based on the Segformer architecture was constructed to automatically detect TZ and PSZ. The model was optimized and trained multiple times, and the areas of TZ, PSZ, and TZ decentration were calculated based on the segmentation results. Results: The mean Intersection over Union (mIoU) of the overall segmentation results of the model reached over 97% after multiple training with different optimization methods, and the IoU for the TZ and PSZ segmentation tasks were 98.08% and 94.54% in test set, respectively. Moreover, the model demonstrated high consistency with the expert annotation for the TZ segmentation, while a significant difference was found in the PSZ segmentation and expert annotation due to several interference factors. Conclusion: This study presents an efficient and repeatable system for clinical research, based on a deep neural network that accurately determines TZ and PSZ after OK treatment using the Segformer architecture. However, further deployment validation may be necessary.
更多查看译文
关键词
Orthokeratology,Artificial Intelligence,Treatment Zone,Peripheral Steepened Zone,Fitting estimation
AI 理解论文
溯源树
样例
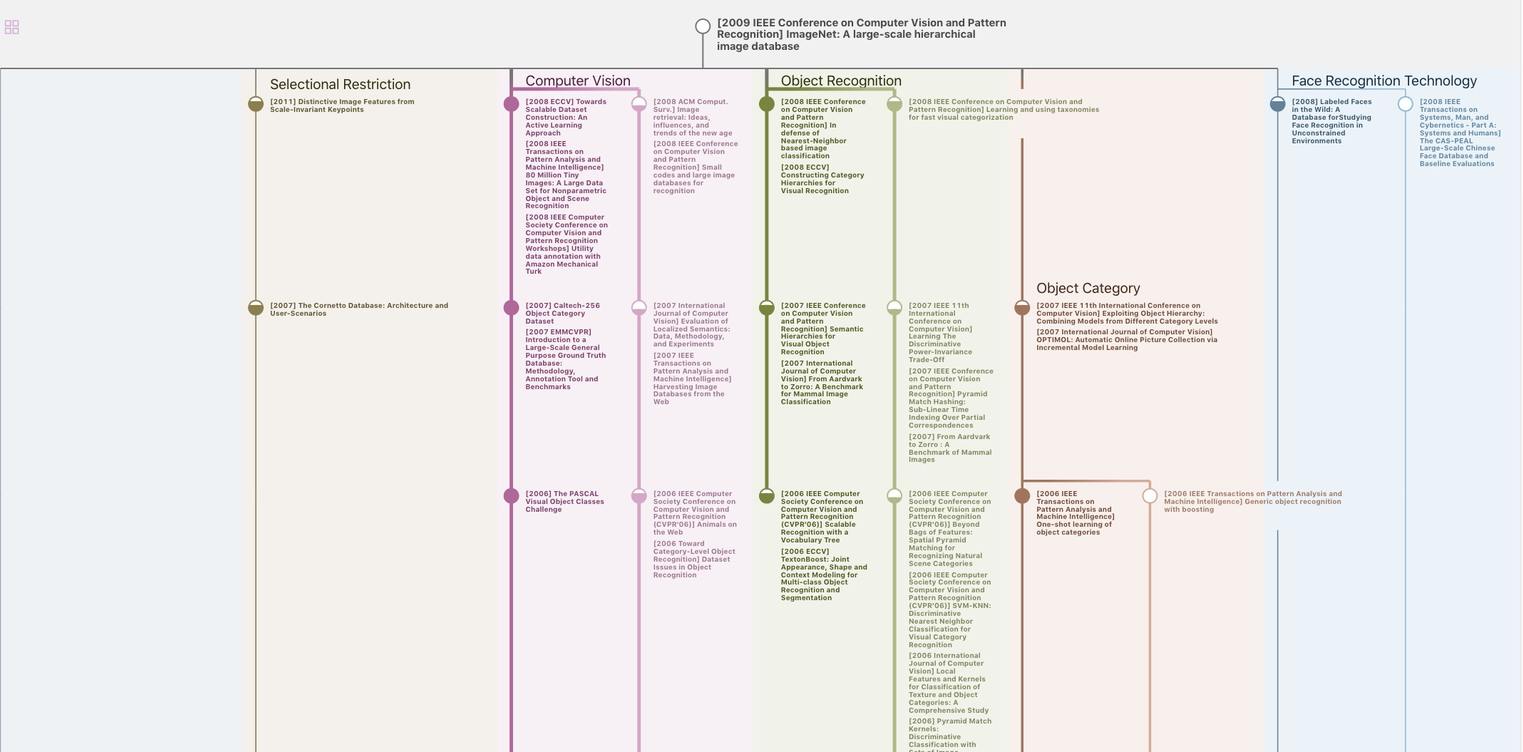
生成溯源树,研究论文发展脉络
Chat Paper
正在生成论文摘要