A Siamese-Transport Domain Adaptation Framework for 3D MRI Classification of Gliomas and Alzheimer's Diseases
IEEE JOURNAL OF BIOMEDICAL AND HEALTH INFORMATICS(2024)
摘要
Accurate and fully automated brain structure examination and prediction from 3D volumetric magnetic resonance imaging (MRI) is a necessary step in medical imaging analysis, which can assist greatly in clinical diagnosis. Traditional deep learning models suffer from severe performance degradation when applied to clinically acquired unlabeled data. The performance degradation is mainly caused by domain discrepancy such as different device types and parameter settings for data acquisition. However, existing approaches focus on the reduction of domain discrepancies but ignore the entanglement of semantic features and domain information. In this article, we explore the feature invariance of categories and domains in different projection spaces and propose a Siamese-Transport Domain Adaptation (STDA) method using a joint optimal transport theory and contrastive learning for automatic 3D MRI classification and glioma multi-grade prediction. Specifically, the learning framework updates the distribution of features across domains and categories by Siamese transport network training with an Optimal Cost Transfer Strategy (OCTS) and a Mutual Invariant Constraint (MIC) in two projective spaces to find multiple invariants in potential heterogeneity. We design three sets of transfer task scenarios with different source and target domains, and demonstrate that STDA yields substantially higher generalization performance than other state-of-the-art unsupervised domain adaptation (UDA) methods. The method is applicable on 3D MRI data from glioma to Alzheimer's disease and has promising applications in the future clinical diagnosis and treatment of brain diseases.
更多查看译文
关键词
Glioma grading,unsupervised domain adaptation,feature representation,probability distribution,invariant constraint
AI 理解论文
溯源树
样例
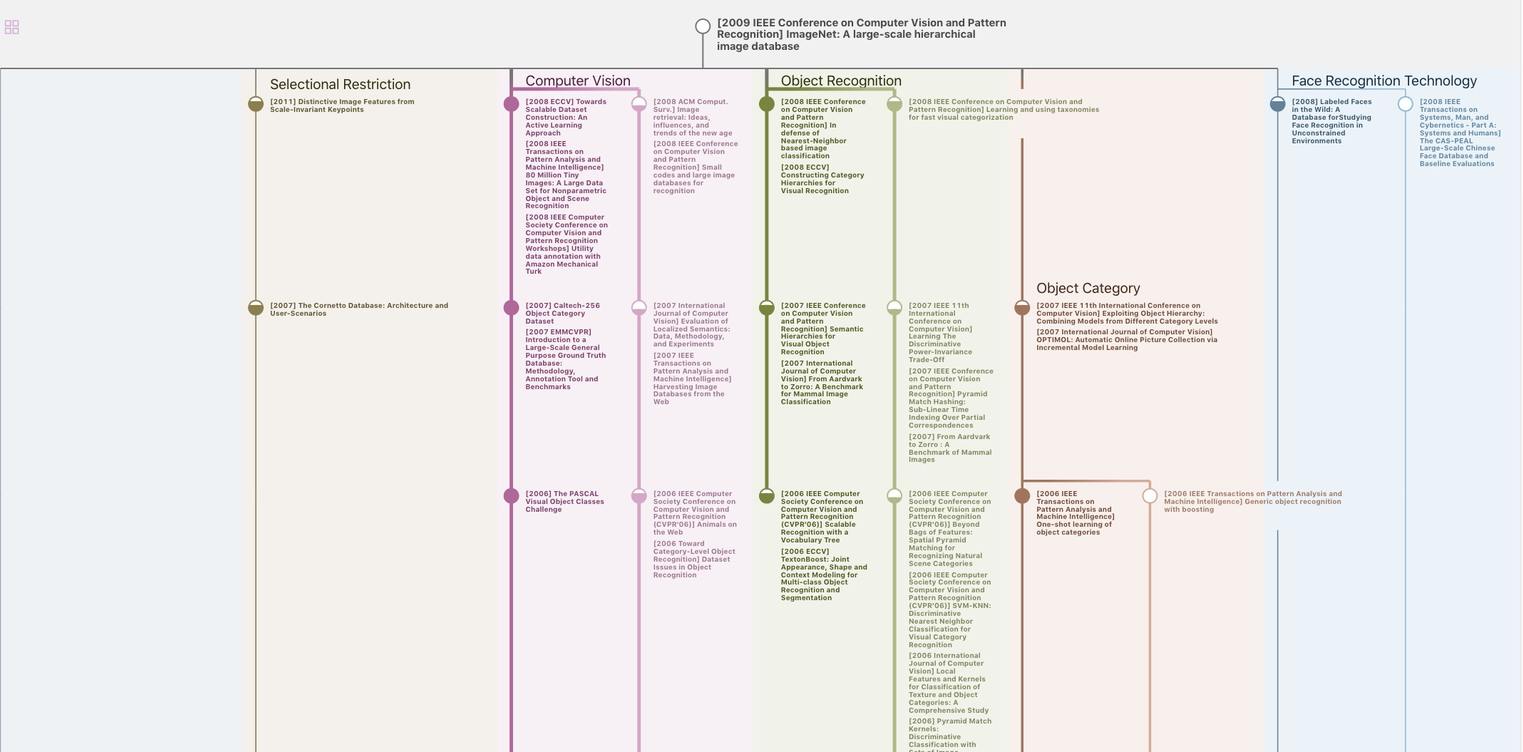
生成溯源树,研究论文发展脉络
Chat Paper
正在生成论文摘要