PriOmics: Integration of High-Throughput Proteomic Data with Complementary Omics Layers Using Mixed Graphical Modeling with Group Priors
biorxiv(2023)
Key words
Proteomics,Genomic Data Integration,Protein-Protein Interaction Networks,Phosphoproteomics,Mass Spectrometry
AI Read Science
Must-Reading Tree
Example
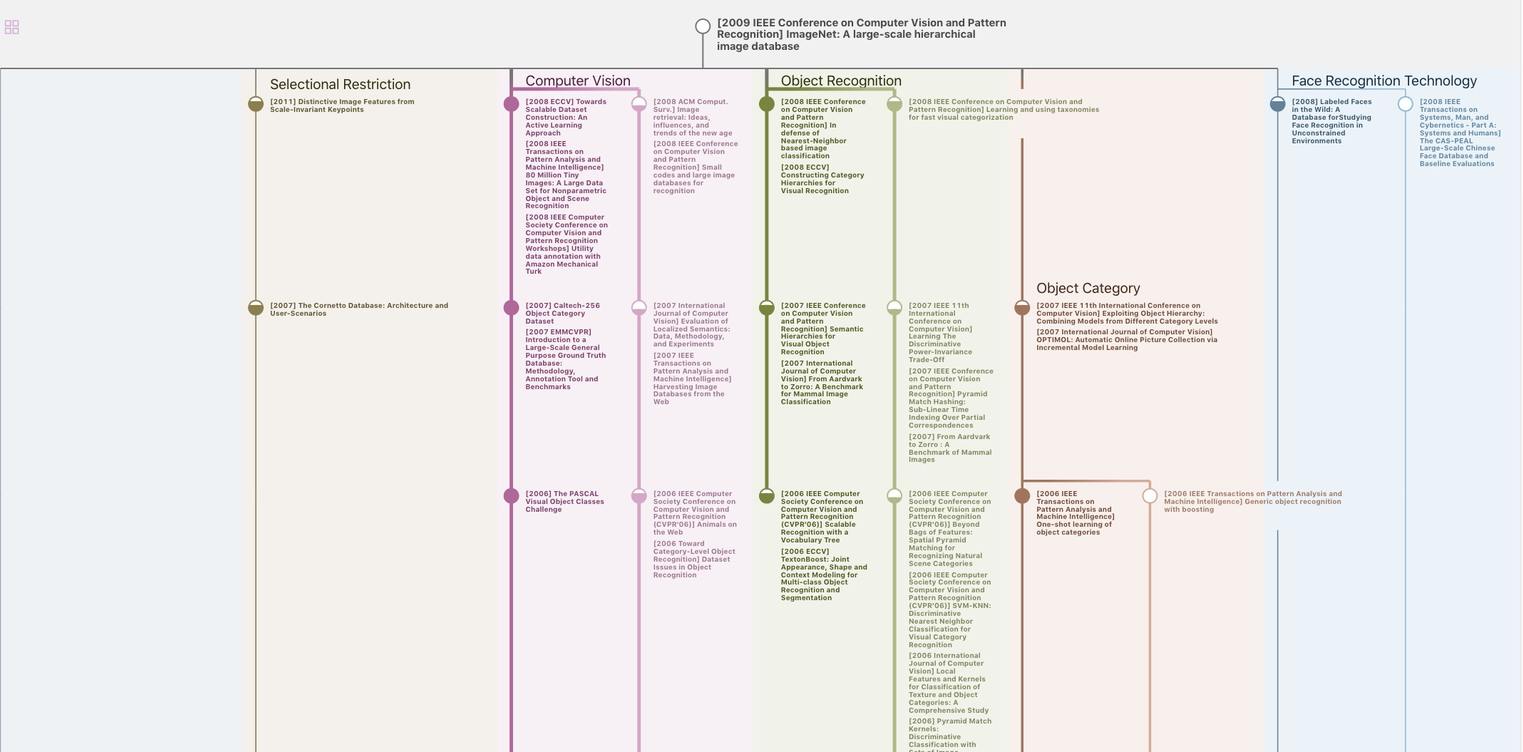
Generate MRT to find the research sequence of this paper
Chat Paper
Summary is being generated by the instructions you defined