Learning Bayes-Optimal Channel Estimation for Holographic MIMO in Unknown EM Environments
arXiv (Cornell University)(2023)
Abstract
Holographic MIMO (HMIMO) has recently been recognized as a promising enabler
for future 6G systems through the use of an ultra-massive number of antennas in
a compact space to exploit the propagation characteristics of the
electromagnetic (EM) channel. Nevertheless, the promised gain of HMIMO could
not be fully unleashed without an efficient means to estimate the
high-dimensional channel. Bayes-optimal estimators typically necessitate either
a large volume of supervised training samples or a priori knowledge of the true
channel distribution, which could hardly be available in practice due to the
enormous system scale and the complicated EM environments. It is thus important
to design a Bayes-optimal estimator for the HMIMO channels in arbitrary and
unknown EM environments, free of any supervision or priors. This work proposes
a self-supervised minimum mean-square-error (MMSE) channel estimation algorithm
based on powerful machine learning tools, i.e., score matching and principal
component analysis. The training stage requires only the pilot signals, without
knowing the spatial correlation, the ground-truth channels, or the received
signal-to-noise-ratio. Simulation results will show that, even being totally
self-supervised, the proposed algorithm can still approach the performance of
the oracle MMSE method with an extremely low complexity, making it a
competitive candidate in practice.
MoreTranslated text
Key words
6G,holographic MIMO,channel estimation,score matching,self-supervised learning,MMSE estimation
AI Read Science
Must-Reading Tree
Example
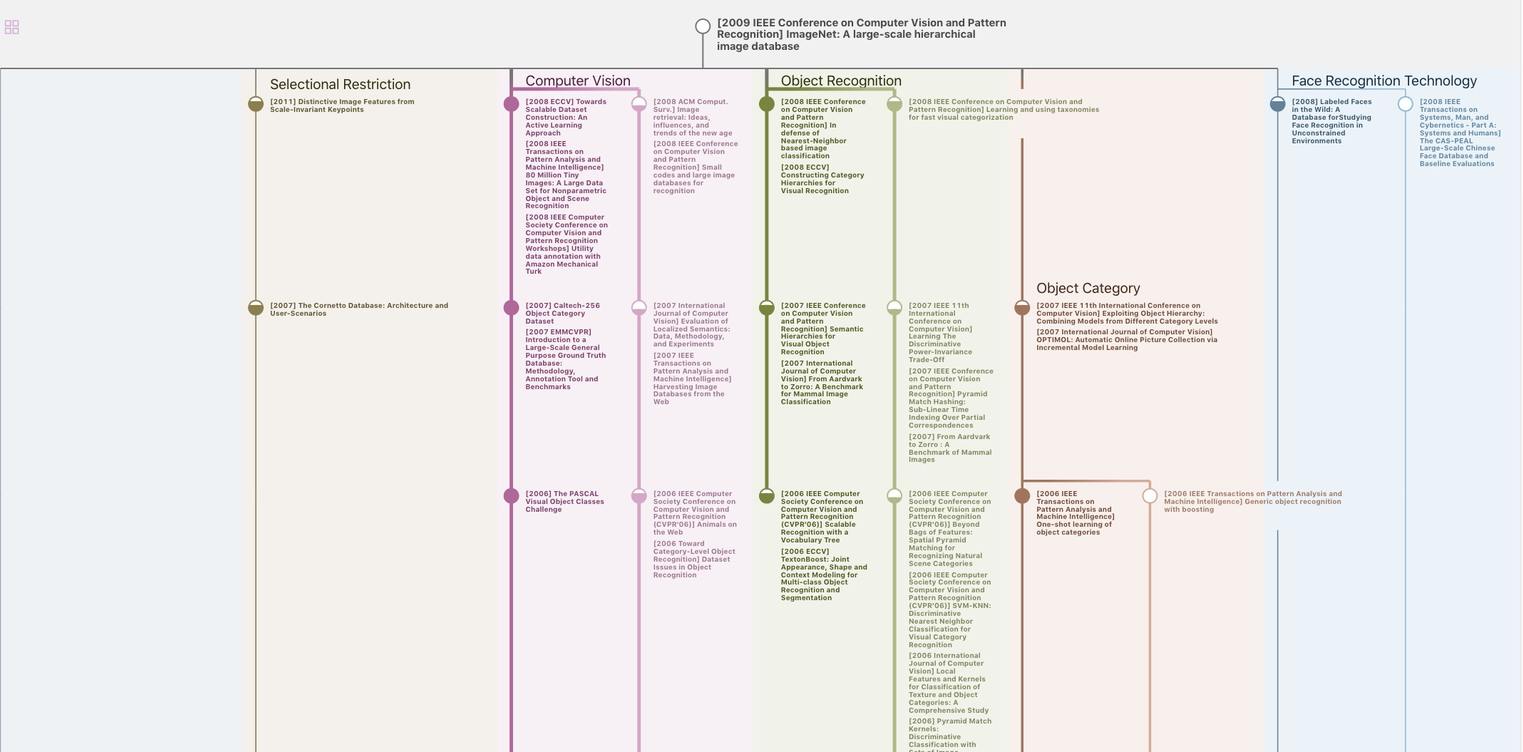
Generate MRT to find the research sequence of this paper
Chat Paper
Summary is being generated by the instructions you defined