Asymmetrical Attention Networks Fused Autoencoder for Debiased Recommendation
ACM TRANSACTIONS ON INTELLIGENT SYSTEMS AND TECHNOLOGY(2023)
Abstract
Popularity bias is a massive challenge for autoencoder-based models, which decreases the level of personalization and hurts the fairness of recommendations. User reviews reflect their preferences and help mitigate bias or unfairness in the recommendation. However, most existing works typically incorporate user (item) reviews into a long document and then use the same module to process the document in parallel. Actually, the set of user reviews is completely different from the set of item reviews. User reviews are heterogeneous in that they reflect a variety of items purchased by users, while item reviews are only related to the item itself and are thus typically homogeneous. In this article, a novel asymmetric attention network fused with autoencoders is proposed, which jointly learns representations from the user and item reviews and implicit feedback to perform recommendations. Specifically, we design an asymmetric attentive module to capture rich representations from user and item reviews, respectively, which solves data sparsity and explainable problems. Furthermore, to further address popularity bias, we apply a noise-contrastive estimation objective to learn high-quality "de-popularity" embedding via the decoder structure. A series of extensive experiments are conducted on four benchmark datasets to show that leveraging user review information can eliminate popularity bias and improve performance compared to various state-of-the-art recommendation techniques.
MoreTranslated text
Key words
Popularity bias,review-aware Recommendation,asymmetrical hierarchical network,autoencoder
AI Read Science
Must-Reading Tree
Example
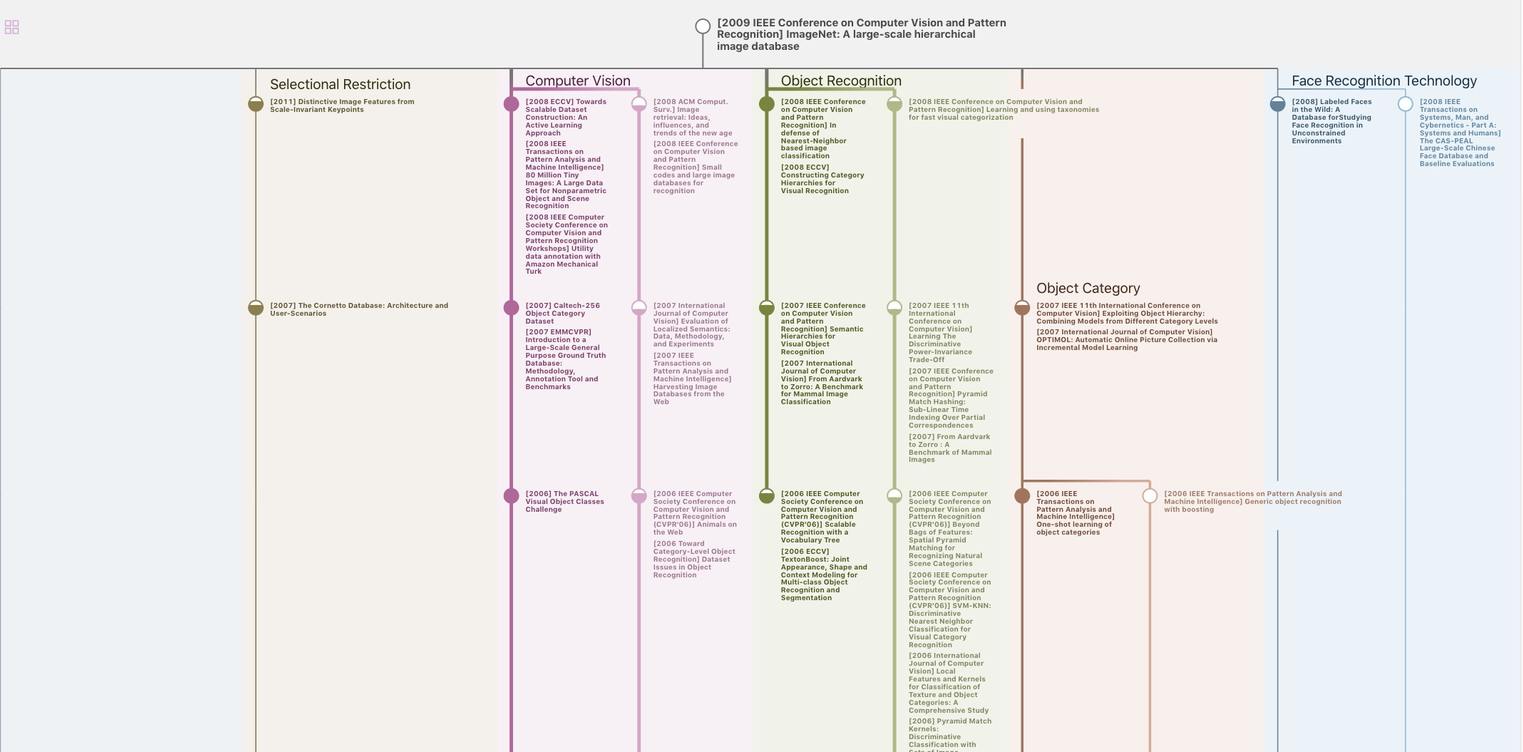
Generate MRT to find the research sequence of this paper
Chat Paper
Summary is being generated by the instructions you defined