Quality assessment of anterior segment OCT images: Development and validation of quality criteria.
Photodiagnosis and photodynamic therapy(2023)
Abstract
BACKGROUND:The utility of medical imaging is dependant on image quality. We aimed to develop and validate quality criteria for ocular anterior segment optical coherence tomography (AS-OCT) images.
METHODS:We undertook a cross-sectional study using AS-OCT images from patients aged 6-16. A novel three-level grading system (good, limited or poor) was developed based on the presence of image artefact (categorised as lid, eyelash, cropping, glare, or movement artefact). Three independent experts graded 2825 images, with agreement assessed using confusion matrices and intraclass correlation coefficients (ICC) for each parameter.
RESULTS:There was very good inter-grader IQA agreement assessing image quality with ICC 0.85 (95 %CI: 0.84-0.87). The most commonly occurring artefact was eyelash artefact (1008/2825 images, 36 %). Graders labelled 621/2825 (22 %) images as good and 384 (14 %) as poor. There was complete agreement at either end of the confusion matrix with no 'good' images labelled as 'poor' by other graders, and vice versa. Similarly, there was very good agreement when assessing presence of lash (0.96,0.94-0.98), movement (0.97,0.96-0.99), glare (0.82,0.80-0.84) and cropping (0.90,0.88-0.92).
CONCLUSIONS:The novel image quality assessment criteria (IQAC) described here have good interobserver agreement overall, and excellent agreement on the differentiation between 'good' and 'poor' quality images. The large proportion of images graded as 'limited' suggests the need for refine this classification, using the specific IQAC features, for which we also report high interobserver agreement. These findings support the future potential for wider clinical and community care implementation of AS-OCT for the diagnosis and monitoring of ocular disease.
MoreTranslated text
AI Read Science
Must-Reading Tree
Example
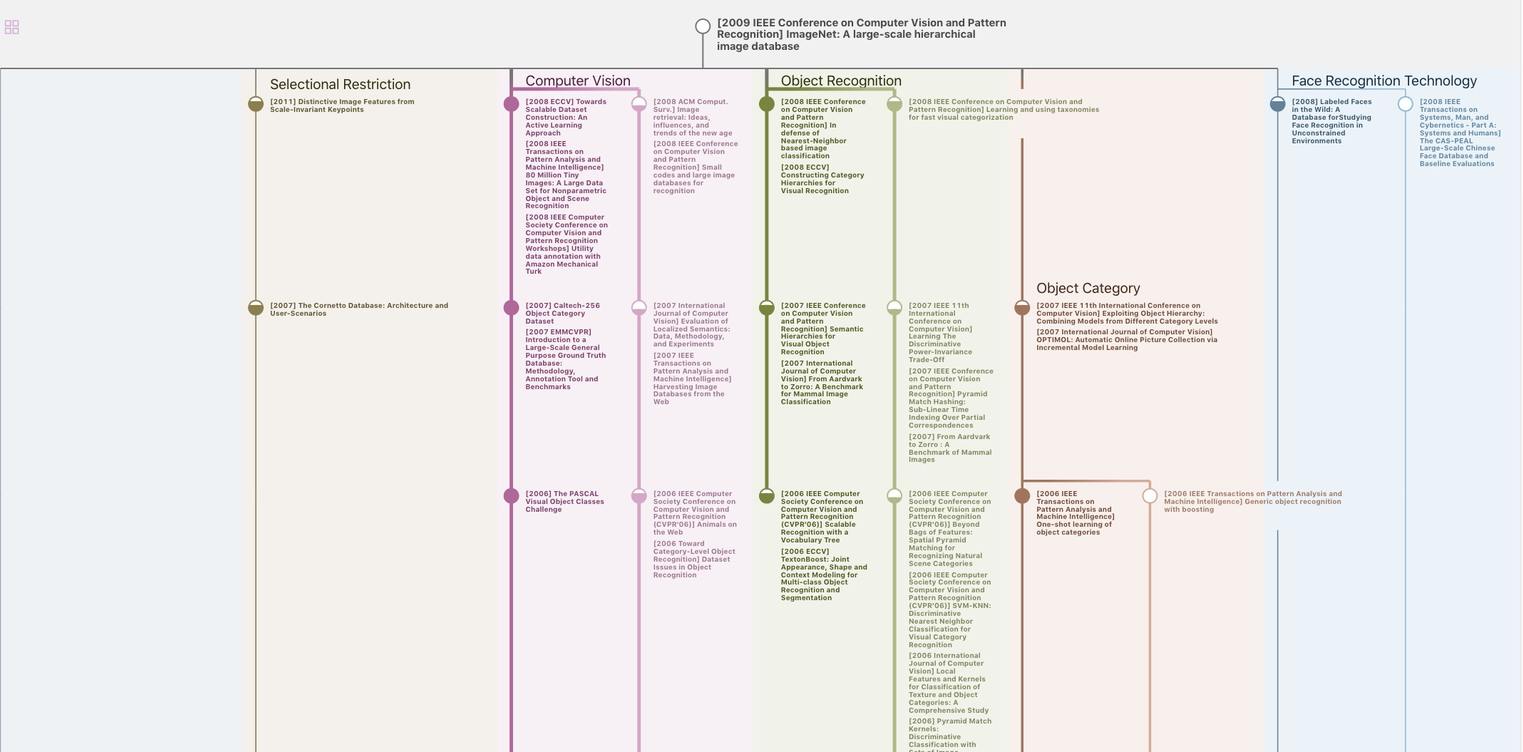
Generate MRT to find the research sequence of this paper
Chat Paper
Summary is being generated by the instructions you defined