Spatiotemporal heterogeneity of ecosystem service interactions and their drivers at different spatial scales in the Yellow River Basin
SCIENCE OF THE TOTAL ENVIRONMENT(2024)
Abstract
Accurately understanding ecosystem service (ES) interactions and an analysis of the complex, multiscale driving mechanisms are foundational prerequisites for implementing effective multiscale ES management. This study dives into the spatial and temporal variations of ES interactions in the Yellow River Basin across four spatial scales. The eXtreme Gradient Boosting (XGBoost) model is later deployed to pinpoint the key drivers of ecosystem services and their indirect pathways to ESs are illuminated utilizing Partial Least Squares-Structural Equation Modeling (PLS-SEM). The results indicate that (1) The synergistic effect between ES pairs in the Yellow River Basin surpasses that of trade-offs. Various types of ecosystem service bundles have transformed into each other from 2000 to 2020, and the spatial patterns of ES interactions bear resemblances at different scales. (2) The factors driving habitat quality (HQ), carbon sequestration (CS), and landscape aesthetics (LA) are mainly the landscape configuration and biophysical conditions. The factor driving food production (FP) is mainly the level of urbanization, whereas soil conservation (SC) and water yield (WY) are mainly subject to climate. (3) When biophysical conditions and level of urbanization serve as mediating variables in pathways, driving factors invariably have negative indirect effects on ESs. When landscape configuration serves as a mediating variable, biophysical conditions positively influence HQ and CS, and negatively impact FP, WY, and LA. Conversely, the level of urbanization negatively affects all ESs. (4) The combination of XGBoost and PLS-SEM offers a comprehensive and innovative lens for analyzing ESs driving mechanisms. Based on our findings, scientific management of ESs should account not only for the direct impacts of driving elements but also for their scale and indirect effects.
MoreTranslated text
Key words
Trade-off/synergies,Ecosystem service bundles,Machine learning model,Indirect effect,Scale effect
AI Read Science
Must-Reading Tree
Example
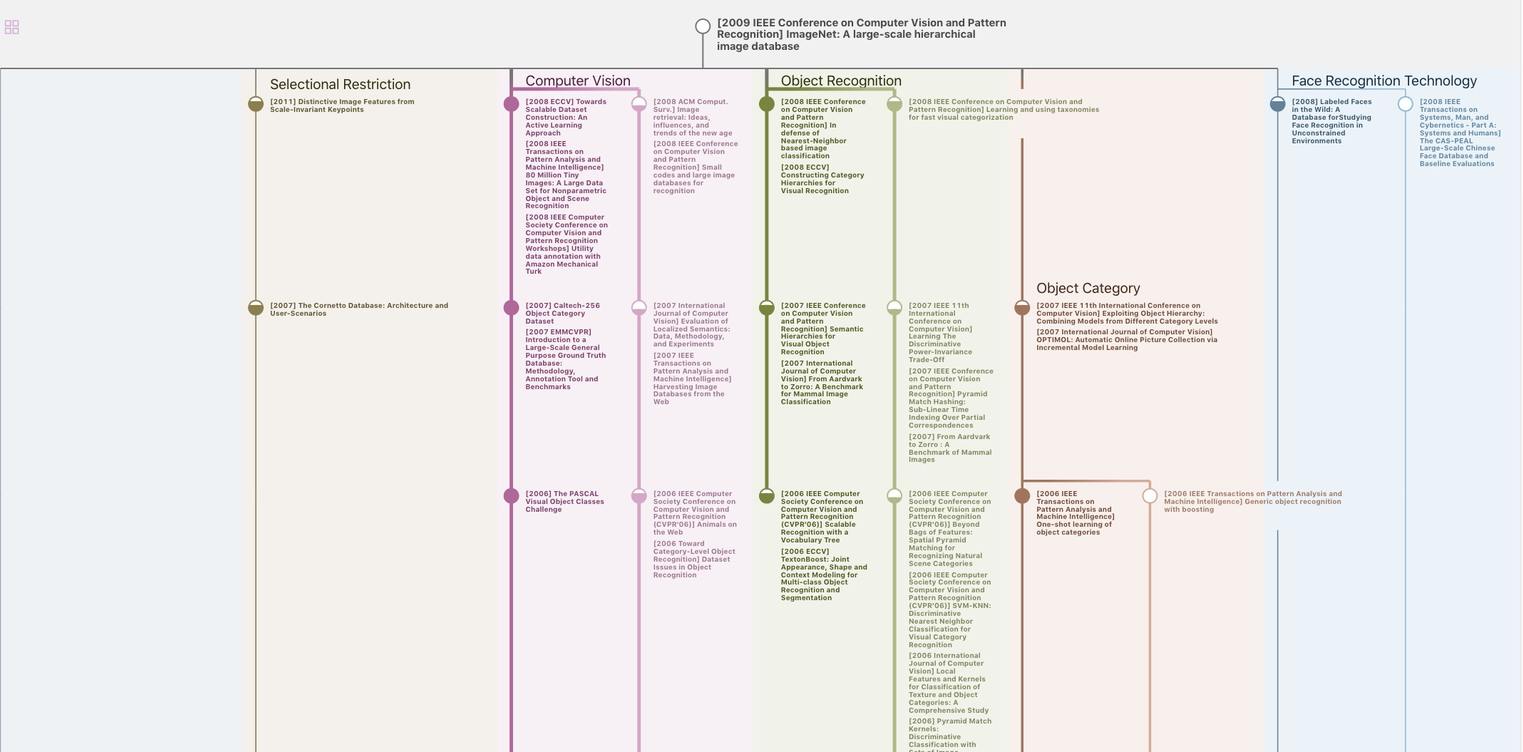
Generate MRT to find the research sequence of this paper
Chat Paper
Summary is being generated by the instructions you defined