Data-Efficient Task Generalization via Probabilistic Model-based Meta Reinforcement Learning
IEEE Robotics and Automation Letters(2023)
摘要
We introduce PACOH-RL, a novel model-based Meta-Reinforcement Learning
(Meta-RL) algorithm designed to efficiently adapt control policies to changing
dynamics. PACOH-RL meta-learns priors for the dynamics model, allowing swift
adaptation to new dynamics with minimal interaction data. Existing Meta-RL
methods require abundant meta-learning data, limiting their applicability in
settings such as robotics, where data is costly to obtain. To address this,
PACOH-RL incorporates regularization and epistemic uncertainty quantification
in both the meta-learning and task adaptation stages. When facing new dynamics,
we use these uncertainty estimates to effectively guide exploration and data
collection. Overall, this enables positive transfer, even when access to data
from prior tasks or dynamic settings is severely limited. Our experiment
results demonstrate that PACOH-RL outperforms model-based RL and model-based
Meta-RL baselines in adapting to new dynamic conditions. Finally, on a real
robotic car, we showcase the potential for efficient RL policy adaptation in
diverse, data-scarce conditions.
更多查看译文
关键词
Reinforcement Learning,Model Learning for Control,Learning from Experience
AI 理解论文
溯源树
样例
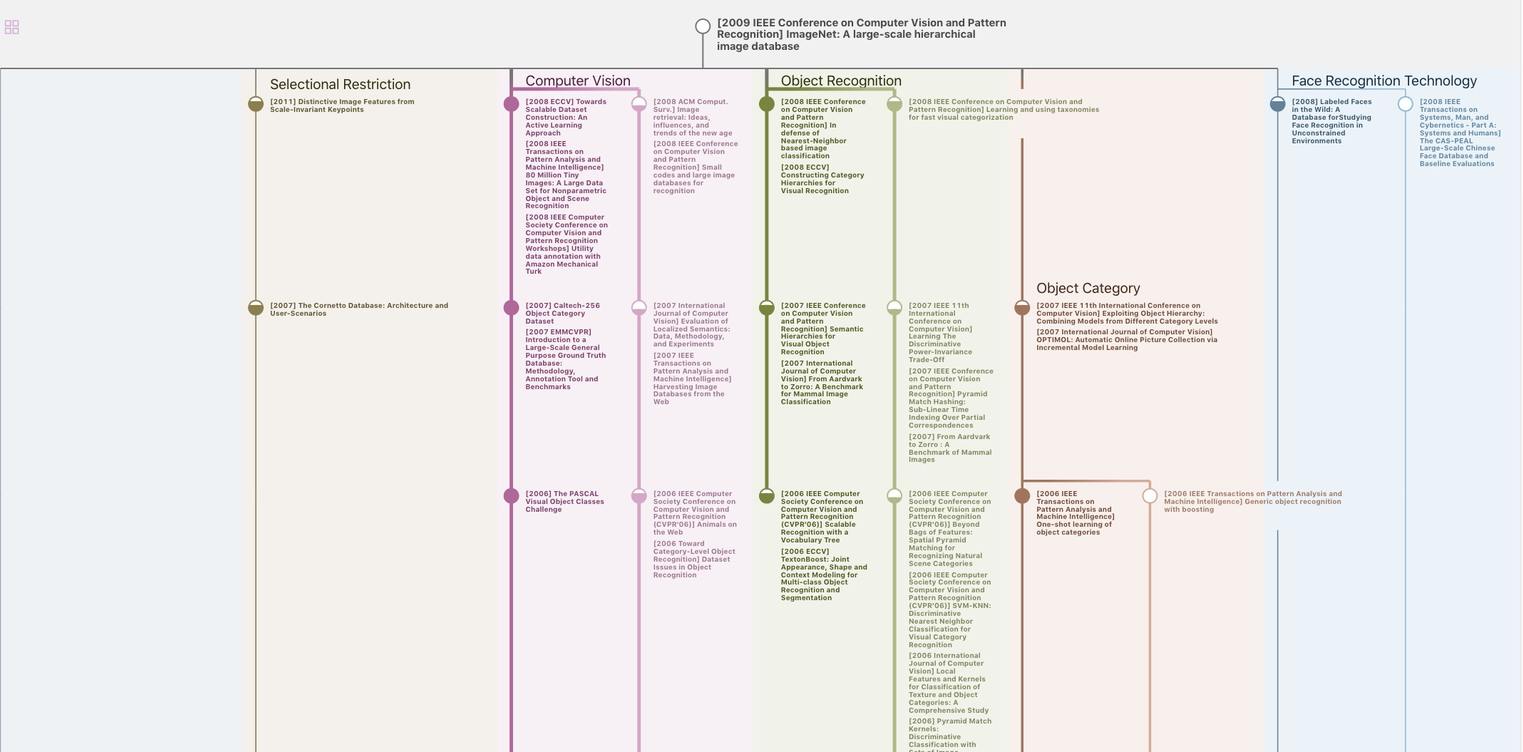
生成溯源树,研究论文发展脉络
Chat Paper
正在生成论文摘要