Probing omics data via harmonic persistent homology
arXiv (Cornell University)(2023)
摘要
Identifying molecular signatures from complex disease patients with
underlying symptomatic similarities is a significant challenge in analysis of
high dimensional multi-omics data. Topological data analysis (TDA) provides a
way of extracting such information from the geometric structure of the data and
identify multiway higher-order relationships. Here, we propose an application
of Harmonic persistent homology which overcomes the limitations of ambiguous
assignment of the topological information to the original elements in a
representative topological cycle from the data. When applied to multi-omics
data, this leads to the discovery of hidden patterns highlighting the
relationships between different omic profiles, while allowing for common tasks
in multiomics analyses such as disease subtyping, and most importantly
biomarker identification for similar latent biological pathways that are
associated with complex diseases. Our experiments on multiple cancer data show
that harmonic persistent homology and TDA can be very useful in dissecting
muti-omics data and identify biomarkers while detecting representative cycles
of the data which also predicts disease subtypes.
更多查看译文
AI 理解论文
溯源树
样例
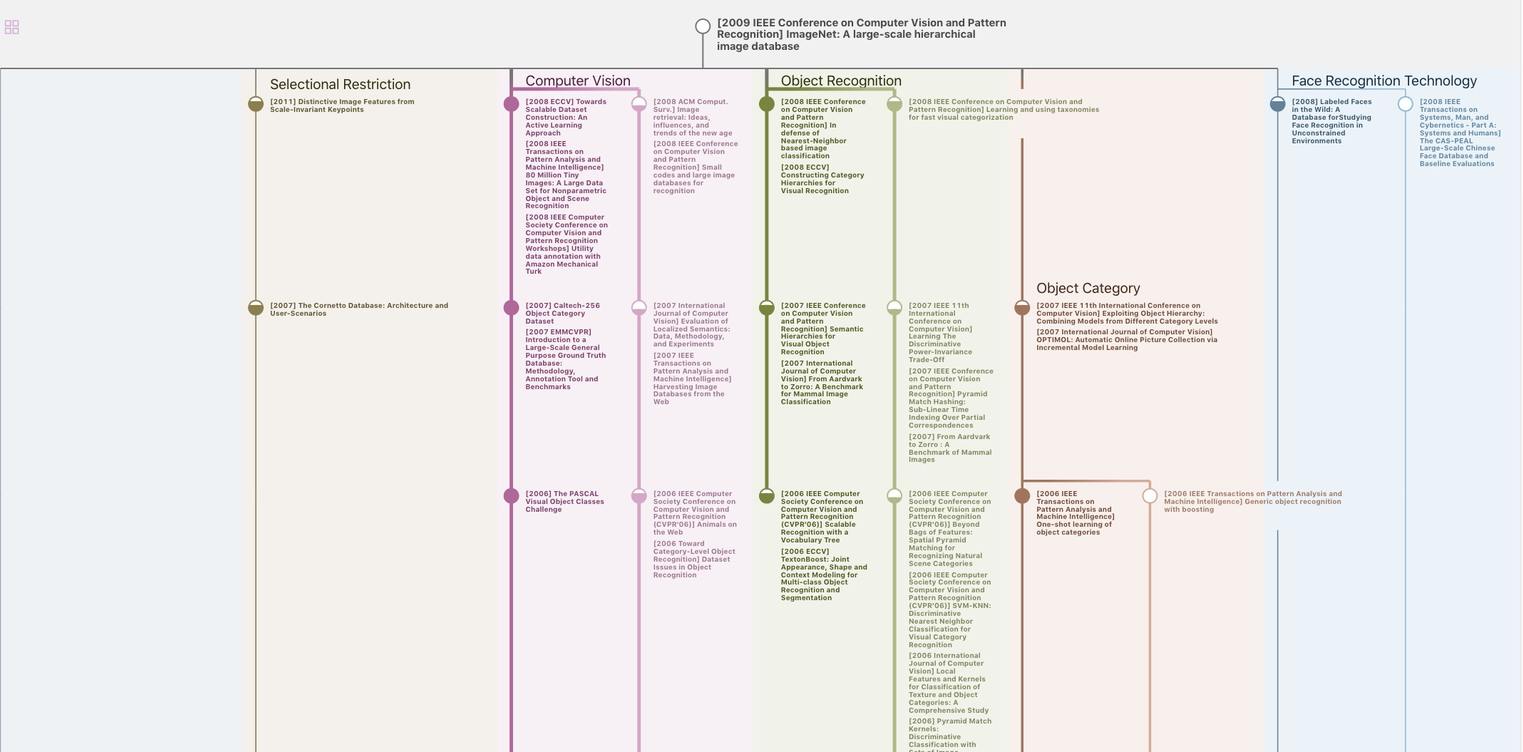
生成溯源树,研究论文发展脉络
Chat Paper
正在生成论文摘要