Learning Arithmetic Formulas in the Presence of Noise: A General Framework and Applications to Unsupervised Learning.
CoRR(2023)
Abstract
We present a general framework for designing efficient algorithms for unsupervised learning problems, such as mixtures of Gaussians and subspace clustering. Our framework is based on a meta algorithm that learns arithmetic circuits in the presence of noise, using lower bounds. This builds upon the recent work of Garg, Kayal and Saha (FOCS 20), who designed such a framework for learning arithmetic circuits without any noise. A key ingredient of our meta algorithm is an efficient algorithm for a novel problem called Robust Vector Space Decomposition. We show that our meta algorithm works well when certain matrices have sufficiently large smallest non-zero singular values. We conjecture that this condition holds for smoothed instances of our problems, and thus our framework would yield efficient algorithms for these problems in the smoothed setting.
MoreTranslated text
AI Read Science
Must-Reading Tree
Example
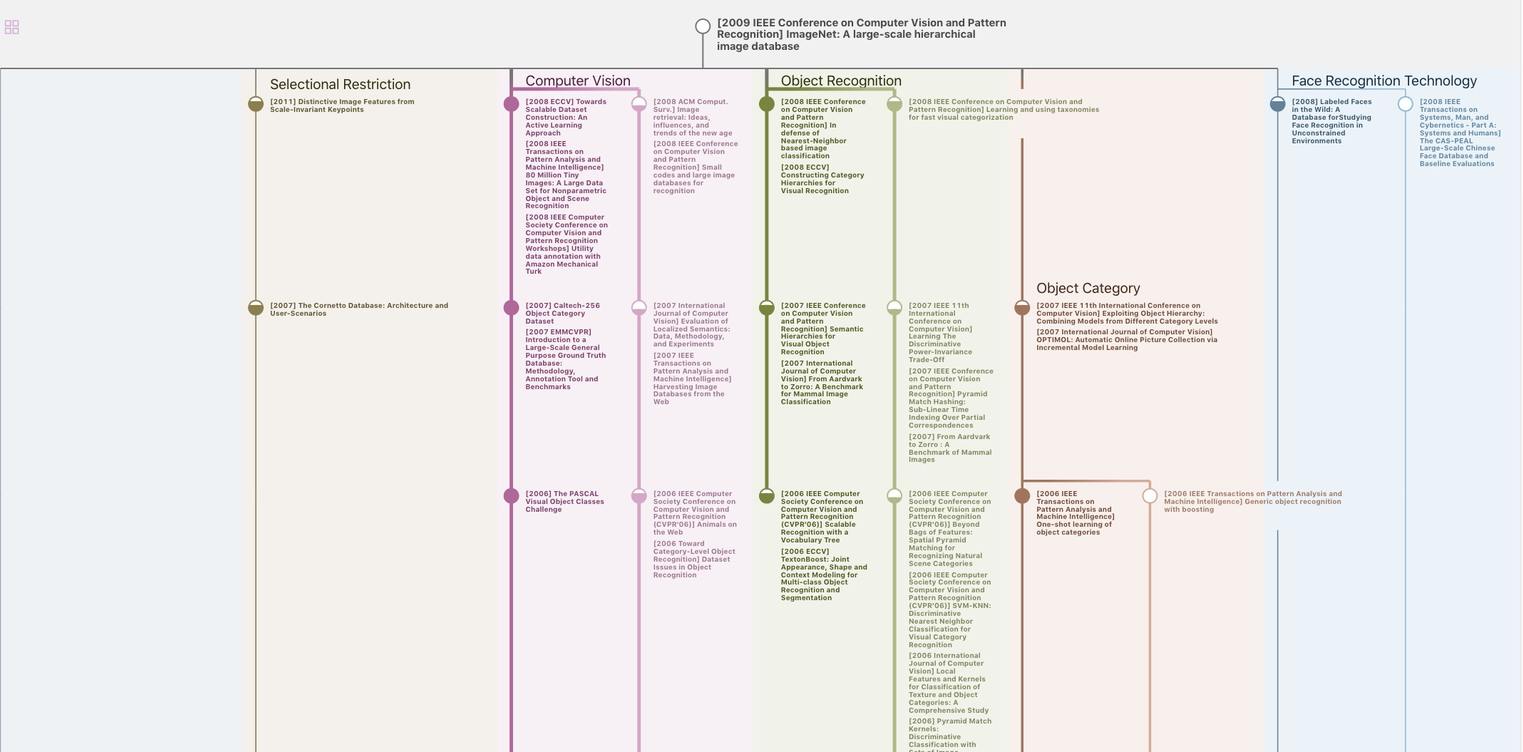
Generate MRT to find the research sequence of this paper
Chat Paper
Summary is being generated by the instructions you defined