Simultaneous Clutter Detection and Semantic Segmentation of Moving Objects for Automotive Radar Data.
CoRR(2023)
摘要
The unique properties of radar sensors, such as their robustness to adverse weather conditions, make them an important part of the environment perception system of autonomous vehicles. One of the first steps during the processing of radar point clouds is often the detection of clutter, i.e. erroneous points that do not correspond to real objects. Another common objective is the semantic segmentation of moving road users. These two problems are handled strictly separate from each other in literature. The employed neural networks are always focused entirely on only one of the tasks. In contrast to this, we examine ways to solve both tasks at the same time with a single jointly used model. In addition to a new augmented multi-head architecture, we also devise a method to represent a network's predictions for the two tasks with only one output value. This novel approach allows us to solve the tasks simultaneously with the same inference time as a conventional task-specific model. In an extensive evaluation, we show that our setup is highly effective and outperforms every existing network for semantic segmentation on the RadarScenes dataset.
更多查看译文
关键词
Semantic Segmentation,Clutter Detection,Neural Network,Point Cloud,Autonomous Vehicles,Inference Time,Radar Sensor,Results Of Experiments,Convolutional Neural Network,Convolutional Layers,State Of The Art,F1 Score,Object Detection,Grid Cells,Individual Tasks,Separate Networks,Inaccurate Measurements,Specular Reflection,Single Label,Grid Map,Small Measurement Error,Network Setup,Large Vehicles,Point Cloud Segmentation,Basic Setup,Advanced Driver Assistance Systems,Object Velocity,Increase In Precision
AI 理解论文
溯源树
样例
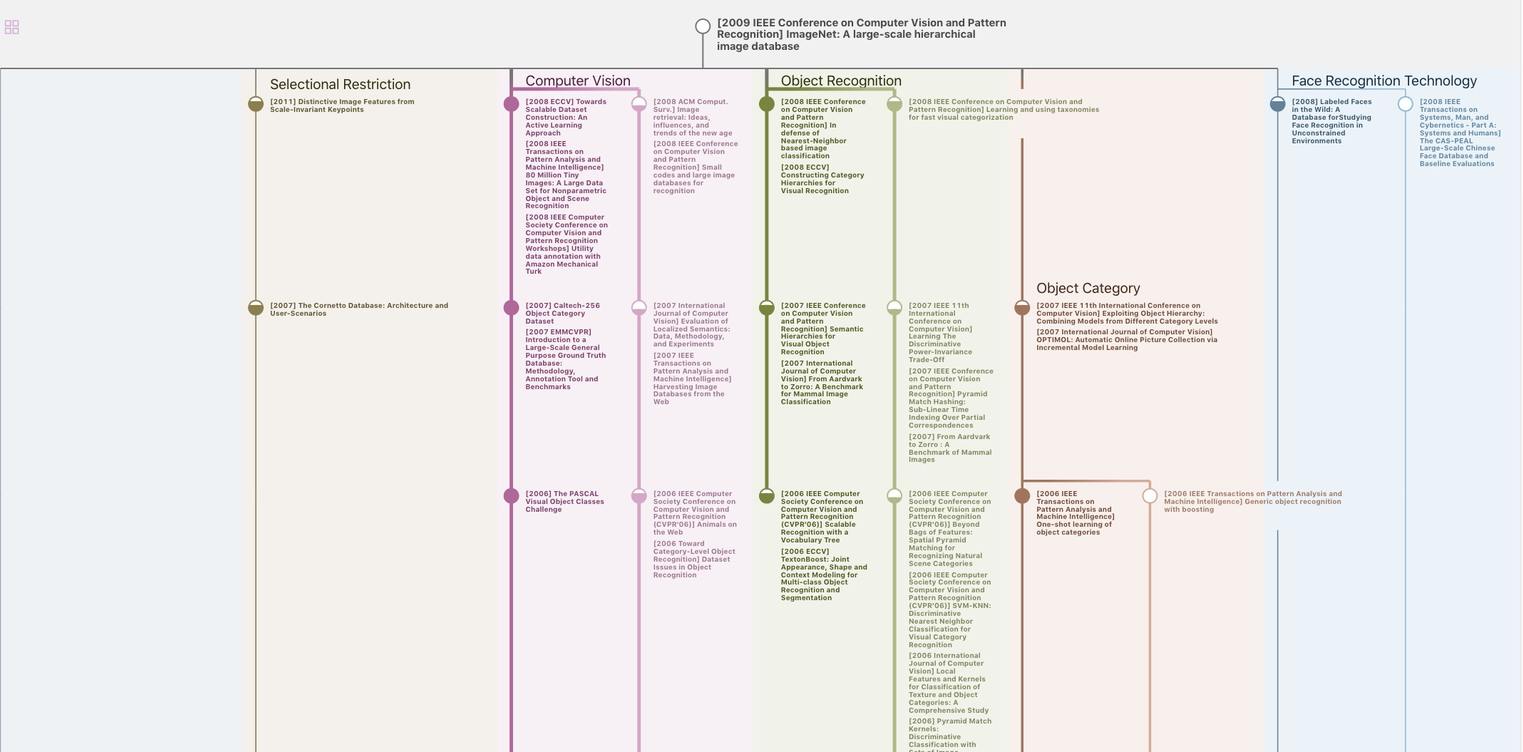
生成溯源树,研究论文发展脉络
Chat Paper
正在生成论文摘要