Discrete Nonparametric Causal Discovery Under Latent Class Confounding
CoRR(2023)
摘要
Directed acyclic graphs are used to model the causal structure of a system.
“Causal discovery” describes the problem of learning this structure from
data. When data is an aggregate from multiple sources (populations or
environments), global confounding obscures conditional independence properties
that drive many causal discovery algorithms. This setting is sometimes known as
a mixture model or a latent class. While some modern methods for causal
discovery are able to work around unobserved confounding in specific cases, the
only known ways to deal with a global confounder involve parametric
assumptions. that are unsuitable for discrete distributions.Focusing on
discrete and non-parametric observed variables, we demonstrate that causal
discovery can still be identifiable under bounded latent classes. The
feasibility of this problem is governed by a trade-off between the cardinality
of the global confounder, the cardinalities of the observed variables, and the
sparsity of the causal structure.
更多查看译文
AI 理解论文
溯源树
样例
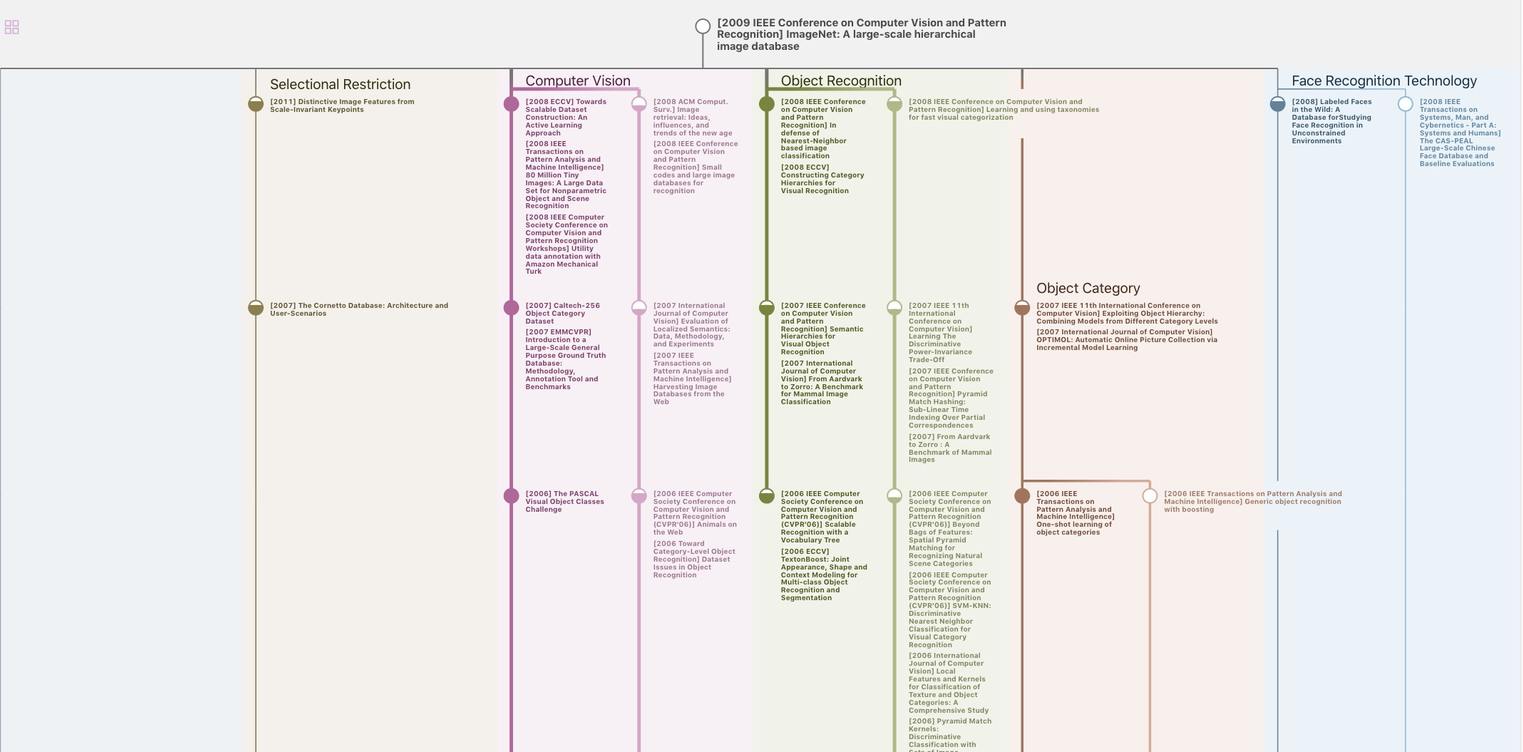
生成溯源树,研究论文发展脉络
Chat Paper
正在生成论文摘要