Explaining Black Boxes With a SMILE: Statistical Model-Agnostic Interpretability With Local Explanations
IEEE SOFTWARE(2024)
摘要
Explainability is a key aspect of improving trustworthiness. We therefore propose SMILE, a new method that builds on previous approaches by making use of statistical distance measures to improve explainability while remaining applicable to a wide range of input data domains.
更多查看译文
关键词
Closed Box,Perturbation Methods,Predictive Models,Gaussian Distribution,Data Models,Machine Learning,Training,Object Object,Use Of Measures,Statistical Measures,Wide Range Of Domains,Growth In Recent Years,Statistical Distance,Variety Of Supports,Linear Model Object Object,Alternative Models Object Object,Model Coefficients,Maximum Distance,Intersection Over Union Object Object Object Object Object Object,Kernel Function,Input Samples,Light Signal Object Object,Part Of The Image,Adversarial Attacks,Random Perturbations,Perturbation Vector,Human Intuition,Game Theory Object Object,Understanding Of Models
AI 理解论文
溯源树
样例
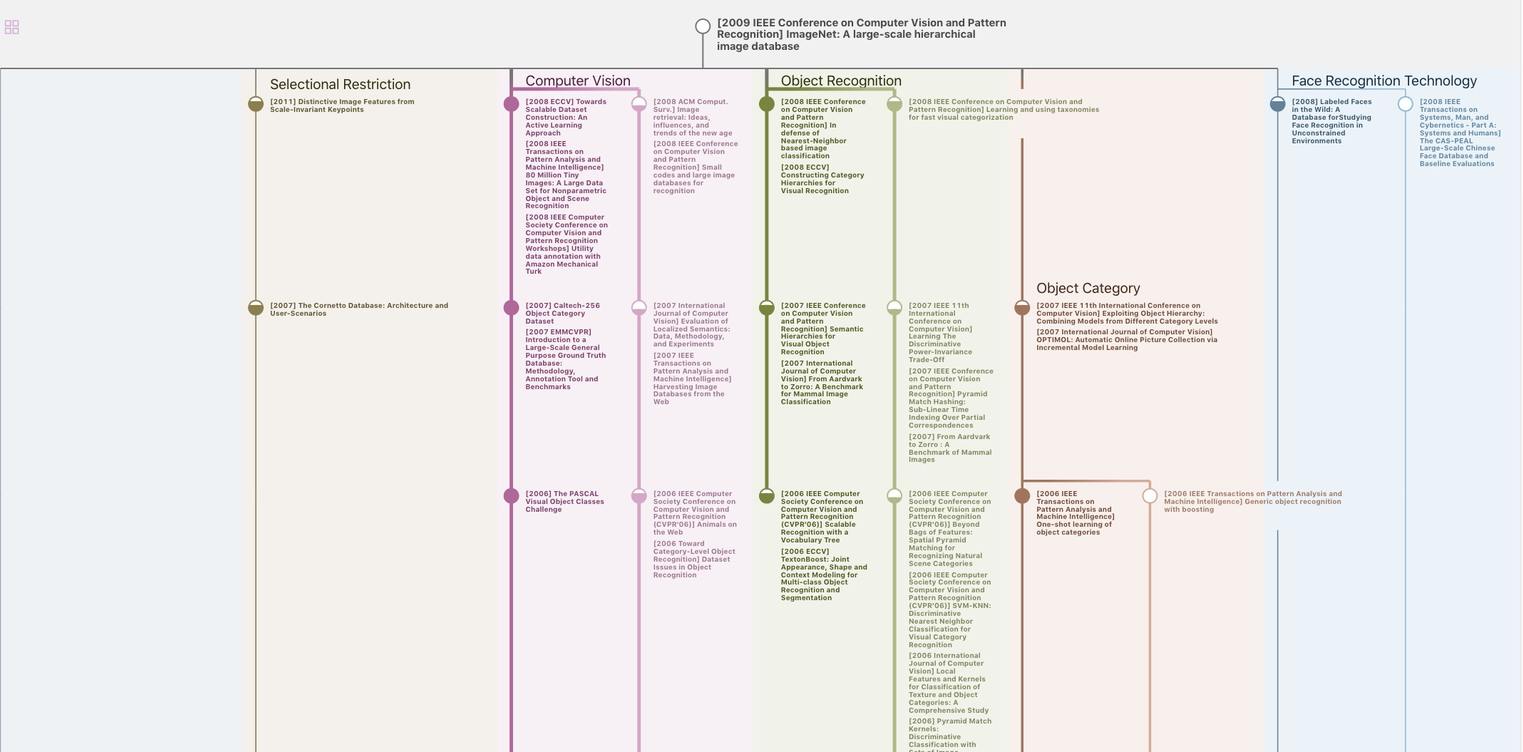
生成溯源树,研究论文发展脉络
Chat Paper
正在生成论文摘要