Learning Control Policies of Hodgkin-Huxley Neuronal Dynamics.
CoRR(2023)
摘要
We present a neural network approach for closed-loop deep brain stimulation (DBS). We cast the problem of finding an optimal neurostimulation strategy as a control problem. In this setting, control policies aim to optimize therapeutic outcomes by tailoring the parameters of a DBS system, typically via electrical stimulation, in real time based on the patient's ongoing neuronal activity. We approximate the value function offline using a neural network to enable generating controls (stimuli) in real time via the feedback form. The neuronal activity is characterized by a nonlinear, stiff system of differential equations as dictated by the Hodgkin-Huxley model. Our training process leverages the relationship between Pontryagin's maximum principle and Hamilton-Jacobi-Bellman equations to update the value function estimates simultaneously. Our numerical experiments illustrate the accuracy of our approach for out-of-distribution samples and the robustness to moderate shocks and disturbances in the system.
更多查看译文
AI 理解论文
溯源树
样例
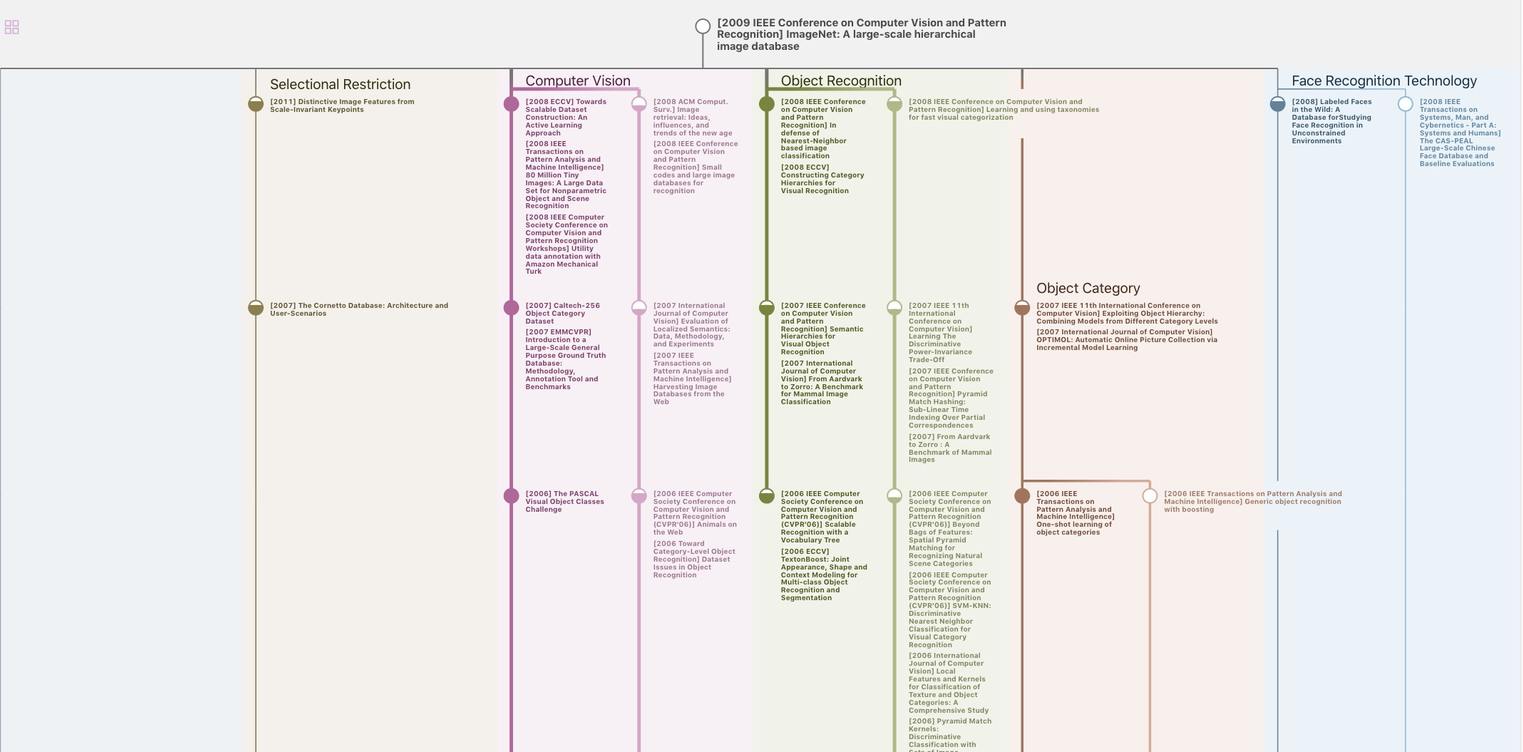
生成溯源树,研究论文发展脉络
Chat Paper
正在生成论文摘要