An inexact $q$-order regularized proximal Newton method for nonconvex composite optimization
arXiv (Cornell University)(2023)
摘要
This paper concerns the composite problem of minimizing the sum of a twice continuously differentiable function $f$ and a nonsmooth convex function. For this class of nonconvex and nonsmooth problems, by leveraging a practical inexactness criterion and a novel selection strategy for iterates, we propose an inexact $q\in[2,3]$-order regularized proximal Newton method, which becomes an inexact cubic regularization (CR) method for $q=3$. We justify that its iterate sequence converges to a stationary point for the KL objective function, and if the objective function has the KL property of exponent $\theta\in(0,\frac{q-1}{q})$, the convergence has a local $Q$-superlinear rate of order $\frac{q-1}{\theta q}$. In particular, under a locally H\"{o}lderian error bound of order $\gamma\in(\frac{1}{q-1},1]$ on a second-order stationary point set, the iterate sequence converges to a second-order stationary point with a local $Q$-superlinear rate of order $\gamma(q\!-\!1)$, which is specified as $Q$-quadratic rate for $q=3$ and $\gamma=1$. This is the first practical inexact CR method with $Q$-quadratic convergence rate for nonconvex composite optimization. We validate the efficiency of the proposed method with ZeroFPR as the solver of subproblems by applying it to convex and nonconvex composite problems with a highly nonlinear $f$.
更多查看译文
AI 理解论文
溯源树
样例
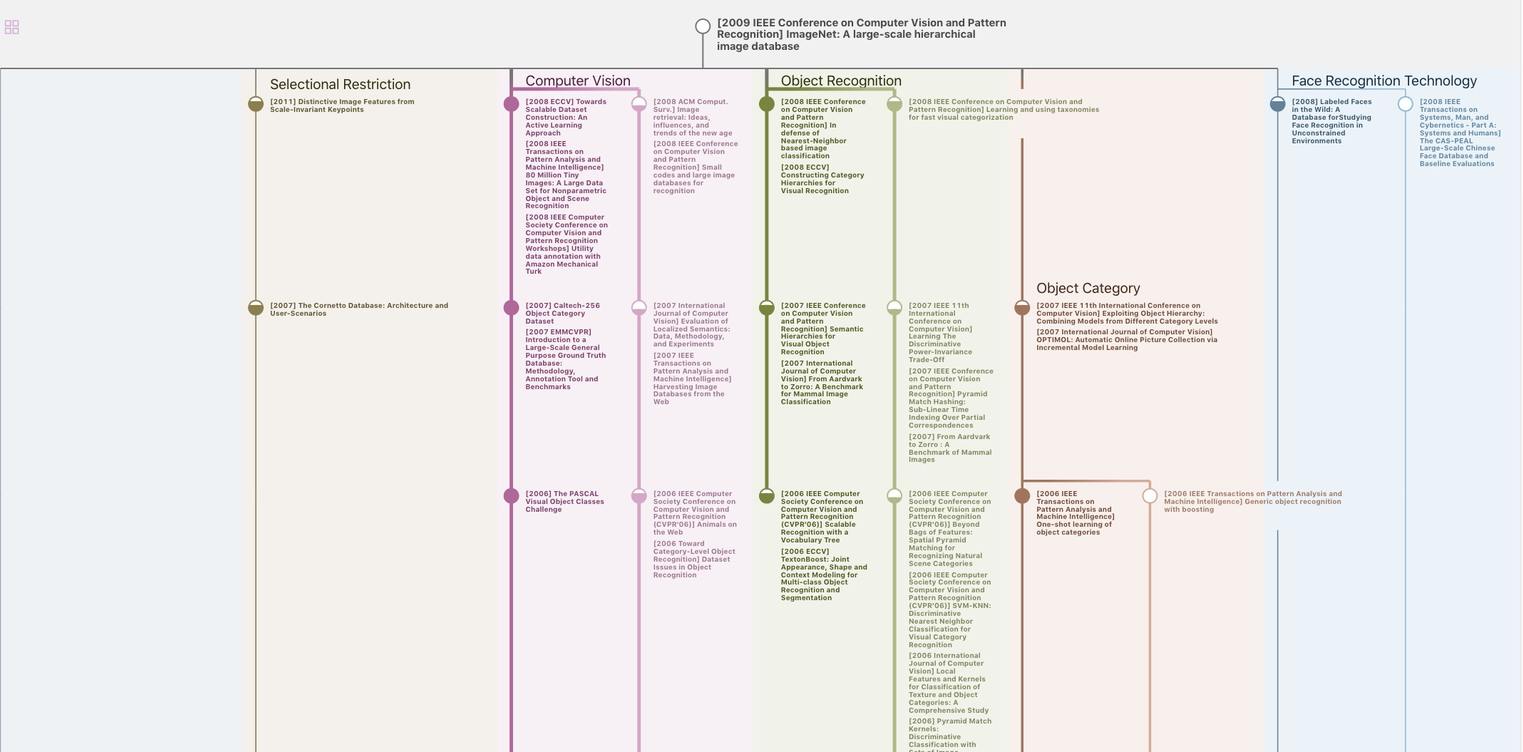
生成溯源树,研究论文发展脉络
Chat Paper
正在生成论文摘要