Dopamine neurons encode a multidimensional probabilistic map of future reward
bioRxiv (Cold Spring Harbor Laboratory)(2023)
摘要
Learning to predict rewards is a fundamental driver of adaptive behavior. Midbrain dopamine neurons (DANs) play a key role in such learning by signaling reward prediction errors (RPEs) that teach recipient circuits about expected rewards given current circumstances and actions. However, the algorithm that DANs are thought to provide a substrate for, temporal difference (TD) reinforcement learning (RL), learns the mean of temporally discounted expected future rewards, discarding useful information concerning experienced distributions of reward amounts and delays. Here we present time-magnitude RL (TMRL), a multidimensional variant of distributional reinforcement learning that learns the joint distribution of future rewards over time and magnitude using an efficient code that adapts to environmental statistics. In addition, we discovered signatures of TMRL-like computations in the activity of optogenetically identified DANs in mice during a classical conditioning task. Specifically, we found significant diversity in both temporal discounting and tuning for the magnitude of rewards across DANs, features that allow the computation of a two dimensional, probabilistic map of future rewards from just 450ms of neural activity recorded from a population of DANs in response to a reward-predictive cue. In addition, reward time predictions derived from this population code correlated with the timing of anticipatory behavior, suggesting the information is used to guide decisions regarding when to act. Finally, by simulating behavior in a foraging environment, we highlight benefits of access to a joint probability distribution of reward over time and magnitude in the face of dynamic reward landscapes and internal physiological need states. These findings demonstrate surprisingly rich probabilistic reward information that is learned and communicated to DANs, and suggest a simple, local-in-time extension of TD learning algorithms that explains how such information may be acquired and computed.
### Competing Interest Statement
The authors have declared no competing interest.
更多查看译文
关键词
multidimensional probabilistic map,future
AI 理解论文
溯源树
样例
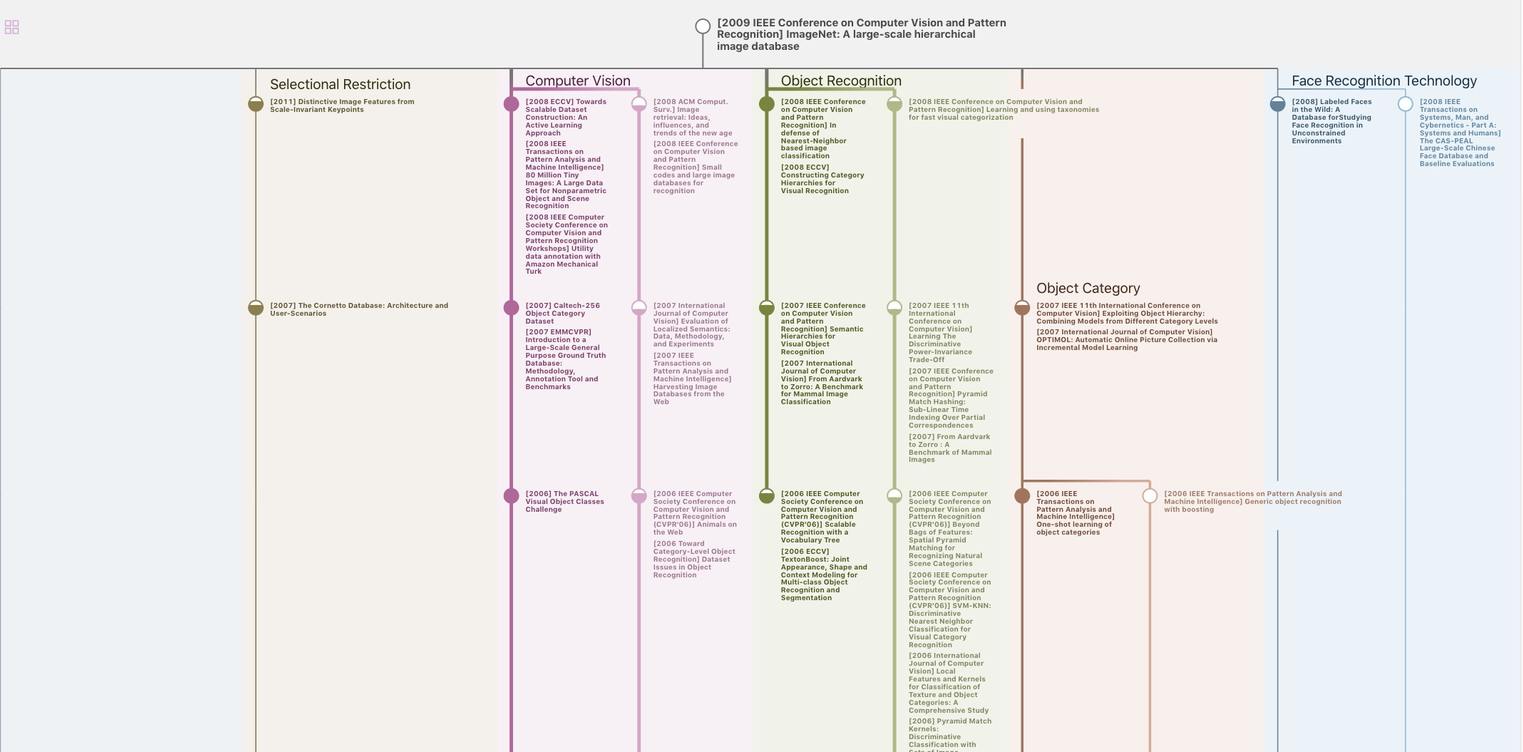
生成溯源树,研究论文发展脉络
Chat Paper
正在生成论文摘要