Exploiting Conversation-Branch-Tweet HyperGraph Structure to Detect Misinformation on Social Media
ACM TRANSACTIONS ON KNOWLEDGE DISCOVERY FROM DATA(2024)
Abstract
The spread of misinformation on social media is a serious issue that can have negative consequences for public health and political stability. While detecting and identifying misinformation can be challenging, many attempts have been made to address this problem. However, traditional models that focus on pairwise relationships on misinformation propagation paths may not be effective in capturing the underlying connections among multiple tweets. To address this limitation, the proposed "Conversation-Branch-Tweet" hypergraph convolutional network (CBT-HGCN) uses a hypergraph to represent the internal structure and content of tweet data, with tweets and their replies viewed as nodes and hyperedges, respectively. The model first pre-processes the tweets of a conversation and then uses a pre-trained model as an encoder to extract node information. Finally, a hypergraph convolution network is used as an information fuser for classification. Experimental results on three benchmark datasets (Twitter15, Twitter16, and Pheme) show that the proposed model outperforms several strong baseline models and achieves state-of-the-art performance. This indicates that the CBT-HGCN approach is effective in detecting and identifying misinformation on social media by capturing the underlying connections among multiple tweets.
MoreTranslated text
Key words
Misinformation detection,social media,graph neural network,hypergraph modeling
AI Read Science
Must-Reading Tree
Example
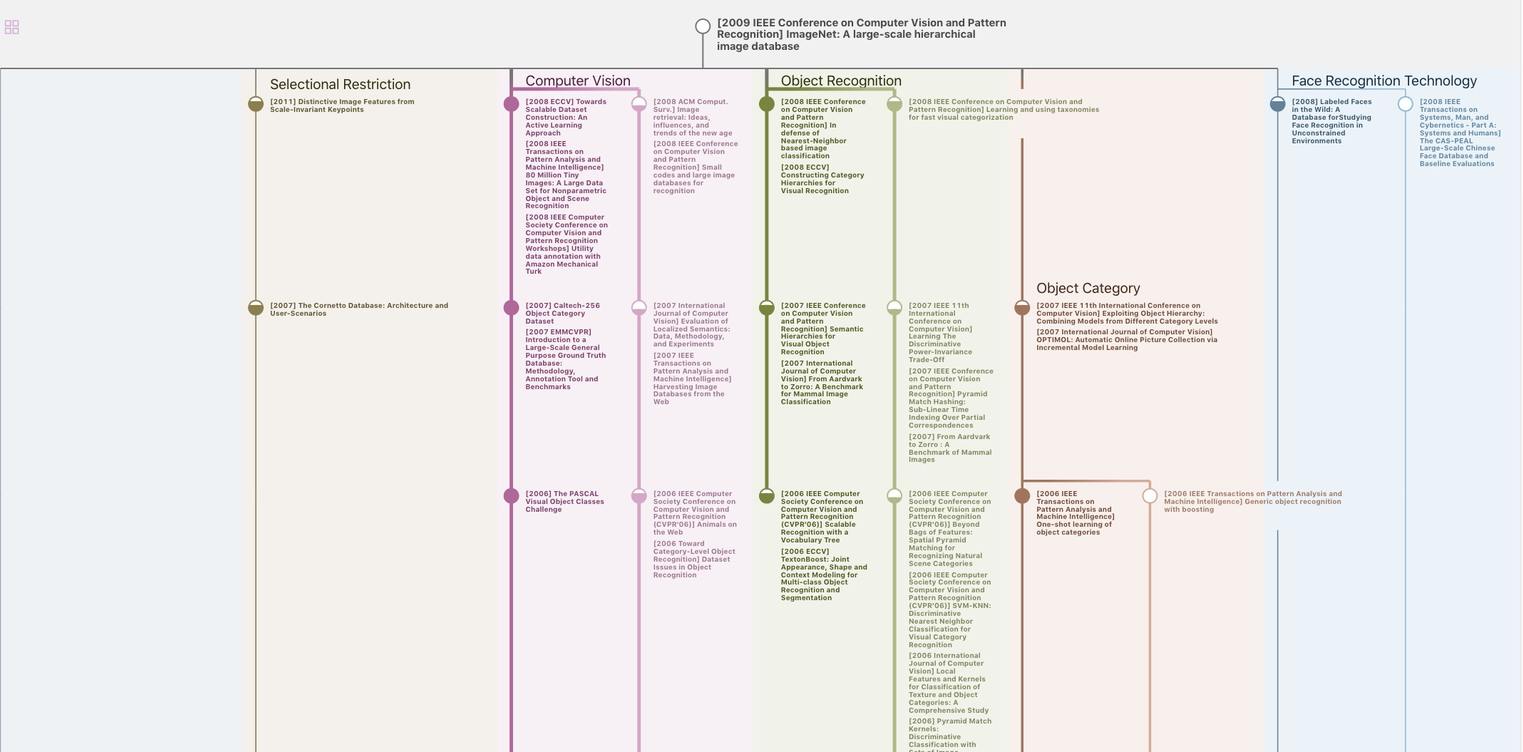
Generate MRT to find the research sequence of this paper
Chat Paper
Summary is being generated by the instructions you defined