Learning material synthesis-structure-property relationship by data fusion: Bayesian Co-regionalization N-Dimensional Piecewise Function Learning
CoRR(2023)
摘要
Advanced materials are needed to further next-generation technologies such as quantum computing, carbon capture, and low-cost medical imaging. However, advanced materials discovery is confounded by two fundamental challenges: the challenge of a high-dimensional, complex materials search space and the challenge of combining knowledge, i.e., data fusion across instruments and labs. To overcome the first challenge, researchers employ knowledge of the underlying material synthesis-structure-property relationship, as a material's structure is often predictive of its functional property and vice versa. For example, optimal materials often occur along composition-phase boundaries or within specific phase regions. Additionally, knowledge of the synthesis-structure-property relationship is fundamental to understanding underlying physical mechanisms. However, quantifying the synthesis-structure-property relationship requires overcoming the second challenge. Researchers must merge knowledge gathered across instruments, measurement modalities, and even laboratories. We present the Synthesis-structure-property relAtionship coreGionalized lEarner (SAGE) algorithm. A fully Bayesian algorithm that uses multimodal coregionalization to merge knowledge across data sources to learn synthesis-structure-property relationships.
更多查看译文
关键词
fusion,learning,synthesis-structure-property,co-regionalization,n-dimensional
AI 理解论文
溯源树
样例
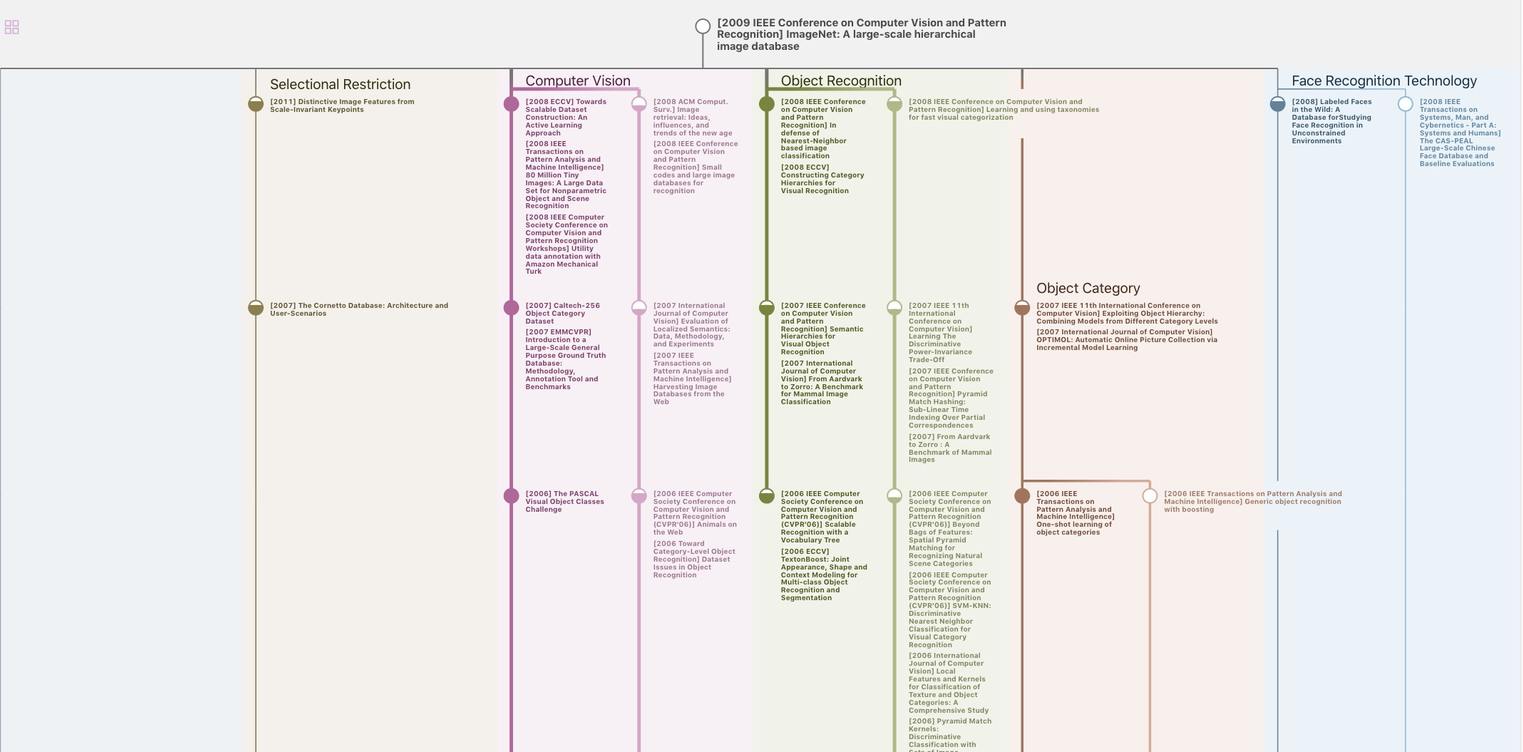
生成溯源树,研究论文发展脉络
Chat Paper
正在生成论文摘要