Multi-Discriminator Active Adversarial Network for Multi-Center Brain Disease Diagnosis
IEEE TRANSACTIONS ON BIG DATA(2023)
Abstract
Multi-center analysis has attracted increasing attention in brain disease diagnosis, because it provides effective approaches to improve disease diagnostic performance by making use of the information from different centers. However, in practical multi-center applications, data uncertainty is more common than that in single center, which brings challenge to robust modeling of diagnosis. In this article, we proposed a multi-discriminator active adversarial network (MDAAN) to alleviate the uncertainties at the center, feature, and label levels for multi-center brain disease diagnosis. First, we extract the latent invariant representation of the source center and target center to reduce domain shift by adversarial learning strategy. Second, the proposed method adaptively evaluates the contribution of different source centers in fusion by measuring data distribution difference between source and target center. Moreover, only the hard learning samples in target center are identified to label with low sample annotation cost. Finally, we treat the selected samples as the auxiliary domain to alleviate the negative transfer and improve the robustness of the multi-center model. We extensively compare the proposed approach with several state-of-the-art multi-center methods on the five-center schizophrenia dataset, and the results demonstrate that our method is superior to the previous methods in identifying brain disease.
MoreTranslated text
Key words
Uncertainty,Diseases,Medical diagnosis,Functional magnetic resonance imaging,Brain modeling,Adaptation models,Mental disorders,Multi-center analysis,adversarial domain adaptation,active learning,brain disease diagnosis
AI Read Science
Must-Reading Tree
Example
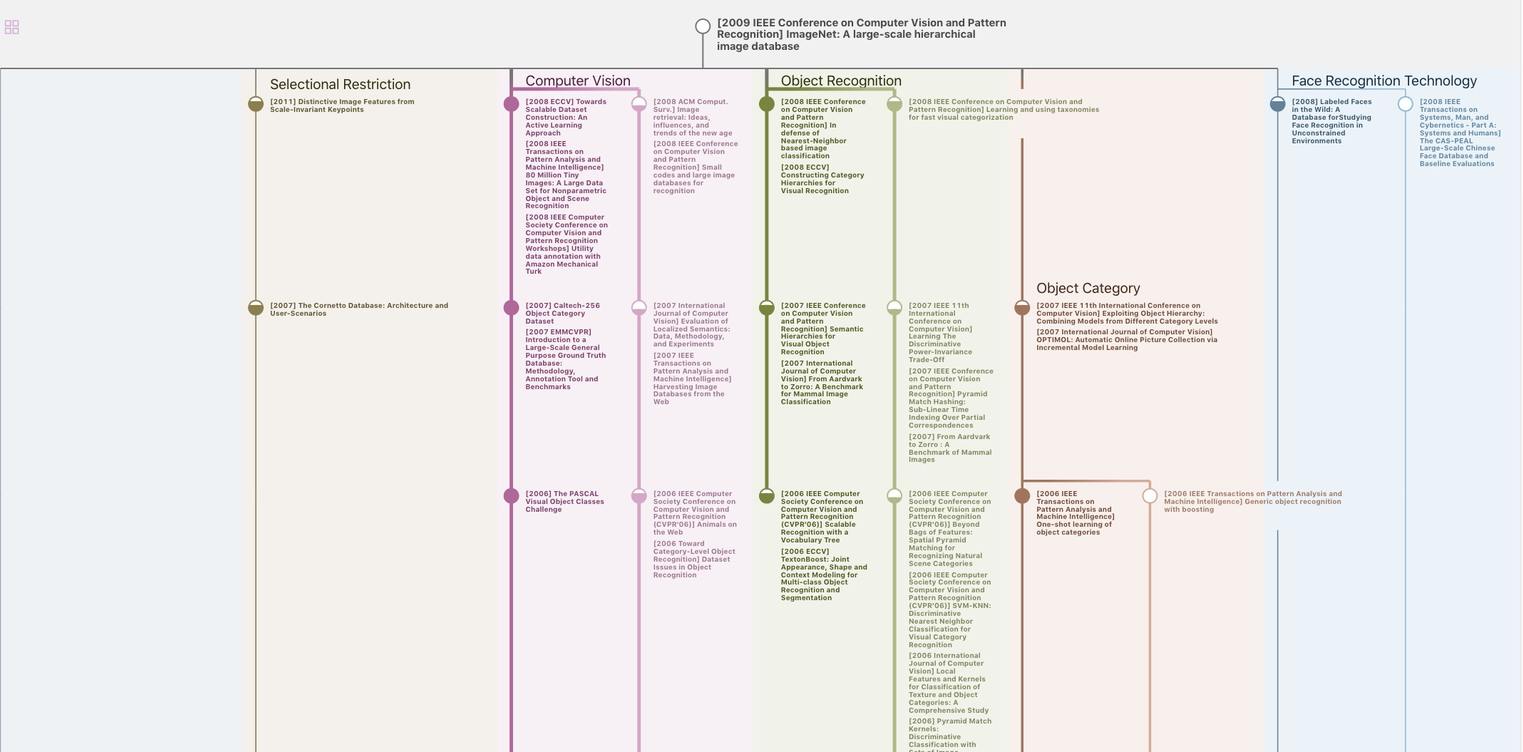
Generate MRT to find the research sequence of this paper
Chat Paper
Summary is being generated by the instructions you defined