An Analysis of Graph Neural Network Memory Access Patterns.
SC-W '23: Proceedings of the SC '23 Workshops of The International Conference on High Performance Computing, Network, Storage, and Analysis(2023)
摘要
Graph Neural Networks (GNNs) are becoming increasingly popular for applying neural networks to graph data. However, as the size of the input graph increases, the GPU memory wall problem becomes an important issue. Since both current solutions to reduce the memory footprint, such as mini-batch approaches and the use of memory-efficient tensor manipulations, have drawbacks, we attempt to solve the problem by expanding the memory size using a virtual memory technology. To overcome the data transfer overhead of virtual memory technology, in this paper we focus on analyzing the memory access pattern of GNNs with the goal of reducing the data transfer latency perceived by the user. A preliminary result of applying optimization techniques guided by our analysis results shows a 40% reduction in the execution time of a combination of training and testing.
更多查看译文
AI 理解论文
溯源树
样例
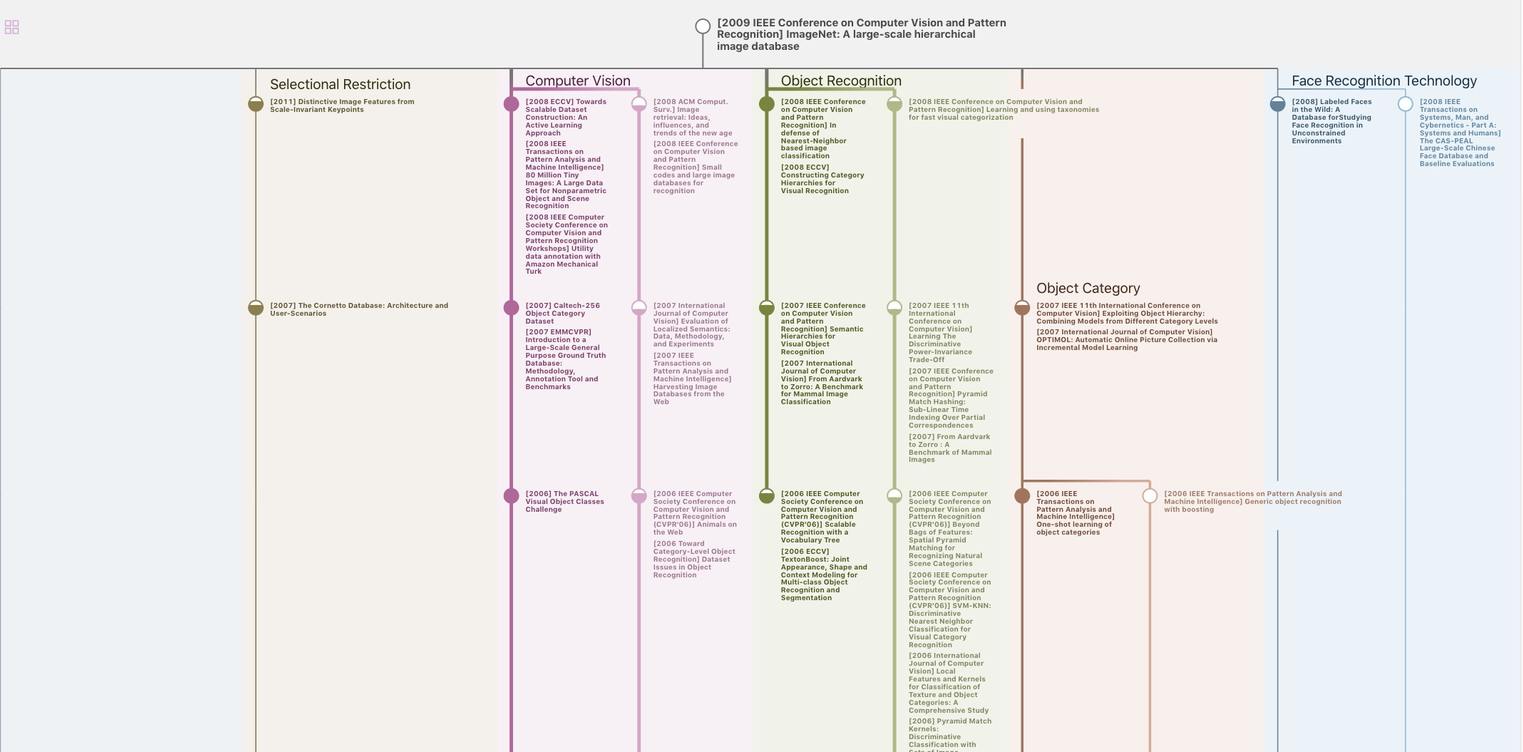
生成溯源树,研究论文发展脉络
Chat Paper
正在生成论文摘要