Predicting the Risk of Developing Type 1 Diabetes Using a One-Week Continuous Glucose Monitoring Home Test With Classification Enhanced by Machine-Learning: An Exploratory Study
JOURNAL OF DIABETES SCIENCE AND TECHNOLOGY(2023)
摘要
Background: Detection of two or more autoantibodies (Ab) in the blood might describe those individuals at increased risk of developing type 1 diabetes (T1D) during the following years. The aim of this exploratory study is to propose a high versus low T1D risk classifier using machine-learning technology based on continuous glucose monitoring (CGM) home data. Methods: Forty-two healthy relatives of people with T1D with mean +/- SD age of 23.8 +/- 10.5 years, HbA1c (glycated hemoglobin) of 5.3% +/- 0.3%, and BMI (body mass index) of 23.2 +/- 5.2 kg/m(2) with zero (low risk; N = 21), and >= 2 (high risk; N = 21) Ab, were enrolled in an NIH (National Institutes of Health)-funded TrialNet ancillary study. Participants wore a CGM for a week and consumed three standardized liquid mixed meals (SLMM) instead of three breakfasts. Glycemic features were extracted from two-hour post-SLMM CGM traces, compared across groups, and used in four supervised machine-learning Ab risk status classifiers. Recursive Feature Elimination (RFE) algorithm was used for feature selection; classifiers were evaluated through 10-fold cross-validation, using the receiver operating characteristic area under the curve (AUC-ROC) to select the best classification model. Results: The percent time of glucose >180 mg/dL (T180), glucose range, and glucose CV (coefficient of variation) were the only significant differences between the glycemic features in the two groups with P values of .040, .035, and .028 respectively. The linear SVM (Support Vector Machine) model with RFE features achieved the best performance of classifying low-risk versus high-risk individuals with AUC-ROC = 0.88. Conclusions: A machine-learning technology, combining a potentially self-administered one-week CGM home test, has the potential to reliably assess the T1D risk.
更多查看译文
关键词
continuous glucose monitoring,islet autoantibodies,machine-learning,prediabetes,type 1 diabetes
AI 理解论文
溯源树
样例
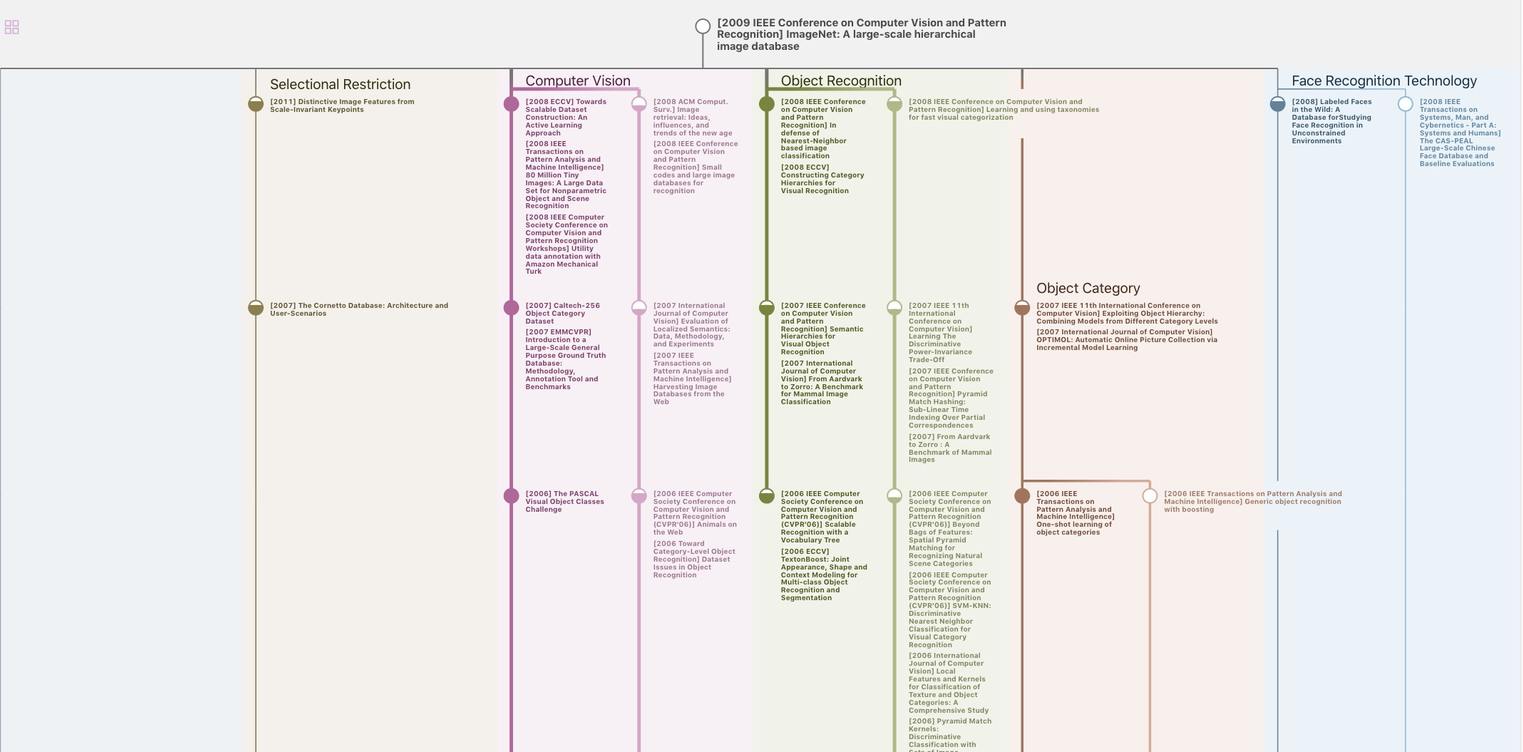
生成溯源树,研究论文发展脉络
Chat Paper
正在生成论文摘要