970 Multiplex-immunofluorescence-based spatial characterization of the tumor-microenvironment of a large bicentric clinical non-small cell lung cancer cohort
Journal for ImmunoTherapy of Cancer(2023)
摘要
Background
Non-small-cell lung cancer (NSCLC) is the leading cause for cancer death. Current targeted- and immuno-therapies are effective in a patient subset, but causes for resistance and the complexity of the immune reaction are difficult to be identified on a single patient level. It is hypothesized that the tumor microenvironment (TME) and its immune cell spatial composition are a key to alleviate that. Arguably, a sufficiently large study cohort, a high-plex (immune) cell characterization and an accordingly scalable analysis is required to advance the discovery of complex patterns. In this study, we tackle this by means of a large NSCLC cohort, a multiplex panel consisting of hematoxylin and eosin (H&E) and 12 immunofluorescence markers (mIF), and a scalable AI-based image analysis approach, combining histomorphological and multiplex immunofluorescence (mIF) data to characterize the TME for detection of prognostic and predictive biomarkers.Methods
We gathered formalin-fixed, paraffin-embedded tissue and clinicopathological data from 1168 patients with resected stage I-IV NSCLCs from two large German hospitals (Charité Berlin and University Hospital Cologne). Four 1.5 mm tissue cores were punched from tumor regions of each case for constructing a tissue microarray. Sections were stained with a 12-plex mIF panel followed by H&E. All stains were scanned and co-registered at single cell accuracy. Deep learning models were developed to detect the different tumor regions: carcinoma, stroma, and necrosis from H&E, and different IF markers from mIF and H&E. The marker-wise cell classification predictions were combined into different cell subtypes. The spatially resolved readouts characterized the TME and were correlated with clinical data.Results
The tissue segmentation model achieved a F1-score of 92%. The cell classification models predict positivity for 12 markers with an F1-score of at least 95% on hold-out data. The predictions were combined to quantify 42 different cell subtypes and overall 53 million cells were characterized. The extracted data confirms the known result of a high density of TREG in the tumor stroma indicating a shorter disease-free survival. Further analysis revealed that data-driven immune cell signatures can be identified separating patient subgroups with respect to overall survival.Conclusions
In our study we demonstrate that the combination of a large cohort, high-plex panel, and automated AI-based analysis has the potential to discover complex, predictive TME signatures. Initial results validate TREG as a known biomarker and suggest that the approach indeed allows for a data-driven TME pattern discovery with clinical impact as a potential stratification tool for future clinical trials.Ethics Approval
This study was approved by Charité Berlin’s Ethics Board with approval number EA1/243/21.查看译文
关键词
tumor-microenvironment tumor-microenvironment,lung cancer,multiplex-immunofluorescence-based,non-small
AI 理解论文
溯源树
样例
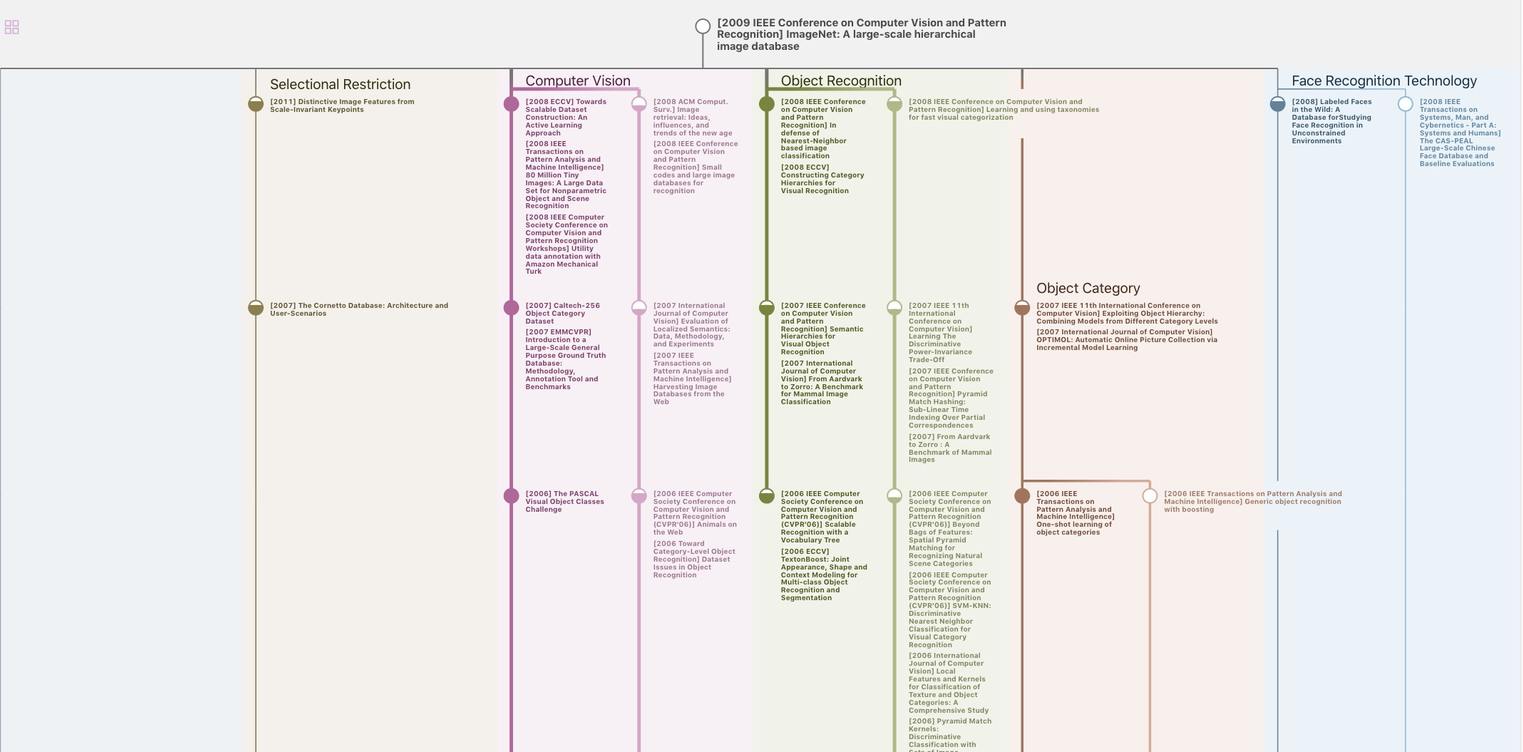
生成溯源树,研究论文发展脉络
Chat Paper
正在生成论文摘要