Prediction of Radiation Treatment Response for Locally Advanced Rectal Cancer via a Longitudinal Trend Analysis Framework on Cone-Beam CT
CANCERS(2023)
摘要
Simple Summary Locally advanced rectal cancer (LARC) is commonly treated with neoadjuvant chemoradiation (CRT) followed by total mesorectal excision (TME). Patients respond to the CRT differently due to inter- and intra- patient variability in radiosensitivity. 15-27% of patients completely respond to the CRT and achieve pathologic complete response (pCR), while a large proportion of patients have a partial or poor response. TME is a highly invasive procedure, which can cause overtreatment, leading to morbidity and even mortality for the LARC patients who had a pCR. This work introduces an integrated framework to inform clinical decision making through systematical analysis of longitudinal Cone Beam Computed Tomography (CBCT) during a treatment course. The effectiveness and robustness of the response prediction model, based on quantitative imaging features derived from targeted volume from pre- and during-treatment images, were validated on a retrospective cohort of LARC patients towards personalized treatment.Abstract Locally advanced rectal cancer (LARC) presents a significant challenge in terms of treatment management, particularly with regards to identifying patients who are likely to respond to radiation therapy (RT) at an individualized level. Patients respond to the same radiation treatment course differently due to inter- and intra-patient variability in radiosensitivity. In-room volumetric cone-beam computed tomography (CBCT) is widely used to ensure proper alignment, but also allows us to assess tumor response during the treatment course. In this work, we proposed a longitudinal radiomic trend (LRT) framework for accurate and robust treatment response assessment using daily CBCT scans for early detection of patient response. The LRT framework consists of four modules: (1) Automated registration and evaluation of CBCT scans to planning CT; (2) Feature extraction and normalization; (3) Longitudinal trending analyses; and (4) Feature reduction and model creation. The effectiveness of the framework was validated via leave-one-out cross-validation (LOOCV), using a total of 840 CBCT scans for a retrospective cohort of LARC patients. The trending model demonstrates significant differences between the responder vs. non-responder groups with an Area Under the Curve (AUC) of 0.98, which allows for systematic monitoring and early prediction of patient response during the RT treatment course for potential adaptive management.
更多查看译文
关键词
locally advanced rectal cancer,cone-beam computed tomography,longitudinal trending analysis,radiomics
AI 理解论文
溯源树
样例
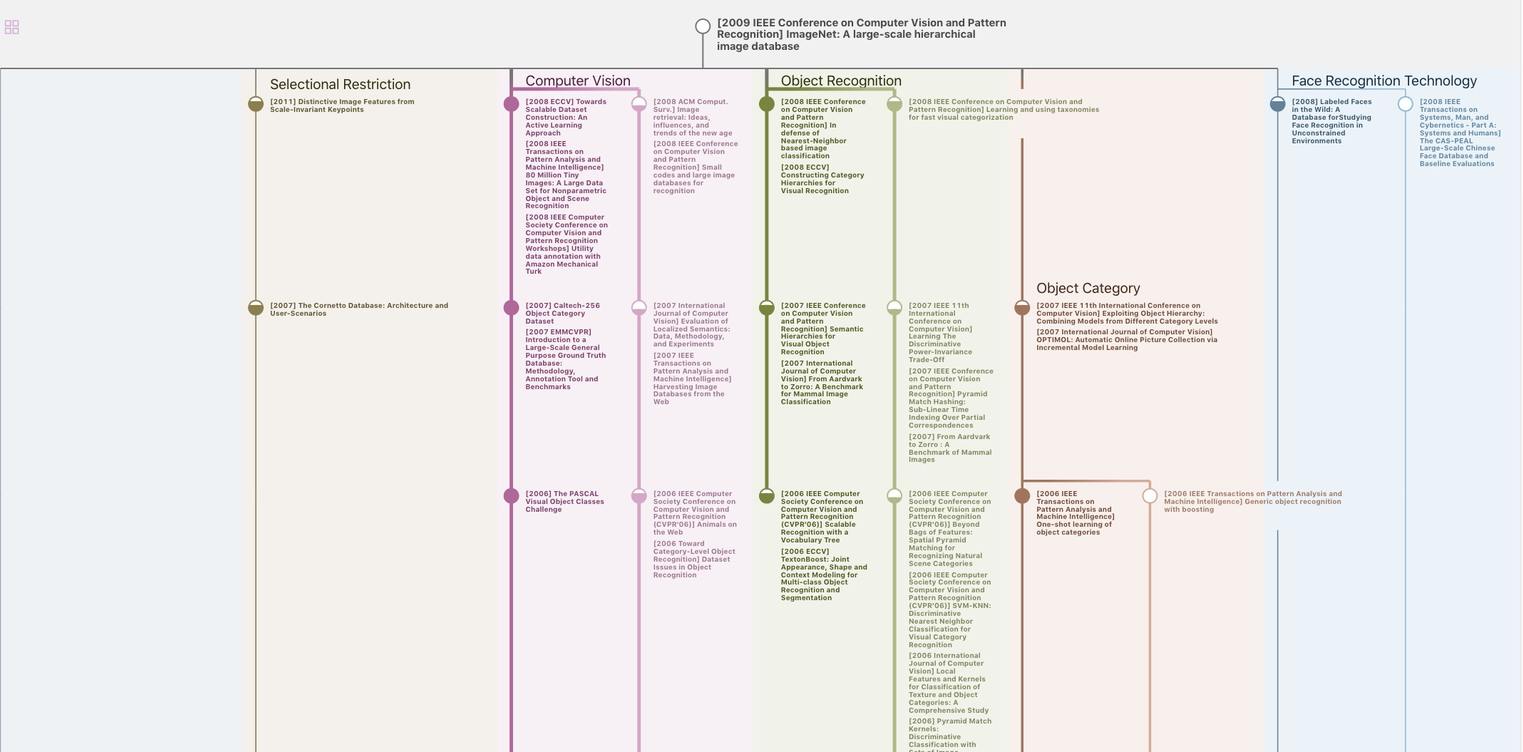
生成溯源树,研究论文发展脉络
Chat Paper
正在生成论文摘要