An Overview of Short-Term Load Forecasting for Electricity Systems Operational Planning: Machine Learning Methods and the Brazilian Experience
ENERGIES(2023)
摘要
The advent of smart grid technologies has facilitated the integration of new and intermittent renewable forms of electricity generation in power systems. Advancements are driving transformations in the context of energy planning and operations in many countries around the world, particularly impacting short-term horizons. Therefore, one of the primary challenges in this environment is to accurately provide forecasting of the short-term load demand. This is a critical task for creating supply strategies, system reliability decisions, and price formation in electricity power markets. In this context, nonlinear models, such as Neural Networks and Support Vector Machines, have gained popularity over the years due to advancements in mathematical techniques as well as improved computational capacity. The academic literature highlights various approaches to improve the accuracy of these machine learning models, including data segmentation by similar patterns, input variable selection, forecasting from hierarchical data, and net load forecasts. In Brazil, the national independent system operator improved the operation planning in the short term through the DESSEM model, which uses short-term load forecast models for planning the day-ahead operation of the system. Consequently, this study provides a comprehensive review of various methods used for short-term load forecasting, with a particular focus on those based on machine learning strategies, and discusses the Brazilian Experience.
更多查看译文
关键词
short-term load forecasting,day-ahead operational planning,time series forecasting,machine learning methods,electricity power systems
AI 理解论文
溯源树
样例
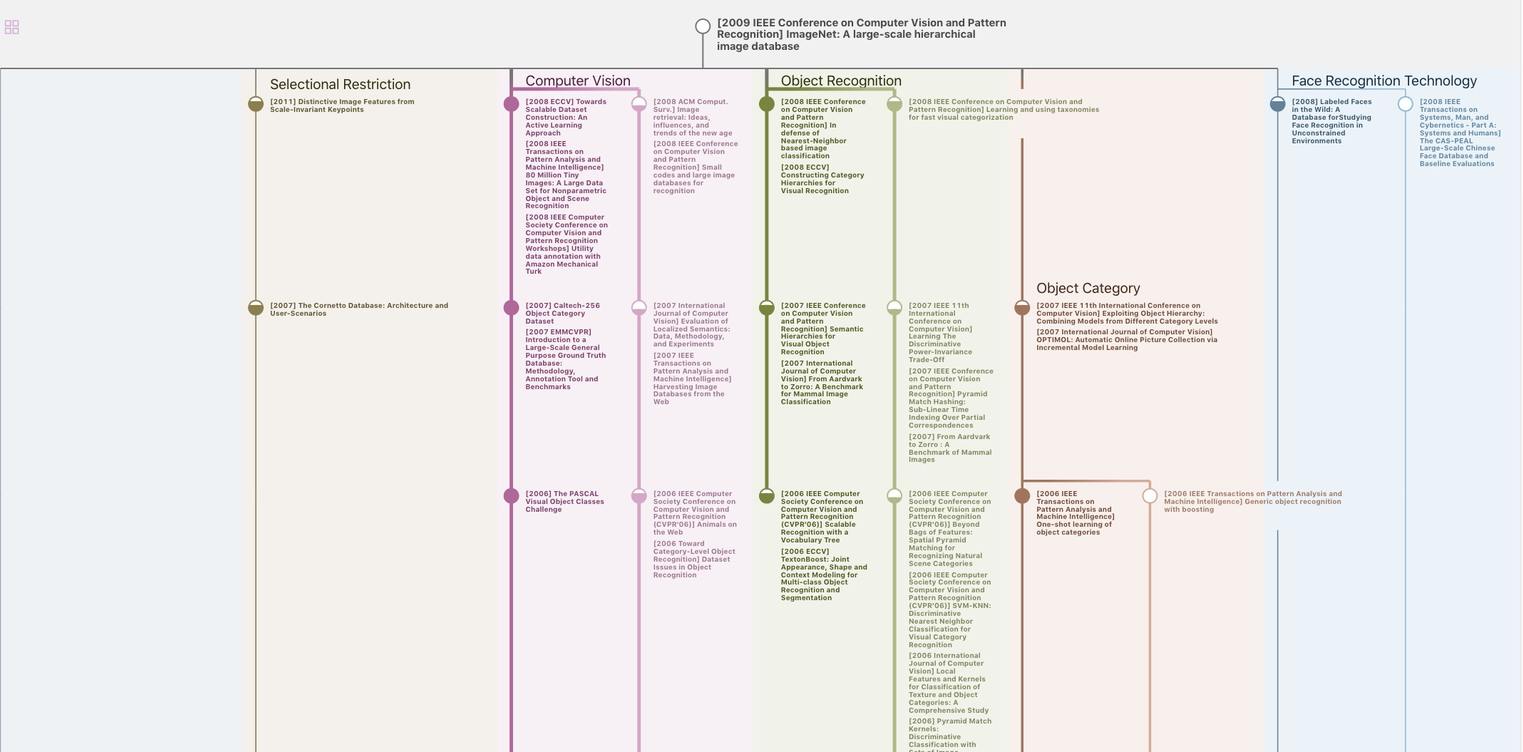
生成溯源树,研究论文发展脉络
Chat Paper
正在生成论文摘要