Prototype calibration for long tailed recognition
2023 IEEE INTERNATIONAL CONFERENCE ON MULTIMEDIA AND EXPO, ICME(2023)
摘要
In the real world, data distribution usually presents imbalanced characteristics, such as long-tailed distribution, which is generally divided into the head and tail classes. For tail classes, image features cannot be represented well due to insufficient training samples. It is a vital task to learn discriminative image representation on imbalanced data distribution. In our work, through exploring prototype information, we propose a prototype-based contrastive learning(PCL) loss and prototype-based feature augmentation(PFA) module to improve the accuracy of the classifier on the imbalanced dataset. Specifically, we utilize the classifier parameters to generate learnable embeddings, which can be regarded as the class centers after using metric learning. The PFA module generates the image features of each tail class with the help of head class information. We validate our approach on common long-tailed benchmarks. Our results indicate that the PCL and PFA make the classification model achieve significant performance boosts on these benchmarks.
更多查看译文
关键词
Long tailed recognition, Few-shot learning, Prototype Network, Contrastive Learning
AI 理解论文
溯源树
样例
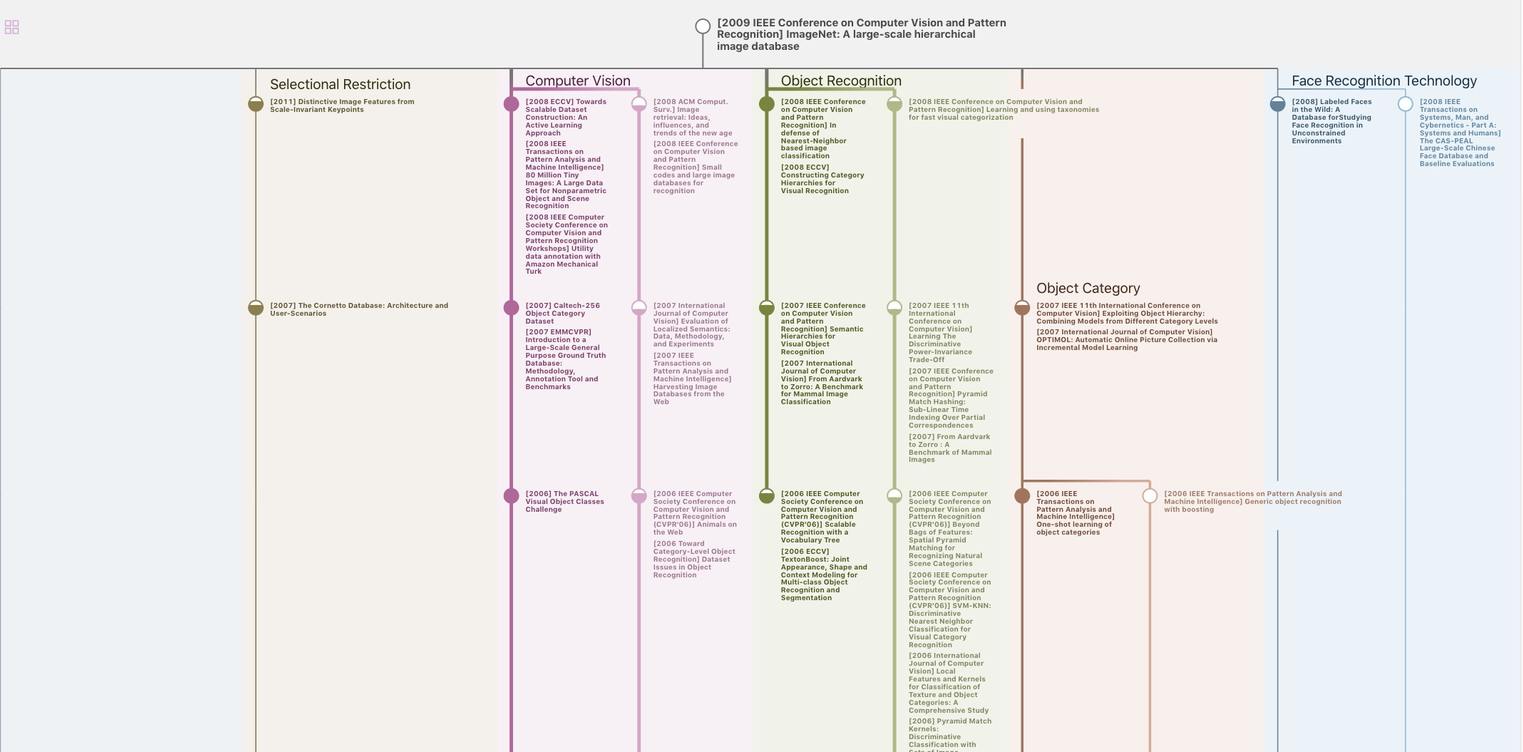
生成溯源树,研究论文发展脉络
Chat Paper
正在生成论文摘要