Detection of public speaking anxiety: a new dataset and algorithm
2023 IEEE INTERNATIONAL CONFERENCE ON MULTIMEDIA AND EXPO, ICME(2023)
Abstract
Precise public speaking anxiety detection (PSAD) in real-classroom teaching is a stepping stone for accurate evaluation of speaking competence and the subsequent interventions for reducing anxiety. But there are two major challenges. First, the predominant approach mainly focuses on the cognitive and physiological information, while the behavioral information has not been fully utilized due to the lack of a suitable multimodal dataset. This research constructs a specified dataset called Speaking Anxiety in Class (SAC) based on videos in class to fill this gap. Unlike the existing datasets, SAC contains a fine-grained annotation unit, large data volume, and multiple modalities. Second, behavioral PSAD only relys on the non-verbal clues in speakers' deliveries, not including the specific content, which makes the migration of the methods in other multimodal tasks not applicable. We propose a baseline model called Linguistic-Acoustic Decoupling (LAD) to explicitly separate the content and the non-verbal clues from different modalities of deliveries and only fuse the non-verbal clues for prediction. Our experiments demonstrate the effectiveness of LAD model. We make the SAC dataset and LAD model publicly available at https://github.com/FFFFFaraway/SAC-LAD
MoreTranslated text
Key words
Dataset, Public Speaking Anxiety, MultiModal Learning
AI Read Science
Must-Reading Tree
Example
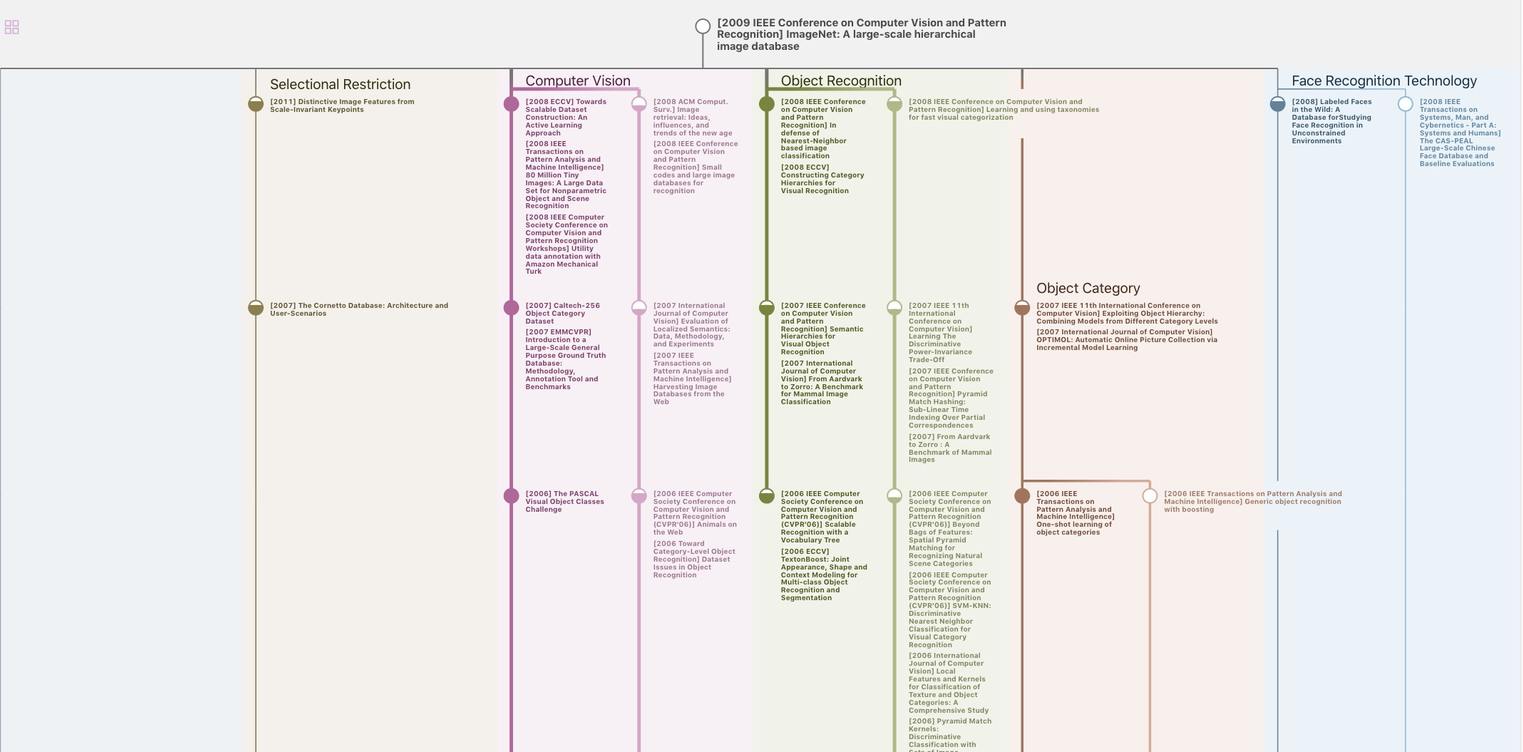
Generate MRT to find the research sequence of this paper
Chat Paper
Summary is being generated by the instructions you defined