Decentralized federated learning via mutual knowledge distillation
2023 IEEE INTERNATIONAL CONFERENCE ON MULTIMEDIA AND EXPO, ICME(2023)
摘要
Federated learning (FL), an emerging decentralized machine learning paradigm, supports the implementation of common modeling without compromising data privacy. In practical applications, FL participants heterogeneity poses a significant challenge for FL. Firstly, clients sometimes need to design custom models for various scenarios and tasks. Secondly, client drift leads to slow convergence of the global model. Recently, knowledge distillation has emerged to address this problem by using knowledge from heterogeneous clients to improve the model's performance. However, this approach requires the construction of a proxy dataset. And FL is usually performed with the assistance of a center, which can easily lead to trust issues and communication bottlenecks. To address these issues, this paper proposes a knowledge distillation-based FL scheme called FedDCM. Specifically, in this work, each participant maintains two models, a private model and a public model. The two models are mutual distillations, so there is no need to build proxy datasets to train teacher models. The approach allows for model heterogeneity, and each participant can have a private model of any architecture. The direct and efficient exchange of information between participants through the public model is more conducive to improving the participants' private models than a centralized server. Experimental results demonstrate the effectiveness of FedDCM, which offers better performance compared to s the most advanced methods.
更多查看译文
关键词
Federated learning,mutual knowledge distillation,decentralized
AI 理解论文
溯源树
样例
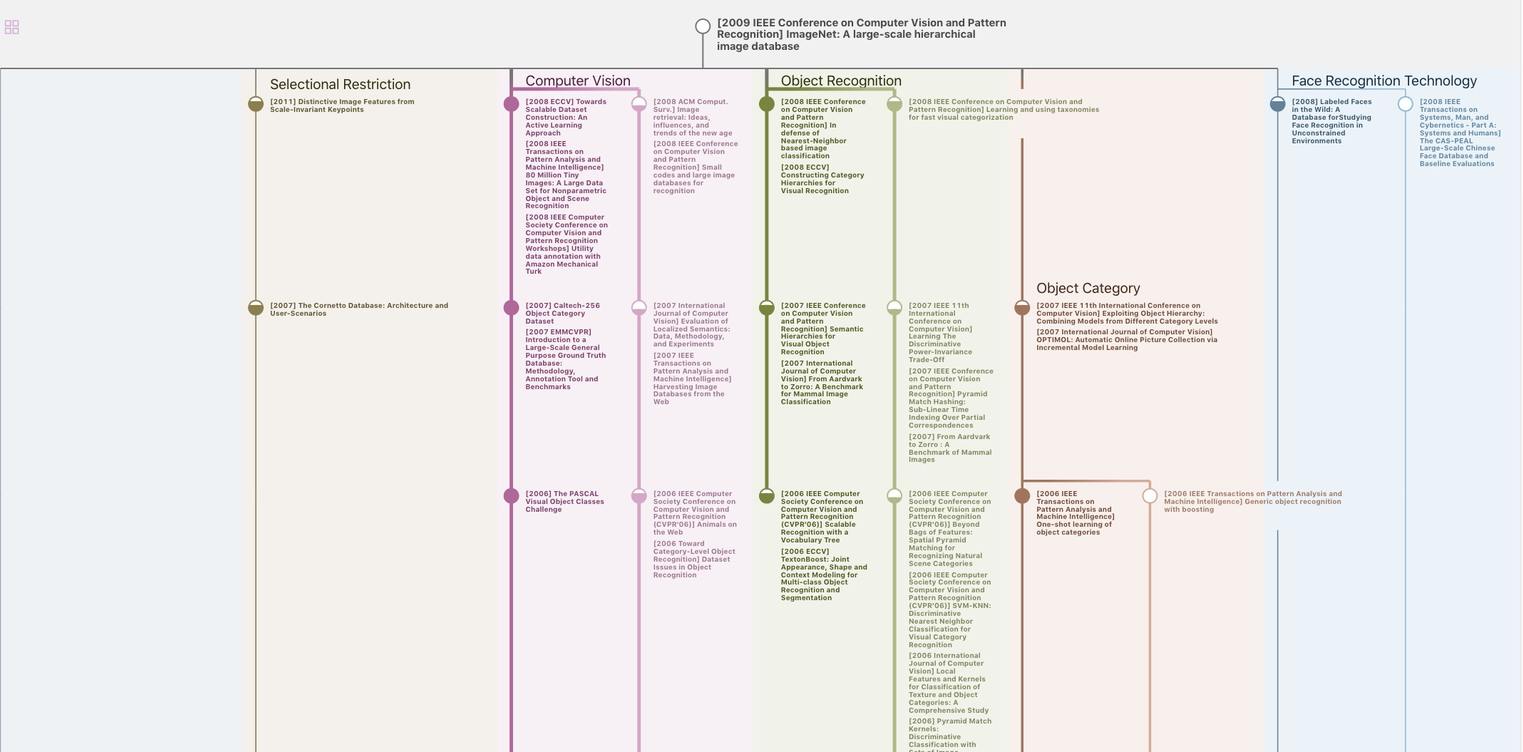
生成溯源树,研究论文发展脉络
Chat Paper
正在生成论文摘要