Transferable Waveform-level Adversarial Attack against Speech Anti-spoofing Models
2023 IEEE INTERNATIONAL CONFERENCE ON MULTIMEDIA AND EXPO, ICME(2023)
摘要
Speech anti-spoofing models protect media from malicious fake speech but are vulnerable to adversarial attacks. Studies of adversarial attacks are conducive to developing robust speech anti-spoofing systems. Existing transfer-based attack methods mainly craft adversarial speech examples at the handcrafted-feature level, which have limited attack ability against the real-world anti-spoofing systems, as these systems only have raw waveform input interfaces. In this work, we propose a waveform-level input data transformation, called the temporal smoothing method, to generate more transferable adversarial speech examples. In the optimization iterations of the adversarial perturbation, we randomly smooth input waveforms to prevent the adversarial examples from overfitting white-box surrogate models. The proposed transformation can be combined with any iterative gradient-based attack method. Extensive experiments demonstrate that our method significantly enhances the transferability of waveform-level adversarial speech examples.
更多查看译文
关键词
adversarial attack, raw waveform, speech anti-spoofing, transferability
AI 理解论文
溯源树
样例
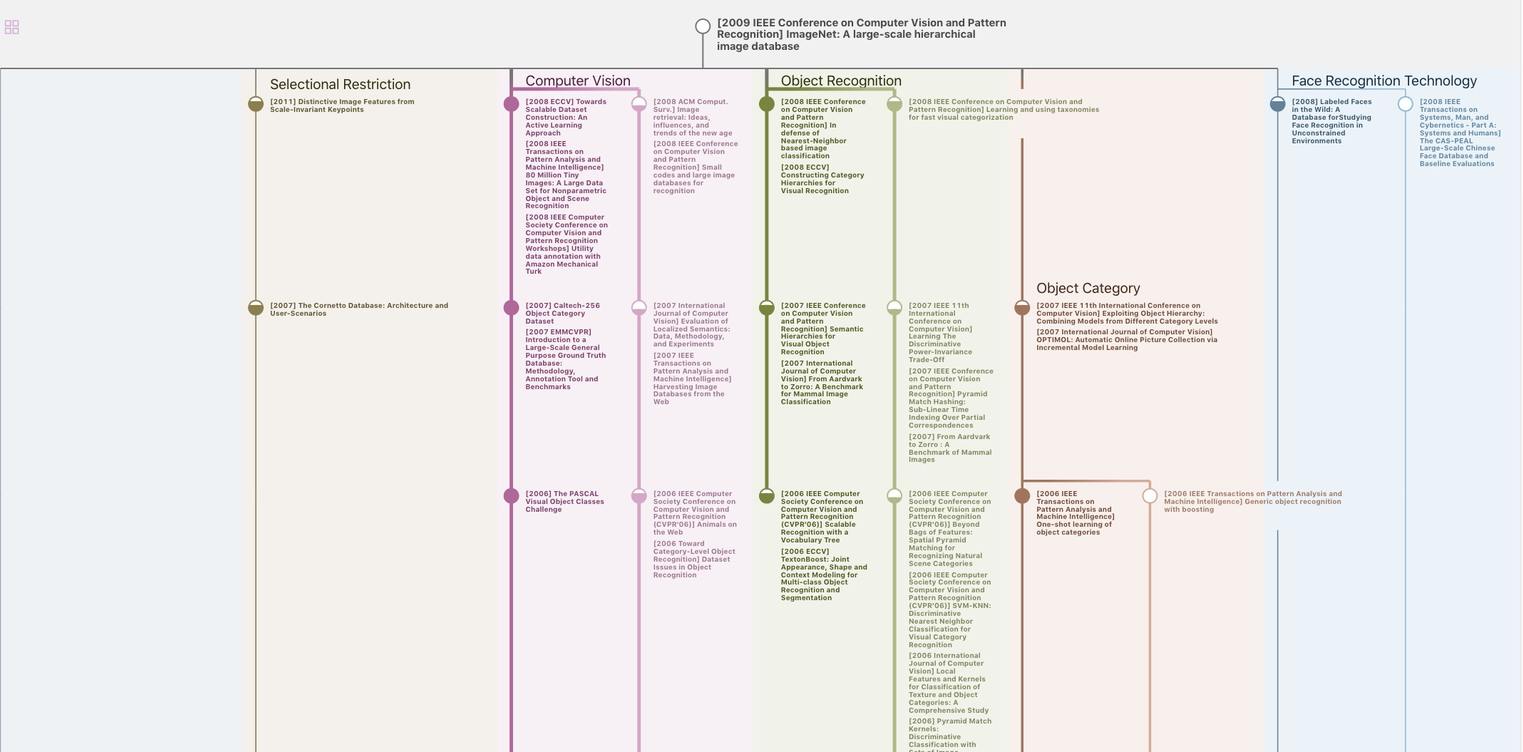
生成溯源树,研究论文发展脉络
Chat Paper
正在生成论文摘要