Privacy-enhanced zero-shot learning via data-free knowledge transfer
2023 IEEE INTERNATIONAL CONFERENCE ON MULTIMEDIA AND EXPO, ICME(2023)
Abstract
Considering the increasing concerns about data copyright and sensitivity issues, we present a novel Privacy-Enhanced Zero-Shot Learning (PE-ZSL) paradigm. The key innovation is to involve a teacher model as the data safeguard to guide the PE-ZSL model training without data sharing. The PE-ZSL model consists of a generator and student network, which can achieve data-free knowledge transfer while maintaining the performance of teacher model. We investigate 'black-' and 'white-box' scenarios in PE-ZSL task as different levels of framework privacy. Besides, we provide the discussion of teacher model in both omniscient and quasi-omniscient settings according to the knowledge space. Despite simple implementations and data-missing disadvantages, our PE-ZSL framework can retain state-of-the-art ZSL and GZSL performance under the 'white-box' scenario. Extensive qualitative and quantitative analysis also demonstrates promising results when deploying the model under 'black-box' scenario.
MoreTranslated text
Key words
Zero-Shot Learning,Privacy Protection,Data-Free Knowledge Transfer
AI Read Science
Must-Reading Tree
Example
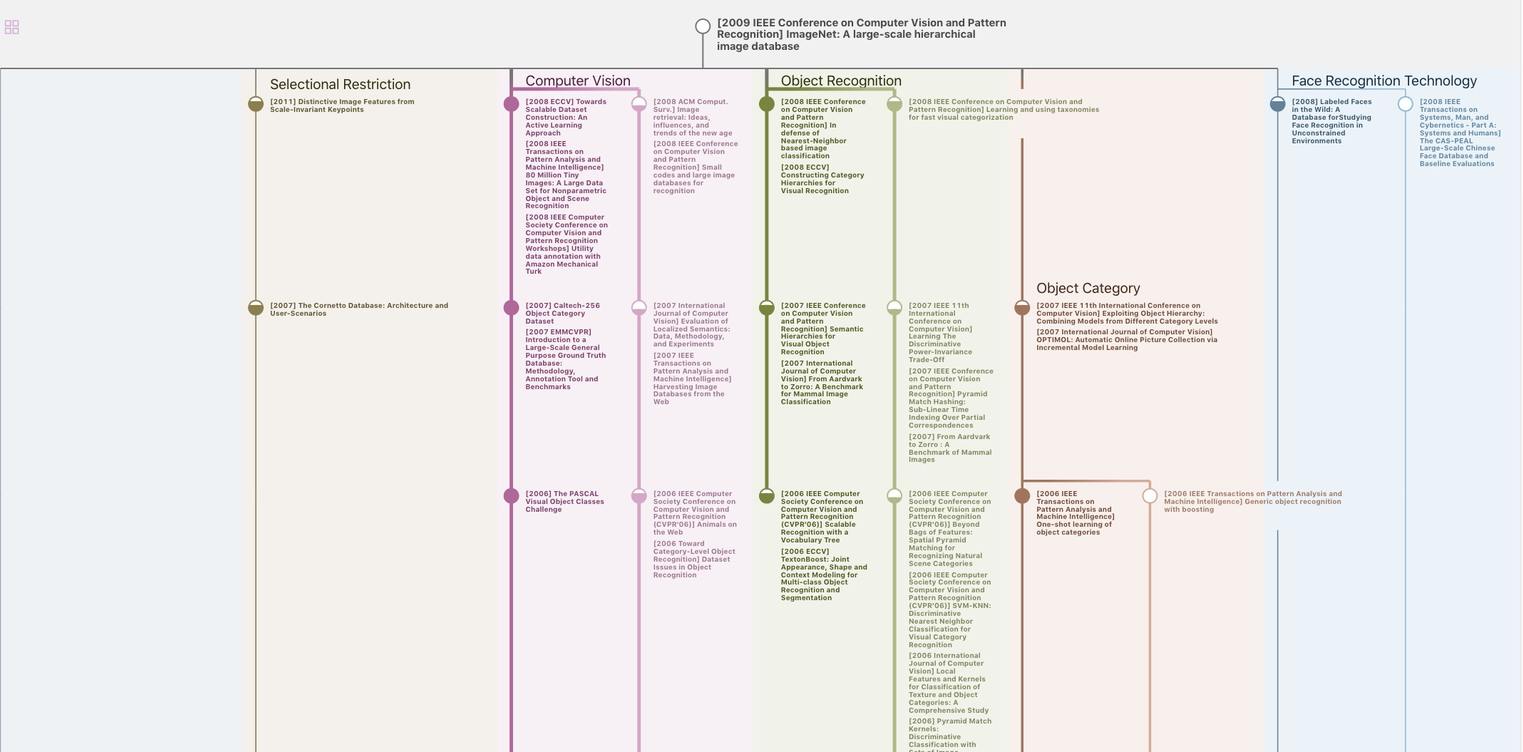
Generate MRT to find the research sequence of this paper
Chat Paper
Summary is being generated by the instructions you defined