Dual Episodic Sampling and Momentum Consistency Regularization for Unsupervised Few-shot Learning
2023 IEEE INTERNATIONAL CONFERENCE ON MULTIMEDIA AND EXPO, ICME(2023)
摘要
Unsupervised Few-shot Learning (UFSL) is a practical approach to adapting knowledge learned from unlabeled data of base classes to novel classes with limited labeled data. Nevertheless, most existing UFSL methods may not learn generalizable features in latter training epochs due to the simplicity of meta-learning tasks constructed by data augmentation. To address this issue, we propose two novel components, namely Dual Episodic Sampling (DES) and Momentum Consistency Regularization (MCR) for UFSL. In the DES, two types of sampling strategies are used to construct harder training tasks with multiple augmentations to generate each pseudo-class of increased diversity. The MCR constrains the consistency of the backbone encoder with its momentum counterpart to learn better generalized features for novel classes. Experimental results on four datasets verify the superiority of our method for unsupervised few-shot image classification.
更多查看译文
关键词
Unsupervised Few-shot Learning,Dual Episodic Sampling,Momentum Consistency Regularization
AI 理解论文
溯源树
样例
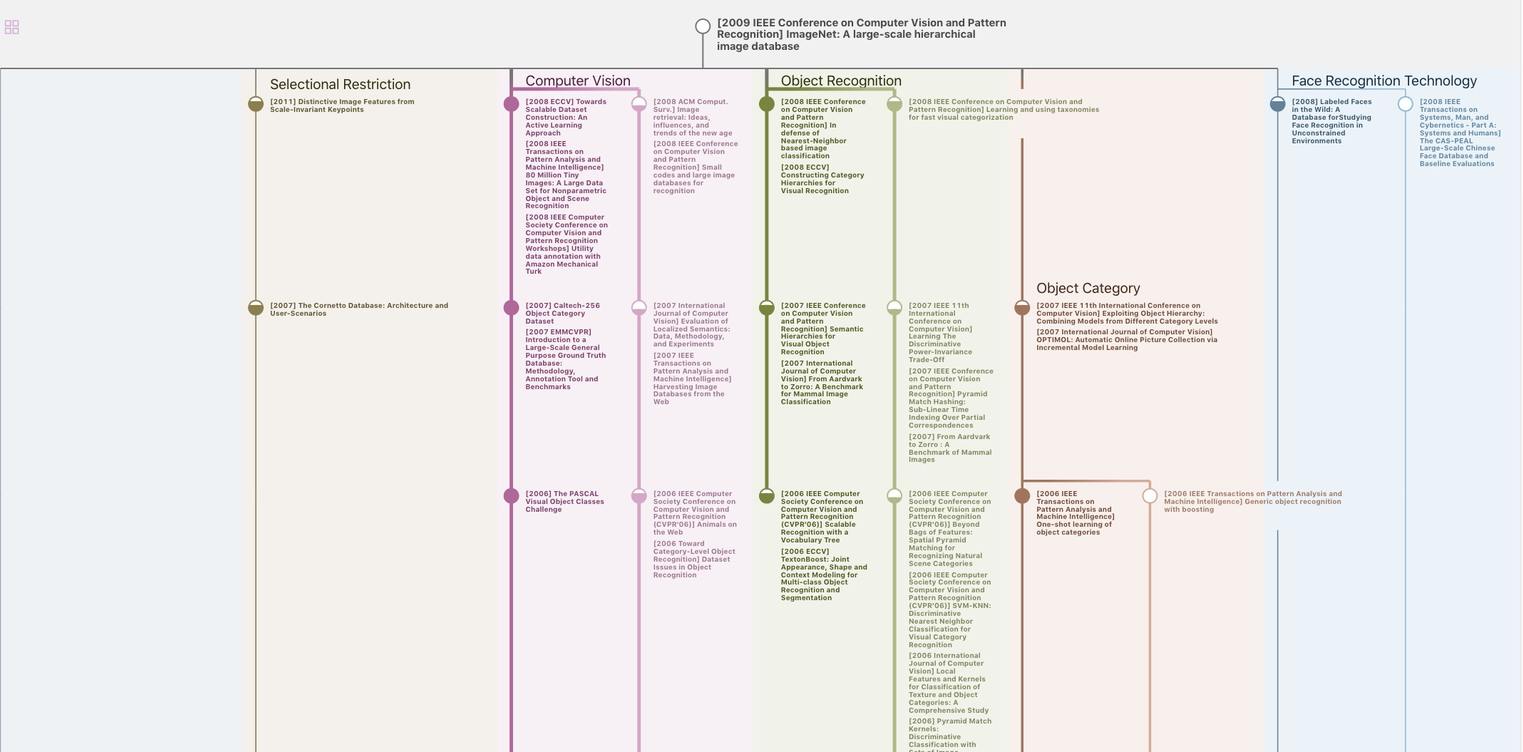
生成溯源树,研究论文发展脉络
Chat Paper
正在生成论文摘要