Eanet: towards lightweight human pose estimation with effective aggregation network
2023 IEEE INTERNATIONAL CONFERENCE ON MULTIMEDIA AND EXPO, ICME(2023)
Abstract
Existing solutions to lightweight human pose estimation typically adopt a depthwise separable strategy, i.e., a normal 2D convolution is factorized into channel aggregation and spatial aggregation. However, this strategy cannot well capture multi-scale Effective Receptive Field (ERF), which is essential to dense prediction tasks like human pose estimation. To address this issue, we propose a novel lightweight network for human pose estimation, namely effective aggregation net (EANet). In EANet, we introduce two lightweight computational units: effective channel aggregating (ECA) and effective spatial aggregating (ESA), which are respectively responsible for channel-wise feature aggregation and pixel-wise feature aggregation. Unlike typical channel-wise aggregation using pointwise (1x1) convolution, the ECA aggregates few feature points that are estimated as effective ones. Moreover, the ESA is designed with re-parameterizing techniques, and it aggregates effective spatial feature points with multi-scale shared convolutions. Comprehensive experiments are conducted on three challenging datasets, i.e., COCO, CrowdPose, Wholebody-COCO. Our EANet demonstrates superior results on human pose estimation over previous lightweight methods, reaching a new state-of-the-art performance with a good trade-off. Our code and models are publicly available(1).
MoreTranslated text
Key words
lightweight, human pose estimation
AI Read Science
Must-Reading Tree
Example
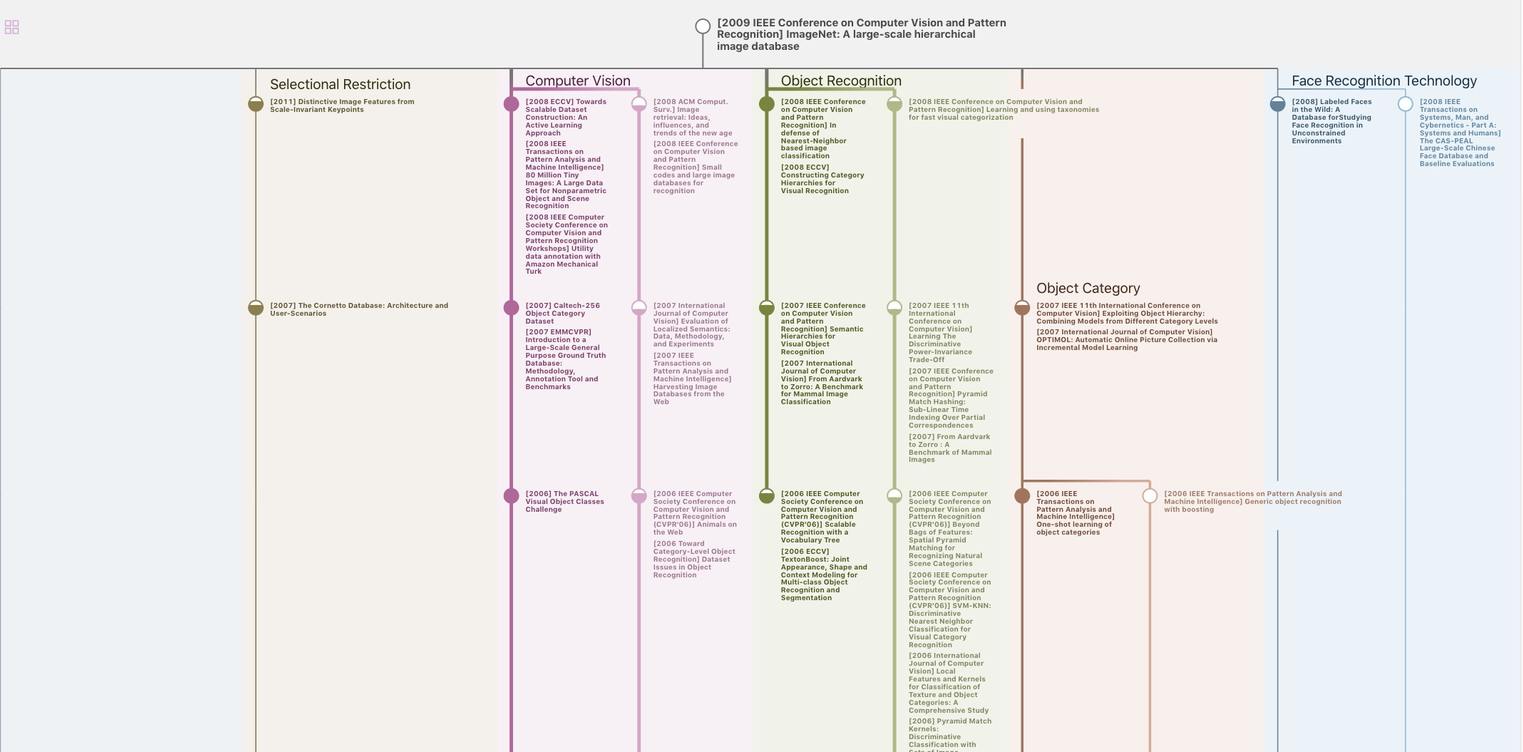
Generate MRT to find the research sequence of this paper
Chat Paper
Summary is being generated by the instructions you defined