Sum-of-Squares Relaxations in Robust DC Optimization and Feature Selection
JOURNAL OF OPTIMIZATION THEORY AND APPLICATIONS(2024)
摘要
This paper presents sum-of-squares (SOS) relaxation results to a difference-of-convex-max (DC-max) optimization involving SOS-convex polynomials in the face of constraint data uncertainty and their applications to robust feature selection. The main novelty of the present work in relation to the recent research in robust convex and DC optimization is the derivation of a new form of minimally exact SOS relaxations for robust DC-max problems. This leads to the identification of broad classes of robust DC-max problems with finitely exact SOS relaxations that are numerically tractable. They allow one to find the optimal values of these classes of DC-max problems by solving a known finite number of semi-definite programs (SDPs) for certain concrete cases of commonly used uncertainty sets in robust optimization. In particular, we derive relaxation results for a class of robust fractional programs. Also, we provide a finitely exact SDP relaxation for a DC approximation problem of an NP-hard robust feature selection model which gives computable upper bounds for the global optimal value.
更多查看译文
关键词
DC optimization,SOS-convex polynomials,Robust optimization,Semi-definite programs,Robust feature selection
AI 理解论文
溯源树
样例
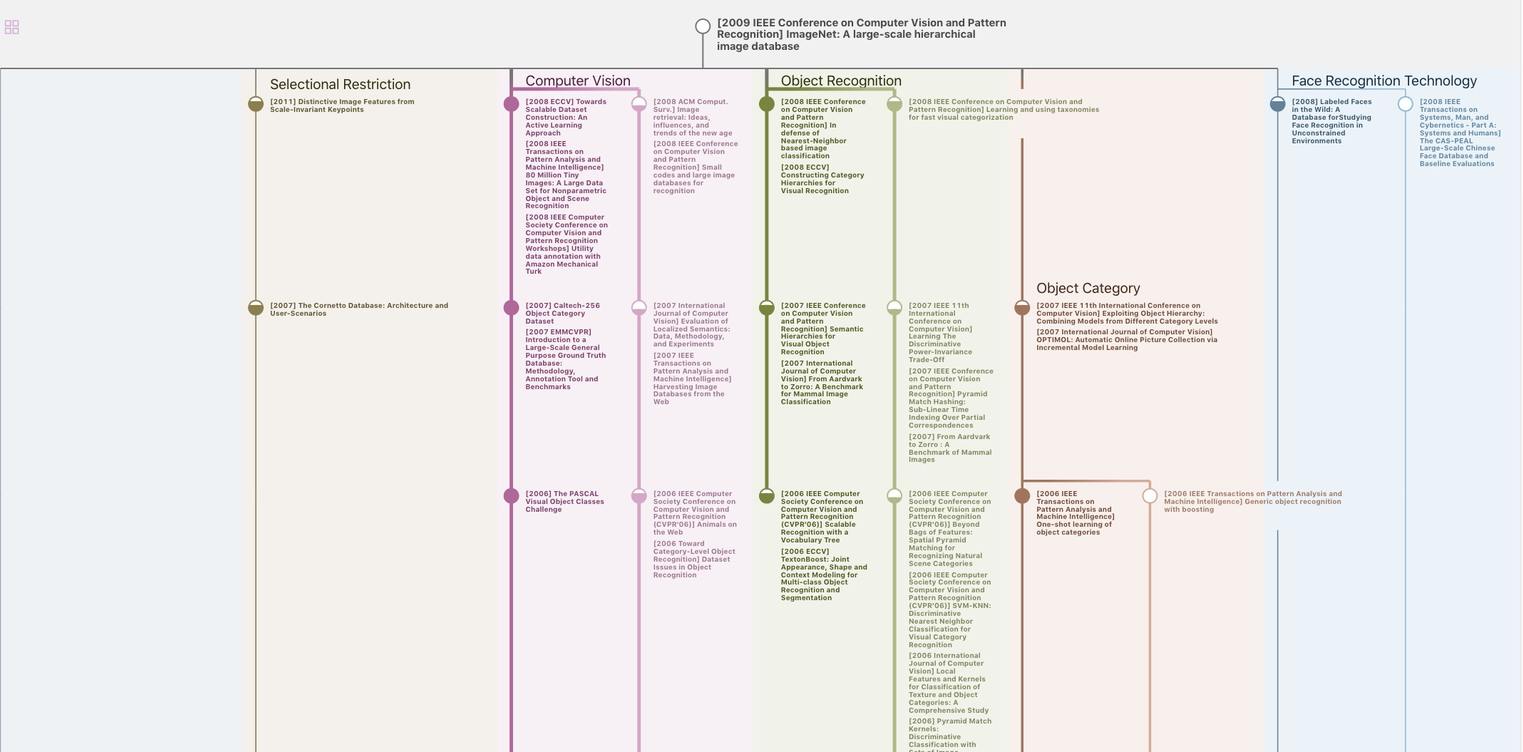
生成溯源树,研究论文发展脉络
Chat Paper
正在生成论文摘要