Inter-Intra Camera Identity Learning for Person Re-Identification with Training in Single Camera
2023 IEEE INTERNATIONAL CONFERENCE ON MULTIMEDIA AND EXPO, ICME(2023)
摘要
Traditional person re-identification (re-ID) methods generally rely on inter-camera person images to smooth the domain disparities between cameras. However, collecting and annotating a large number of inter-camera identities is extremely difficult and time-consuming, and this makes it hard to deploy person re-ID systems in new locations. To tackle this challenge, this paper studies the single-camera-training (SCT) setting where every person in the training set only appears in one camera. In this work, we design a novel inter-intra camera identity learning (I2CIL) framework to effectively address the SCT person re-ID. Specifically, (i) we design a Dual-Branch Identity Learning (DBIL) network consisting of inter-camera and intra-camera learning branches to learn person ID discriminative information. The former learns camera-irrelevant feature representations by constraining the distance of inter-camera negative sample pairs closer than the distance of intra-camera negative sample pairs. The latter focuses on pulling the distance of intra-camera positive sample pairs closer and pushing the distance of intra-camera negative sample pairs further, partially alleviating weak ID discrimination caused by the lack of inter-camera annotations. (ii) We design a Mixed-Sampling Joint Learning (MSJL) strategy, which is capable to capture inter- and intra-camera samples and independently accomplish the inter- and intra-camera learning tasks at the same time, avoiding the mutual interference between the two tasks. Extensive experiments on two public SCT datasets prove the superiority of the proposed approach.
更多查看译文
关键词
Person re-identification, Single Camera Training, Deep Learning
AI 理解论文
溯源树
样例
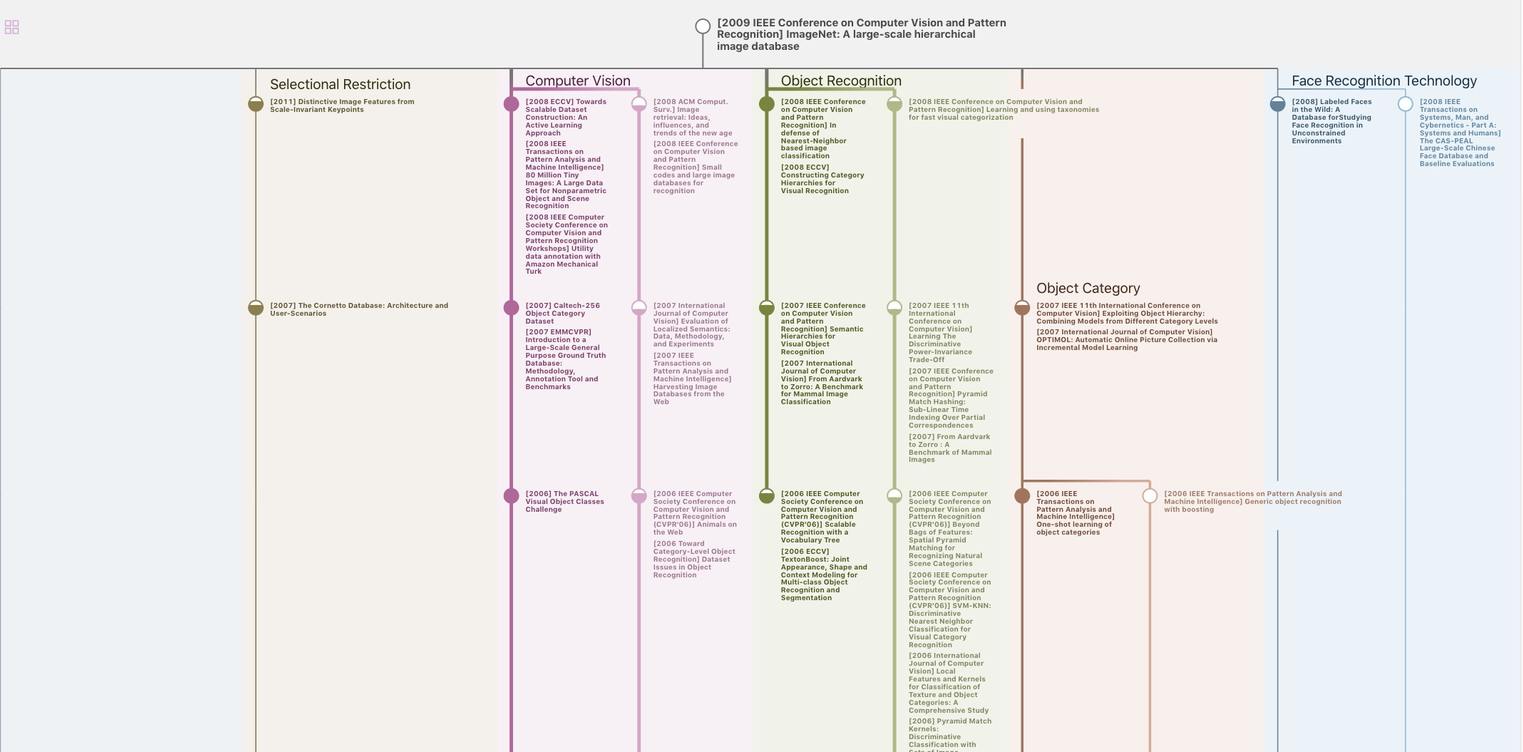
生成溯源树,研究论文发展脉络
Chat Paper
正在生成论文摘要