CTSSeg: Consistent Teacher-Student model for magnetic resonance image Segmentation
2023 IEEE INTERNATIONAL CONFERENCE ON MULTIMEDIA AND EXPO, ICME(2023)
摘要
Segmentation of magnetic resonance images is an essential way of measuring the volume of tissues and lesions, which can improve the efficiency of diagnosis. The mainstream image segmentation methods are based on deep learning, which requires a large amount of labeled data. However, labeling magnetic resonance images is expensive and time-consuming. Therefore, we propose a consistent teacher-student model for magnetic resonance image segmentation, which is abbreviated as CTSSeg. Specifically, the CTSSeg includes a student network and a teacher network, where the student network learns supervised from labeled data, while the teacher network utilizes unlabeled data to improve the student network via contrastive learning and pseudo-label learning. We evaluate the proposed CTSSeg on the Atrial Segmentation Challenge dataset and a local clinical dataset. The experimental results show that our method can make full use of both labeled and unlabeled data and yield state-of-the-art performance.
更多查看译文
关键词
MR image segmentation, Semi-supervised learning
AI 理解论文
溯源树
样例
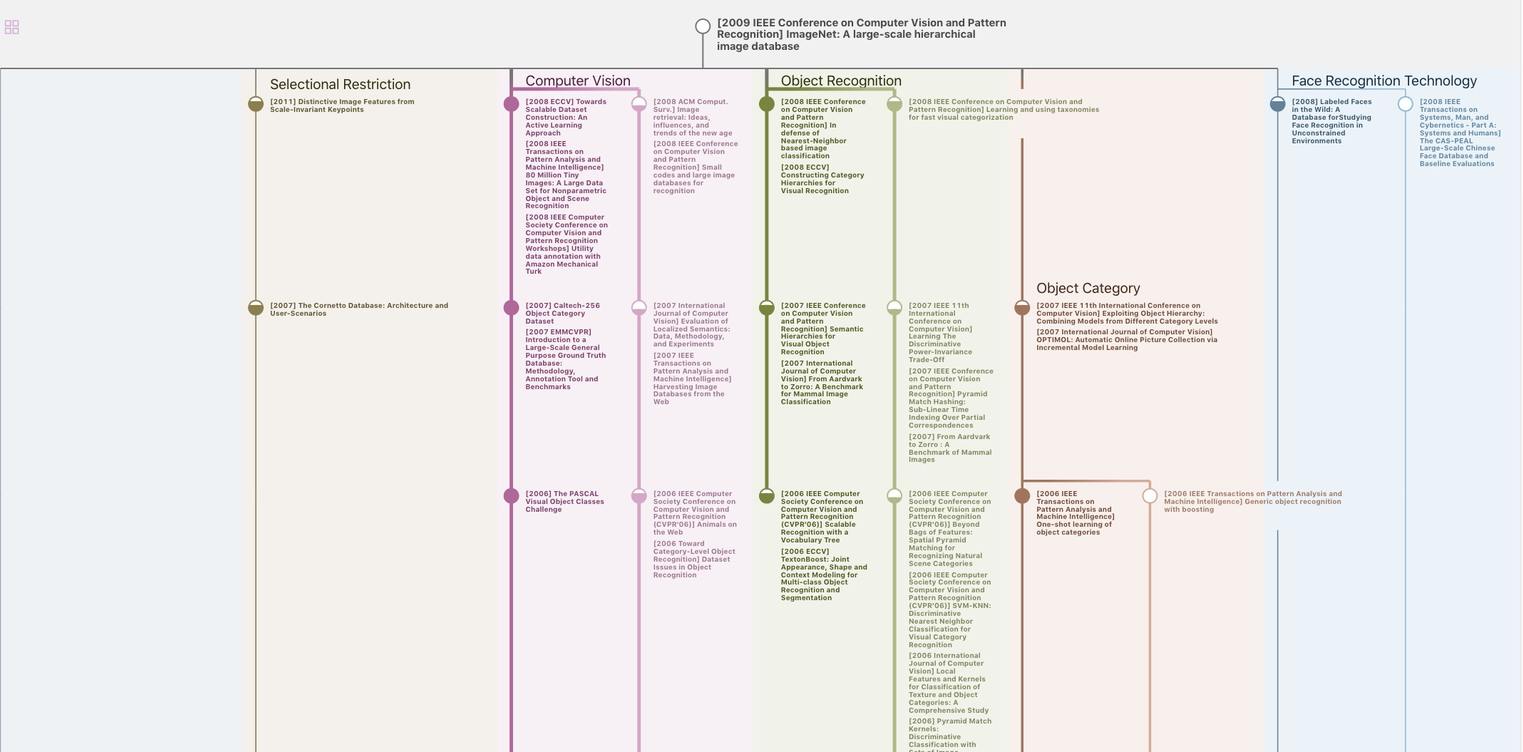
生成溯源树,研究论文发展脉络
Chat Paper
正在生成论文摘要