Feature Bias Correction: A Feature Augmentation Method for Long-tailed Recognition
2023 IEEE INTERNATIONAL CONFERENCE ON MULTIMEDIA AND EXPO, ICME(2023)
Key words
long-tailed recognition,feature saliency rebalancing,distinction of similar features
AI Read Science
Must-Reading Tree
Example
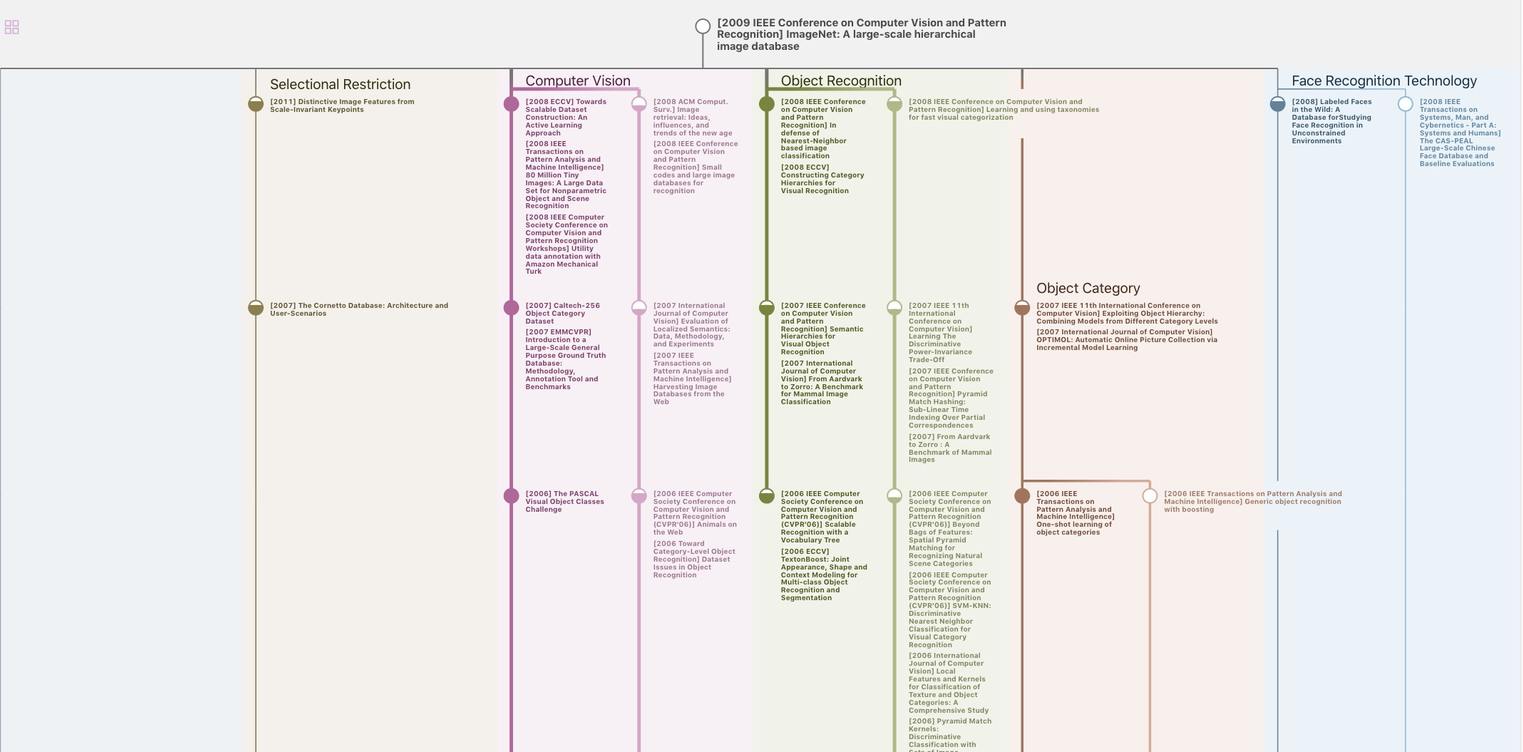
Generate MRT to find the research sequence of this paper
Chat Paper
Summary is being generated by the instructions you defined