Multi-physical predictions in electro-osmotic micromixer by auto-encoder physics-informed neural networks
PHYSICS OF FLUIDS(2023)
摘要
Electro-osmotic micromixers (EMMs) are used for manipulating microfluidics because of the advantages on electro-osmosis mechanisms. The intricate interdependence between various fields in the EMM model presents a challenge for traditional numerical methods. In this paper, the flow parameters and electric potential are predicted based on the solute concentration by utilizing the physics-informed neural networks (PINNs) method. The unknown spatiotemporal dependent fields are derived from a deep neural network trained by minimizing the loss function integrating data of scalar field and corresponding governing equations. Moreover, the auto-encoder structure is developed to improve the performance of PINNs in the EMM. The comparisons between the results of auto-encoder PINNs and previous PINNs show a reduction in relative errors for transverse and longitudinal velocities from 83.35% and 84.24% to 9.88% and 12.29%, respectively, in regions with large-gradient velocities. Furthermore, our results demonstrate that the proposed method is robust to noise in the scalar concentration.
更多查看译文
关键词
neural networks,multi-physical,electro-osmotic,auto-encoder,physics-informed
AI 理解论文
溯源树
样例
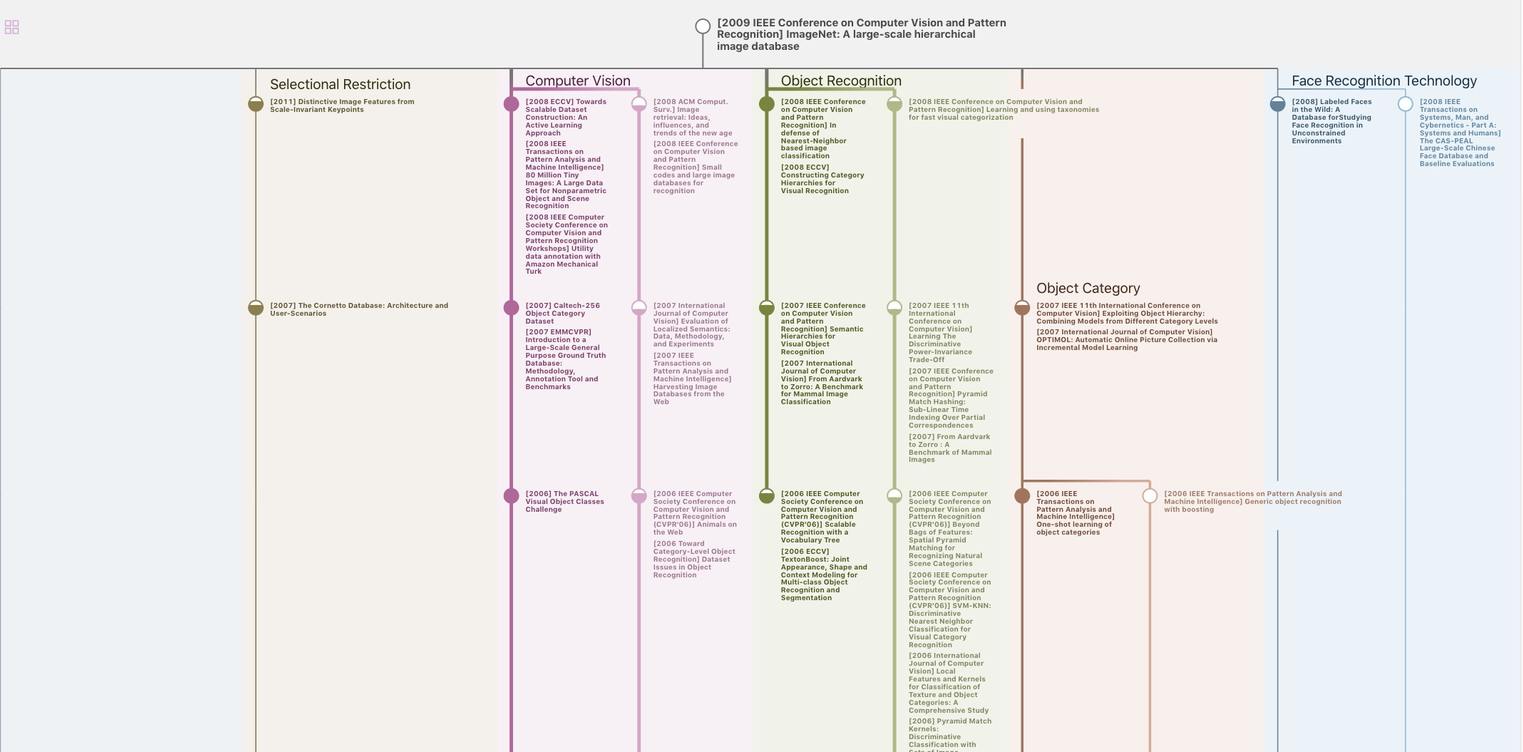
生成溯源树,研究论文发展脉络
Chat Paper
正在生成论文摘要