Dual teacher model for semi-supervised ABUS tumor segmentation
BIOMEDICAL SIGNAL PROCESSING AND CONTROL(2024)
Abstract
Automated Breast Ultrasound (ABUS) has emerged as a crucial modality for the detection of breast tumors in clinical settings. However, obtaining accurate pixel-level annotations of medical images for ABUS tumor seg-mentation is a labor-intensive and expensive process, which hinders the performance of deep learning methods. In this work, we propose a novel semi-supervised tumor segmentation model, namely the Dual-Teacher model, which effectively leverages a small set of labeled images and a large number of unlabeled images for improved segmentation. The Dual-Teacher model extends the original mean teacher (MT) model, MT model only utilizes commonly used consistency criteria, by incorporating an auxiliary teacher branch the Dual-Teacher model captures complementary information from the teacher model and guides the training of the student model. We further introduce a pseudo-label update strategy that combines self-training and consistency constraints to improve the accuracy of pseudo-labels. To optimize the training of the Dual-Teacher model, we design an adaptive loss function that considers the pseudo-labels generated by both teacher models. Our proposed method is evaluated on an ABUS dataset comprising 107 patients with 170 volumes, totaling 3736 2D labeled slices. Experimental results demonstrate that the Dual-Teacher model achieves a Dice similarity coefficient (DSC) of 74.6 %, precision of 75.3 % and recall of 73.9 %, indicating promising performance compared to existing methods.
MoreTranslated text
Key words
Deep learning,Mean teacher,Automated breast ultrasound (ABUS) image,Semi-supervised learning,Pseudo label
AI Read Science
Must-Reading Tree
Example
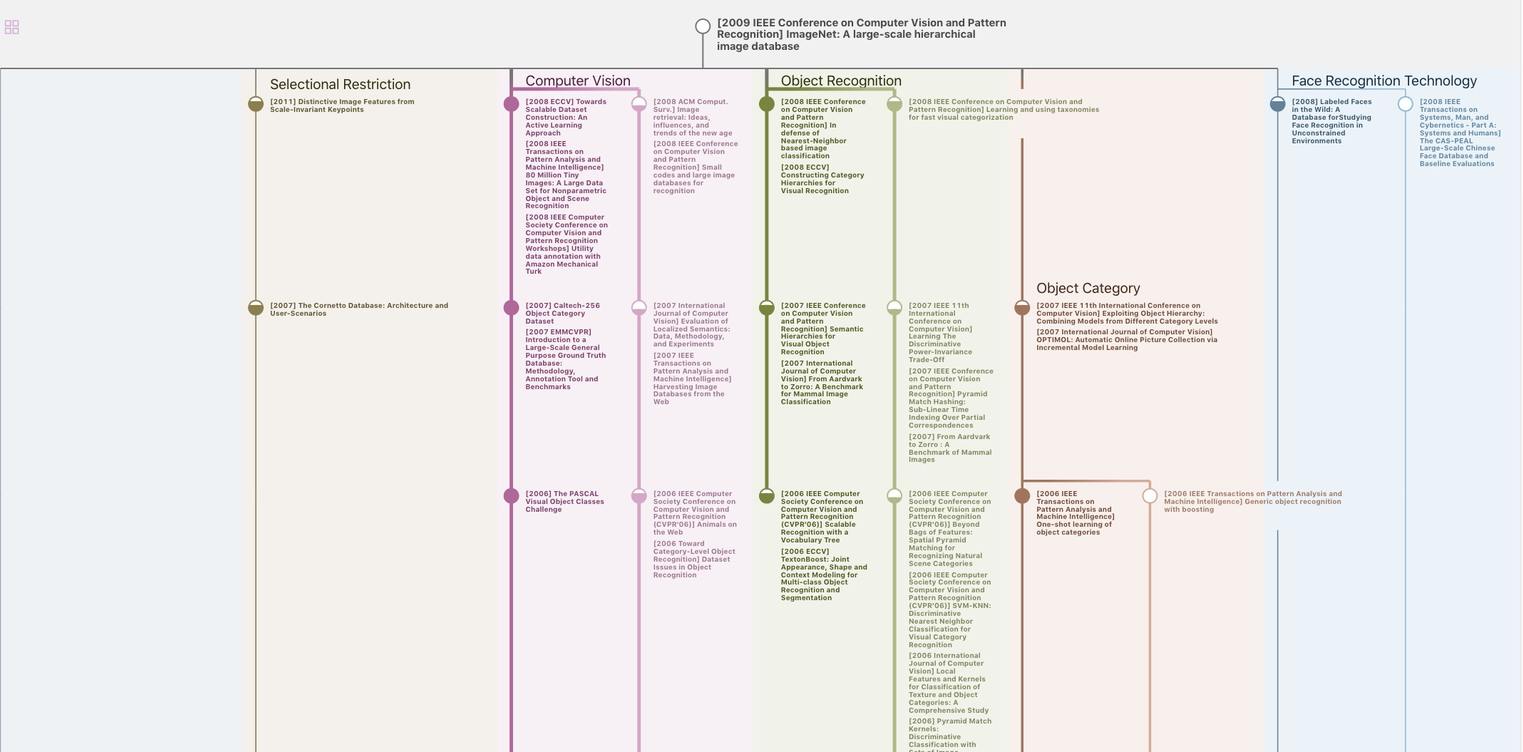
Generate MRT to find the research sequence of this paper
Chat Paper
Summary is being generated by the instructions you defined