PCA-based fast point feature histogram simplification algorithm for point clouds
ENGINEERING REPORTS(2023)
摘要
In order to realize efficient and lightweight digital inspection technology, we propose an improved method to simplification large volumes of scanned point cloud data of aircraft integral panels and facilitate subsequent processing. Fast Point Feature Histogram (FPFH) method is utilized to extract feature information and optimize the Principal Component Analysis (PCA) algorithm to calculate the contribution degree to transform into principal components, yielding PCA-based FPFH features. Next, based on PCA-based FPFH features, we classify the point cloud data into non-feature and feature point clouds and extract the feature point clouds through random downsampling to obtain simplified non-feature point clouds, extract the feature point clouds to retain their boundary integrity, and downsample the remaining feature point clouds by curvature to obtain the simplified feature point clouds. Finally, we combine the two to obtain the final simplified panel point cloud data within 30 s. To evaluate the simplification effect, we adopt a standardized information entropy-based point cloud simplification accuracy evaluation method based on the simplification rate. Our method achieves an information entropy of more than 0.95, indicating its effectiveness in simplification point cloud data for efficient and lightweight digital inspection technology. In order to realize efficient and lightweight digital inspection technology, we propose an PCA-based FPFH method to simplify large volumes of scanned point cloud data of aircraft integral panels. Our method achieves an information entropy of more than 0.95, indicating its effectiveness in streamlining point cloud data for efficient and lightweight digital inspection technology.image
更多查看译文
关键词
data dimensionality reduction, digital detection, point cloud, principal component analysis
AI 理解论文
溯源树
样例
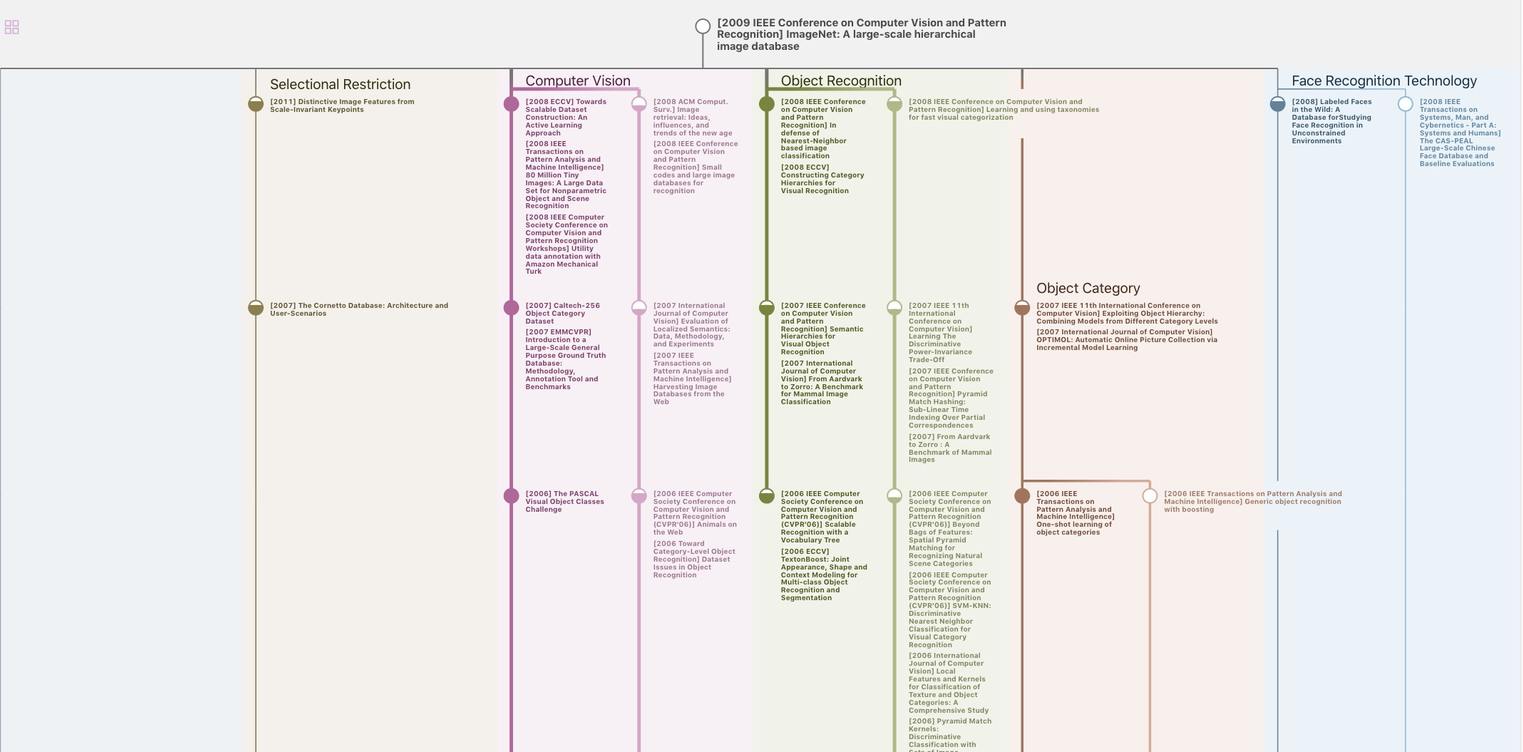
生成溯源树,研究论文发展脉络
Chat Paper
正在生成论文摘要