Rethinking Self-Supervision for Few-Shot Class-Incremental Learning
2023 IEEE INTERNATIONAL CONFERENCE ON MULTIMEDIA AND EXPO, ICME(2023)
摘要
Few-Shot Class-Incremental Learning (FSCIL) focuses on progressively absorbing new concepts given only limited training data. For tackling this challenge, several recent FSCIL works resort to pre-training models with Self-Supervised Learning (SSL) to obtain features that can generalize well to new classes. However, to avoid overfitting and catastrophic forgetting, previous works only leverage SSL in the base session and keep all or most parameters fixed in incremental sessions, resulting in inadequate adaptation to novel classes. Thus, in this paper, we explore the setting where more parameters can be updated for adapting to novel concepts, and discover that the model pre-trained with SSL leads to degraded performance even compared to that without SSL. It can be attributed to the severer forgetting of base class knowledge. To address this issue, we propose an imprinting-based distillation module for effectively regularizing the adaption process, and a mathematically provable routing strategy for further improved results. The effectiveness of our approach is verified on 3 popular FSCIL benchmarks by significantly outperforming previous methods.
更多查看译文
关键词
Few-shot learning, incremental learning, self-supervised learning
AI 理解论文
溯源树
样例
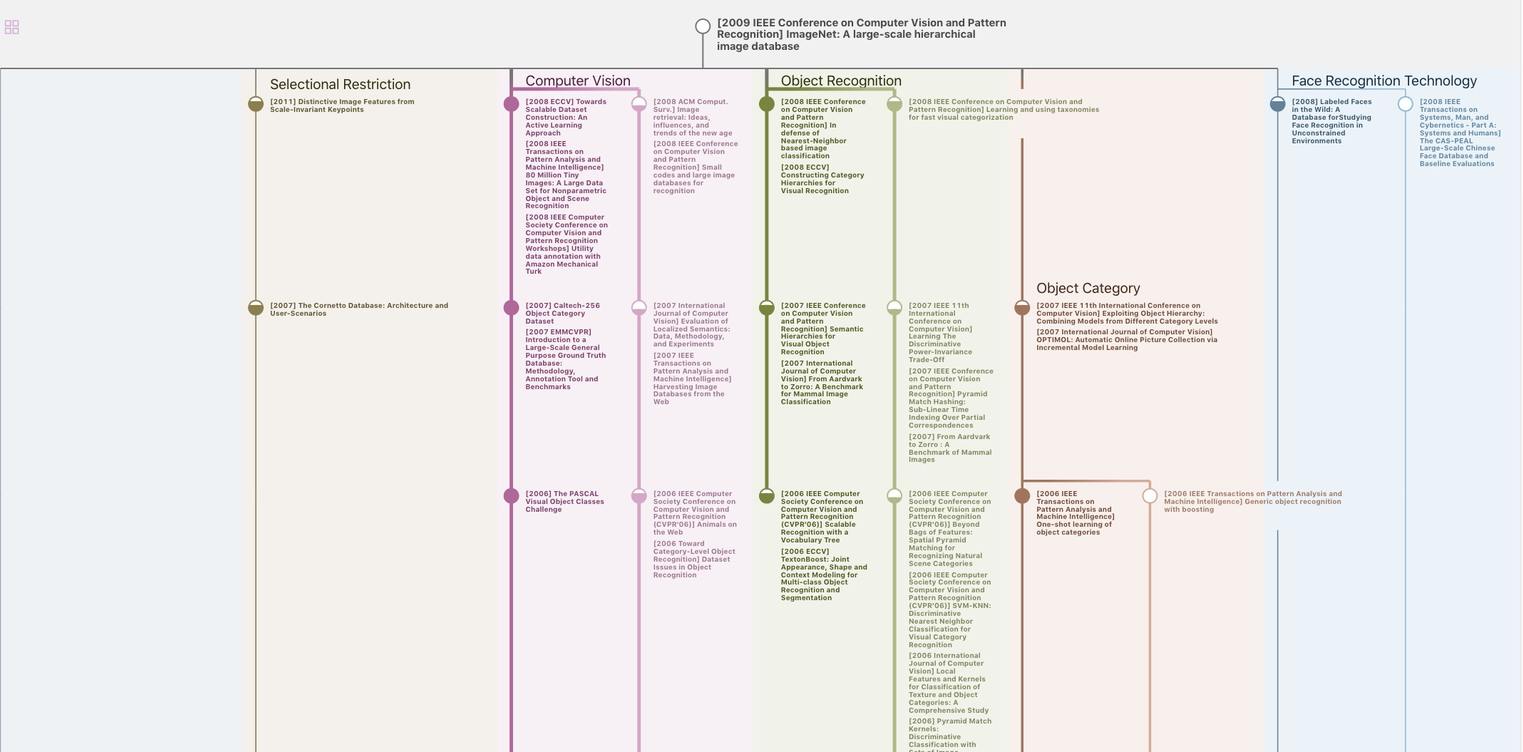
生成溯源树,研究论文发展脉络
Chat Paper
正在生成论文摘要