Rolling bearing fault diagnosis method based on ELMD hybrid feature extraction and wavelet neural network
JOURNAL OF VIBROENGINEERING(2023)
Abstract
Rolling bearings are the most important components in the transmission system of coal mining machinery, and their operating condition significantly impacts the entire mechanical and electrical equipment. Therefore, the fault diagnosis of rolling bearing can effectively ensure the operation reliability of equipment. Given the strong noise, coal impact, and other interference, the vibration signal of the rolling bearing cannot be effectively decomposed, and the fault identification efficiency is low. According to the method based on vibration analysis, this article proposes a rolling bearing fault diagnosis method based on ensemble local mean decomposition (ELMD) hybrid feature extraction and wavelet neural network. ELMD is used to solve the problem of modal aliasing in local mean decomposition (LMD), which can improve the efficiency of LMD. Quantitatively extracting the mixed features of each component and introducing a wavelet neural network for fault type recognition. The experimental results demonstrate that the proposed method has a high accuracy in fault recognition and is an effective fault diagnosis method.
MoreTranslated text
Key words
fault diagnosis method,hybrid feature extraction,wavelet
AI Read Science
Must-Reading Tree
Example
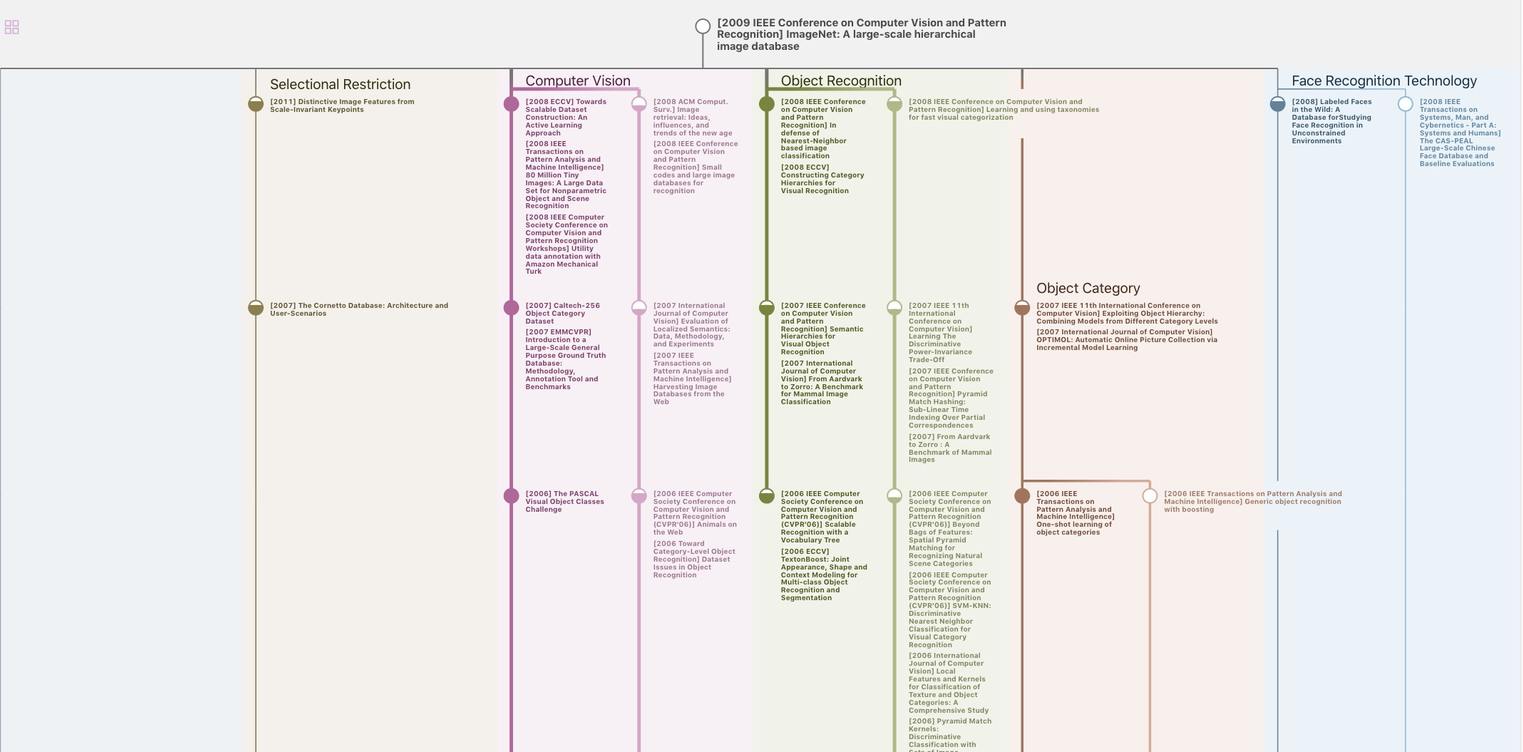
Generate MRT to find the research sequence of this paper
Chat Paper
Summary is being generated by the instructions you defined