Integrating Non-Intrusive Load Monitoring Based on Graph-to-point Learning into a Self-Adaptive Home Energy Management System
INTERNATIONAL JOURNAL OF ELECTRICAL POWER & ENERGY SYSTEMS(2023)
Key words
Non-intrusive load monitoring,Graph-to-point learning,Graph convolutional network,Home energy management system,Discrete Bayesian network,Energy consumption behavior
AI Read Science
Must-Reading Tree
Example
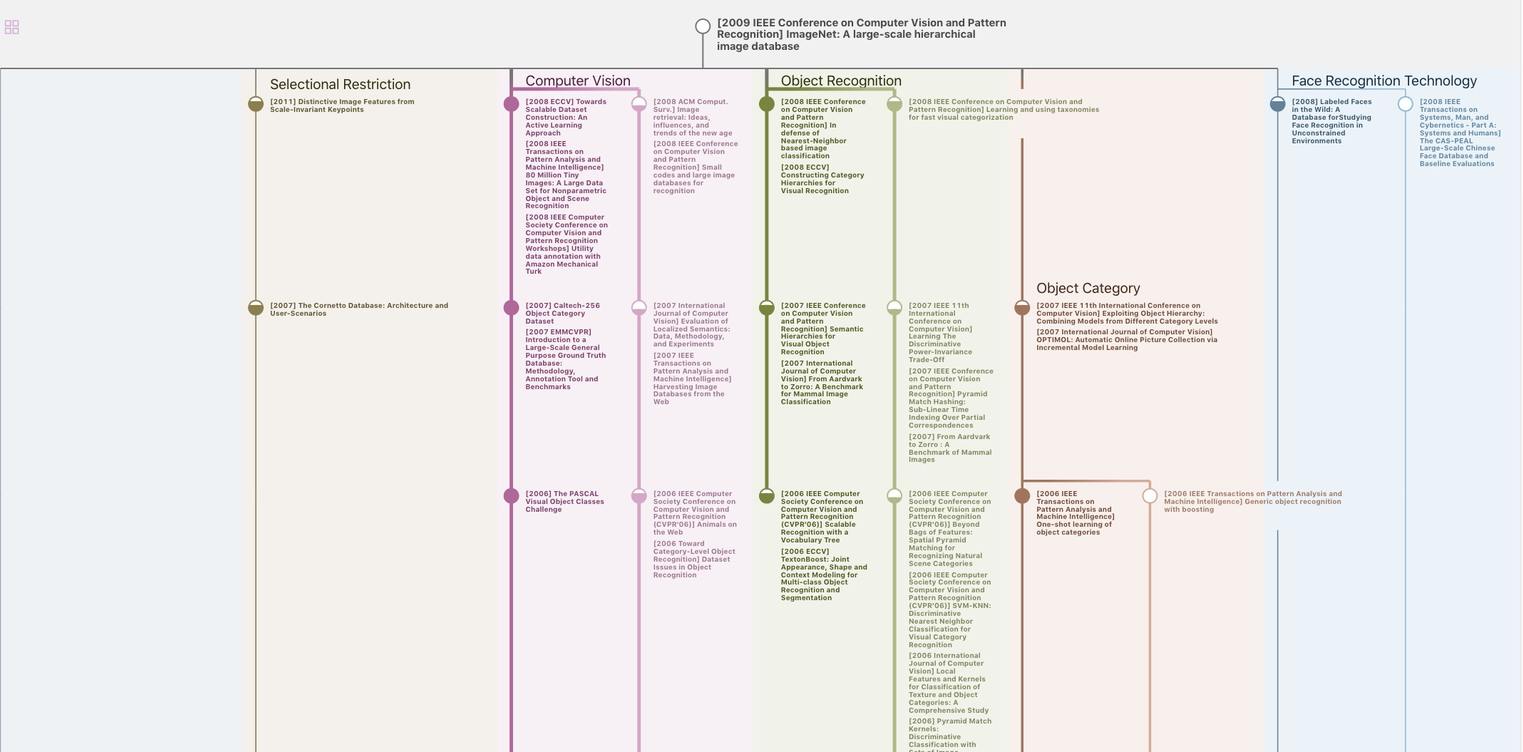
Generate MRT to find the research sequence of this paper
Chat Paper
Summary is being generated by the instructions you defined