Dynamic personalized human body energy expenditure: Prediction using time series forecasting LSTM models
BIOMEDICAL SIGNAL PROCESSING AND CONTROL(2024)
摘要
Dynamic human energy expenditure (EE) consists of energy costs for resting metabolism, food digestion, physical activity, and thermoregulation. Currently, multiple models predict EE mainly concerning physical activity, thus, discarding other factors contributing to the dynamic variation of EE. This paper aimed to demonstrate that (i) a dynamic human body EE prediction requires the time series approach, (ii) personalization of input features and models can outperform the generalized approach. To achieve these objectives, data were collected from 3 sets of experiments with 6 test subjects wearing multiple sensors. The analysis of features' importance showed that the selection of features varies for activity-dominated and non-activity-dominated cases and also varies between individuals. Long-Short Term Memory (LSTM) networks were used to develop personalized models such as a simple LSTM, a convolutional LSTM (CNN-LSTM), and also an ensemble model combining CNN-LSTM with a Gradient Boosting algorithm (LSTM-LGBM). A personalized autoregressive linear model and a generalized approach of the LSTM-LGBM method were also developed to have a base of comparison. The results show that the personalized models provide good prediction accuracy, with the mean absolute percentage error (MAPE) mostly lying in the range of 5-15 %. The CNN-LSTM outperforms a simple LSTM model by 3-5 % in MAPE values, and the ensemble model outperforms the by 5-8 % the simple LSTM. The personalized modeling approach with LSTM has shown the potential to improve the prediction accuracy of dynamic EE and capture the non-activity-related effects such as thermoregulation and postprandial thermogenesis.
更多查看译文
关键词
Human energy expenditure,Dynamic metabolism,Personalized model,Wearable sensing,Machine learning,Time series,Recurrent neural networks
AI 理解论文
溯源树
样例
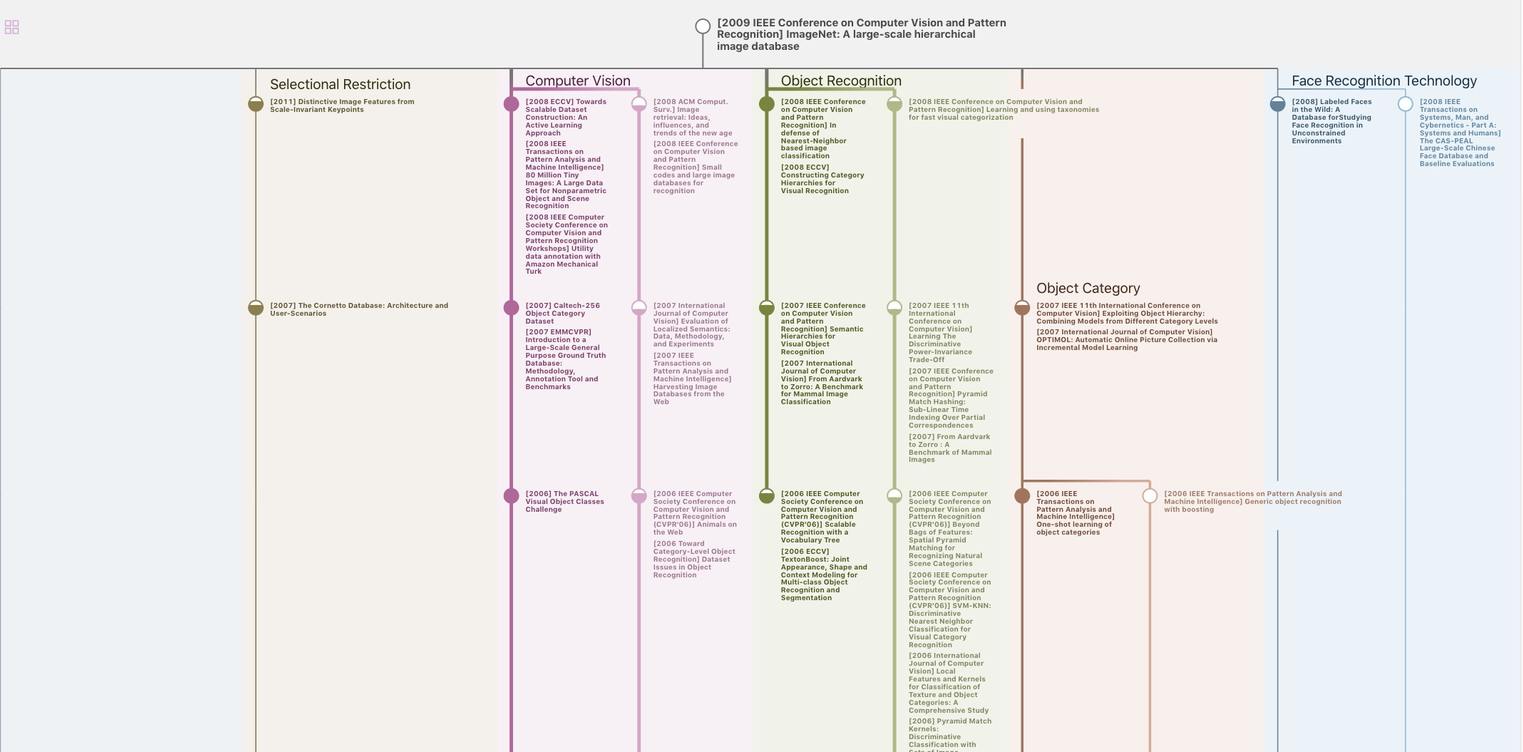
生成溯源树,研究论文发展脉络
Chat Paper
正在生成论文摘要