Securing Recommender System via Cooperative Training
Research Square (Research Square)(2023)
摘要
Abstract Recommender systems are often susceptible to well-crafted fake profiles, leading to biased recommendations. Among existing defense methods, data-processing based methods inevitably exclude normal samples, while model-based methods struggle to enjoy both generalization and robustness. To this end, we suggest integrating data processing and the robust model to propose a general framework, Triple Cooperative Defense (TCD), which employs three cooperative models that mutually enhance data and thereby improve recommendation robustness. Furthermore, Considering that existing attacks struggle to balance bi-level optimization and efficiency, we revisit poisoning attacks in recommender systems and introduce an efficient attack strategy, Co-training Attack (Co-Attack), which cooperatively optimizes the attack optimization and model training, considering the bi-level setting while maintaining attack efficiency. Moreover, we reveal a potential reason for the insufficient threat of existing attacks is their default assumption of optimizing attacks in undefended scenarios. This overly optimistic setting limits the potential of attacks. Consequently, we put forth a Game-based Co-training Attack (GCoAttack), which frames the proposed CoAttack and TCD as a game-theoretic process, thoroughly exploring CoAttack’s attack potential in the cooperative training of attack and defense. Extensive experiments on three real datasets demonstrate TCD’s superiority in enhancing model robustness. Additionally, we verify that the two proposed attack strategies significantly out perform existing attacks, with game-based GCoAttack posing a greater poisoning threat than CoAttack.
更多查看译文
关键词
recommender system,cooperative,training
AI 理解论文
溯源树
样例
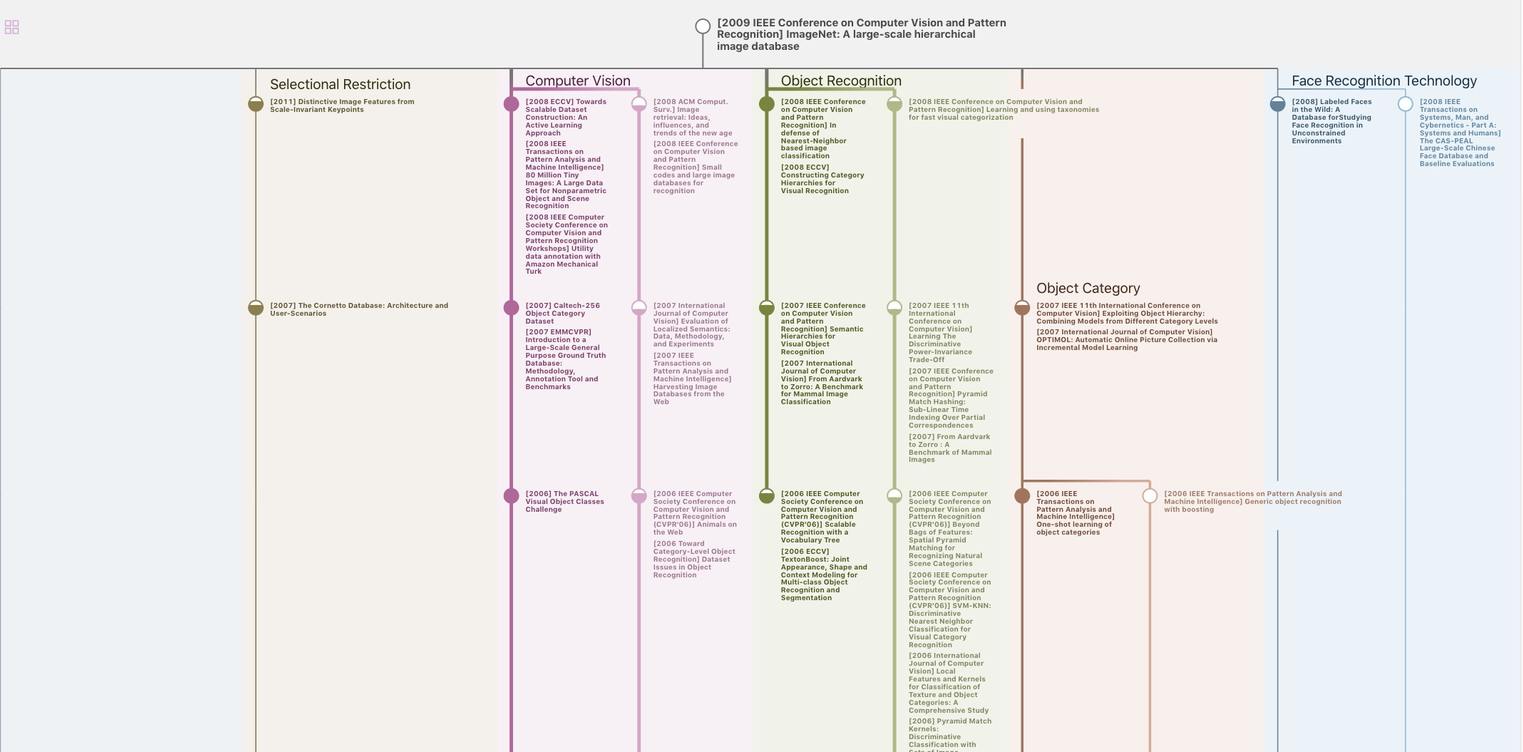
生成溯源树,研究论文发展脉络
Chat Paper
正在生成论文摘要