A recurrent gated unit-based mixture kriging machine Bayesian filtering approach for long-term prediction of dynamic intermittency
IISE TRANSACTIONS(2023)
Abstract
The performance of long-term prediction models is currently impeded due to the mismatch between the nonstationary representations of statistical learning models and the underlying dynamics from real-world systems, which results in low long-term prediction accuracies for many real-world applications. We present a Recurrent Gated Unit-based Mixture Kriging Machine Bayesian Filtering (ReGU-MKMBF) approach for characterizing nonstationary and nonlinear behaviors of one ubiquitous real-world process-dynamic intermittency. It models the transient dynamics in the state space as recurrent transitions between localized stationary segments/attractors. Then, a case study on predicting the onset of pathological symptoms associated with Electrocardiogram signals is presented. The results suggest that ReGU-MKMBF improves the forecasting performance by extending the prediction time horizon with an order of magnitudes while maintaining high accuracies on the foreseen estimates. Implementing the presented approach can subsequently change the current scheme of online monitoring and aftermath mitigation into a prediction and timely prevention for telecardiology.
MoreTranslated text
Key words
prediction,unit-based,long-term
AI Read Science
Must-Reading Tree
Example
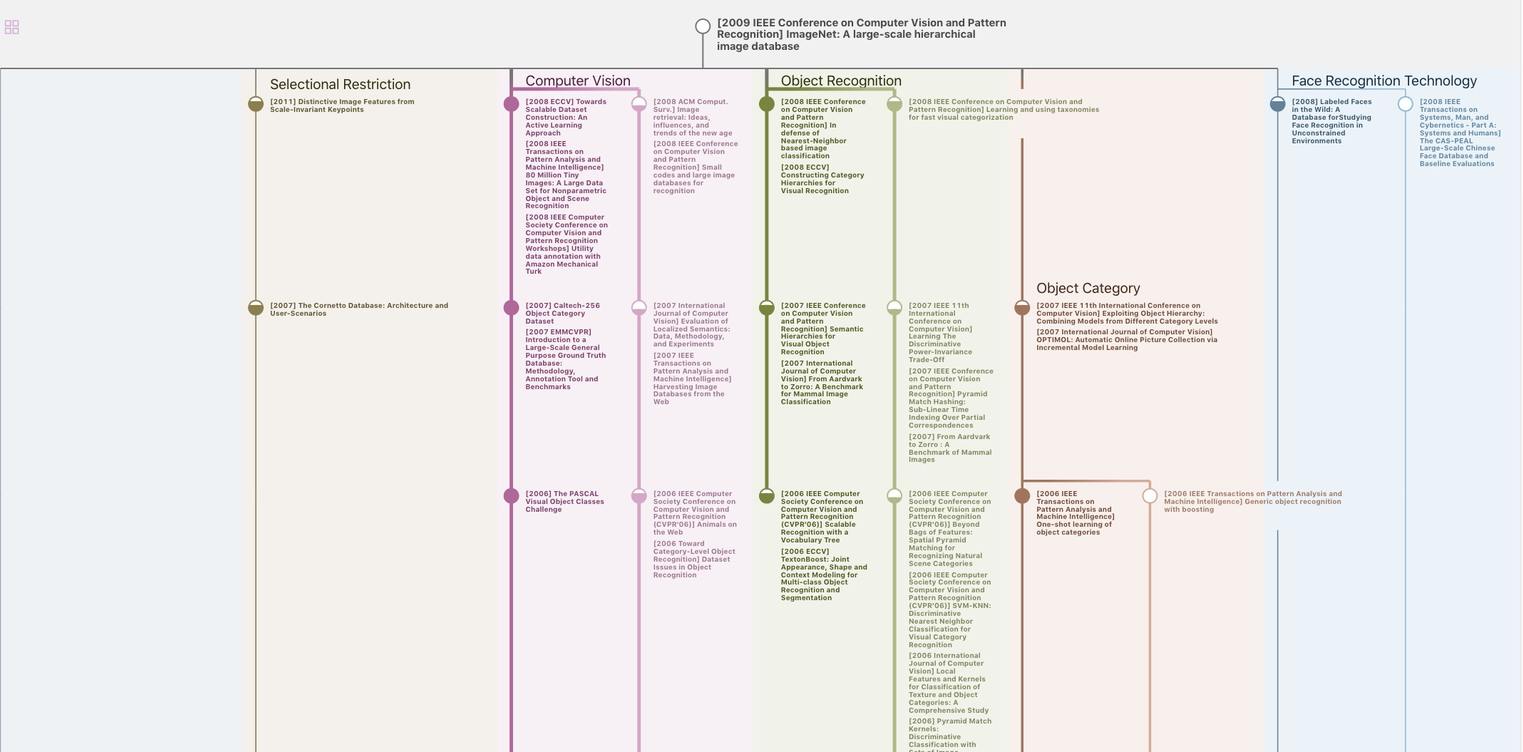
Generate MRT to find the research sequence of this paper
Chat Paper
Summary is being generated by the instructions you defined