Distributed Hybrid DL Cyber-Attacks Detection using Data Parallelism in Cloud-Dew Computing
2023 IEEE TRANSPORTATION ELECTRIFICATION CONFERENCE & EXPO, ITEC(2023)
摘要
Mobility is a synonym for ubiquitous devices on the ground and is more related to secure and distributed connectivity. Research in Machine Learning (ML) has developed techniques and systems that parallelize Deep Learning (DL) training over numerous devices or compute nodes as the pace of innovation in these domains has quickened recently. Many systems have failed to deliver overall performance on a variety of DL models as the models' structural complexity increases. Particularly, the knowledge and time needed to map from a suitable distribution strategy to the model are frequently underestimated when it comes to ML scale-up. More specifically, when Autonomous and Connected Vehicles (CAVs) are used as dew devices in cloud-dew computing systems and are planning a safe route in specific road circumstances. Applying parallel training systems to complicated models leads to lower-than-expected performance and nontrivial development overheads in addition to model prototyping. This article identifies and addresses research difficulties in both usability and performance in parallel ML approaches and system implementations, when a CAV generates the dataset for path planning and communicates it on the cloud-dew network, we use a hybrid DL model that uses an LSTM-AE built-in a cyber-attacks detection system. We emulate the situation of numerous CAVs using the same data profile for motion planning on the same road environment. We examine the configuration in a distributed architecture employing synchronous data parallelism, which enables an optimized speedup and improved training runtime by comparing various processing units.
更多查看译文
关键词
Mobility,CAV,data parallelism,distributed architecture,cloud-dew computing,learning
AI 理解论文
溯源树
样例
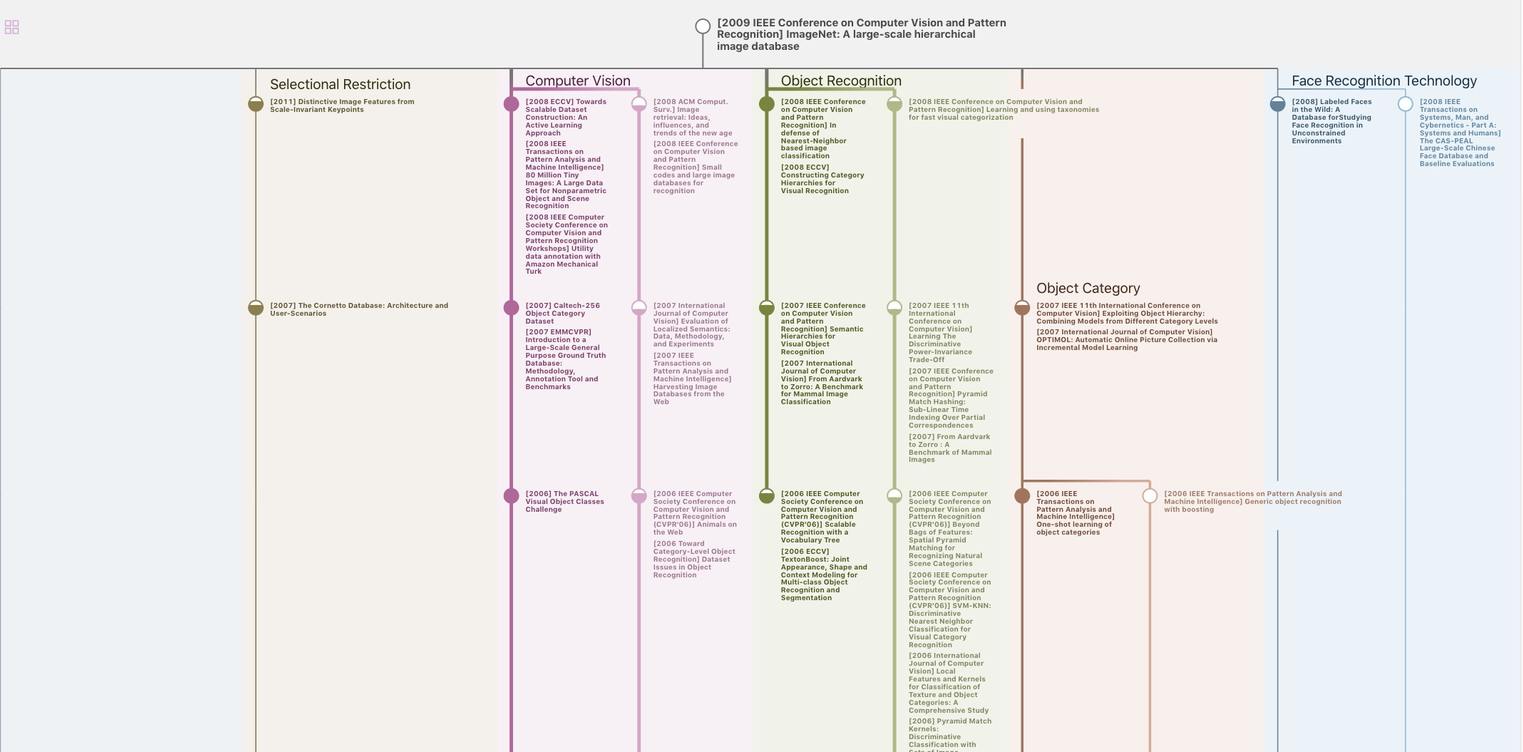
生成溯源树,研究论文发展脉络
Chat Paper
正在生成论文摘要