Electric Vehicle's Range and State of Charge Estimations using AutoML
2023 IEEE TRANSPORTATION ELECTRIFICATION CONFERENCE & EXPO, ITEC(2023)
摘要
This paper examines the potential of AutoML for predicting the range and State of Charge (SOC) of Electric Vehicles (EVs). Unlike traditional SOC estimation methods, such as Coulomb counting, Equivalent Circuit Models (ECM), or Machine Learning (ML)-based approaches, Range Estimation Algorithms (REA) consider route-specific factors to offer more precise battery depletion predictions. However, ML-based REAs can be complex and time-consuming to train, necessitating a deep understanding of Artificial Neural Networks (NN) architecture and optimization strategies. AutoML addresses this issue by automating the selection of the optimal NN architecture, hyperparameters, and data preprocessing techniques, making it more accessible for those with limited expertise to develop effective ML models. Our study centers on constructing SOC estimation and range estimation models using the AutoML library AutoGluon, developed by Amazon Web Services (AWS). Our findings indicate that while SOC estimation alone has limitations in predicting an EV's remaining range, REAs are specifically designed to overcome this challenge by building on SOC estimation to accurately forecast the remaining distance.
更多查看译文
关键词
AutoML, Electric Vehicle, Li-ion, Machine Learning, Range Estimation, SOC
AI 理解论文
溯源树
样例
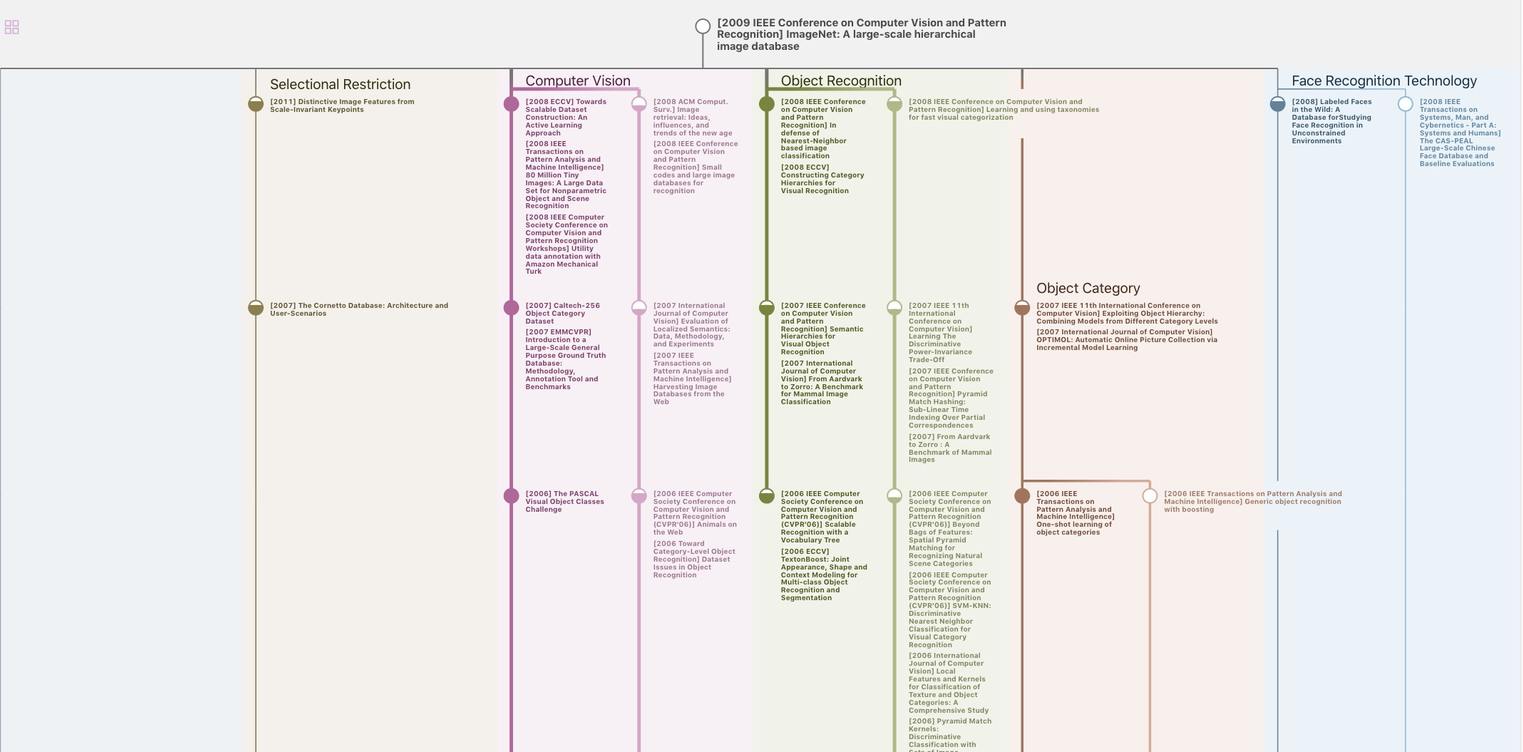
生成溯源树,研究论文发展脉络
Chat Paper
正在生成论文摘要